- Costs Based on the Types of AI Agents
- Reactive (Simple Reflex) Agents
- Model-Based Reflex Agents
- Goal-Based Agents
- Utility-Based Agents
- Learning Agents
- Collaborative Agents
- Factors Impacting the Cost to Develop an AI Agent
- Data Processing and Storage Requirements
- Deployment Costs
- Ongoing Maintenance and Upgrades
- Regulatory Compliance and Ethical Considerations
- User Experience Design
- Security Measures
- Team Expertise and Location
- Time-to-Market Requirements
- Vendor and Licensing Fees
- The Extensive Process to Create an AI Agent Software
- Identify Use Cases and Define Objectives
- Design and Prototype
- Data Collection and Preprocessing
- AI Model Development and Training
- Integration with Existing Systems
- Deployment
- Maintenance
- How to Reduce the AI Agent Development Cost?
- Use Pre-Trained AI Agents Instead of Building from Scratch
- Choose the Right Model Complexity
- Optimize Data Collection and Labeling Costs
- Reduce API Call Costs for AI Agents
- Use Edge AI for Cost-Efficient Processing
- Implement Transfer Learning to Reduce Training Costs
- Optimize AI Model Deployment with Efficient Infrastructure
- Focus on Progressive Development Instead of a Full-Scale Launch
- Use Reinforcement Learning with Simulations to Cut Real-World Training Costs
- Automate AI Model Monitoring and Maintenance
- Why Choose Appinventiv for AI Agent Development?
- FAQs
AI agents are no longer a buzzword. It’s a business advantage. Companies use AI agents to automate customer support, streamline operations, and make smarter real-time decisions. The results? Faster response times, lower costs, and improved customer experiences.
But while the benefits are clear, one question often holds businesses back: How much does it cost to develop an AI agent software? Some claim you can do it for a few thousand dollars, while others discuss six-figure investments.
The reality? There’s no one-size-fits-all answer. The cost of developing an AI agent depends on your need – a simple chatbot or a complex, self-learning system that integrates across multiple platforms and beyond development.
There are hidden costs like training, maintenance, and scaling that many businesses overlook. Cost ranges that get justified once you factor in the market you are going to be a part of.
The AI Agent Software Development Cost ranges from $40,000 to $4,00,000. The difference between a smart investment and an expensive experiment is understanding these costs upfront. This article breaks down the key factors affecting AI agent development cost, helping you decide where to spend, save, and make AI work for your business.
Costs Based on the Types of AI Agents
AI agents tend to vary in complexity, functionality, and purpose. Some operate on predefined rules, while others adapt and learn over time. Like the types of AI agents, the cost of developing these agents also depends on their capabilities, integrations, and level of intelligence.
Here’s a breakdown of different types and their artificial intelligence agent software development cost at basic, mid-level, and advanced stages.
Reactive (Simple Reflex) Agents
These agents operate purely on predefined rules, responding to specific inputs without learning from past experiences. They’re best suited for tasks where the environment is fully observable. Here’s how much reactive AI agent development costs.
Basic Development: Handles simple if-then logic
- Estimated Cost: $5,000 – $15,000
- Example: Motion-sensing home automation systems.
Mid-Level Development: multi-condition responses with basic integrations
- Estimated Cost: $15,000 – $25,000
- Example: Automated quality control systems in manufacturing.
Advanced Development: Extensive rules and real-time data processing
- Estimated Cost: $25,000 – $40,000
- Example: Fraud detection using a machine learning system for financial transactions.
Model-Based Reflex Agents
Unlike reactive agents, these maintain an internal model of the environment, allowing them to make decisions based on historical data. Here’s how much model-based reflex custom AI agent software development cost.
Basic Development: Uses a limited internal model for decision-making
- Estimated Cost: $20,000 – $35,000
- Example: Chatbots that provide responses based on recent interactions.
Mid-Level Development: Predictive capabilities and deeper contextual awareness
- Estimated Cost: $35,000 – $50,000
- Example: AI personal assistants that manage calendar events based on past user behavior.
Bonus Read: AI Personal Assistant App Development Cost
Advanced Development: Machine learning for real-time adaptability
- Estimated Cost: $50,000 – $70,000
- Example: AI-powered virtual doctors that diagnose conditions based on patient history.
Goal-Based Agents
These agents evaluate different possible actions to determine the best course toward a specific goal. Here’s the approximate cost to build an AI agent software
Basic Development: Simple goal-setting and execution
- Estimated Cost: $30,000 – $50,000
- Example: AI-driven route optimization in logistics.
Mid-Level Development: Adapts dynamically to real-time changes
- Estimated Cost: $50,000 – $70,000
- Example: AI-powered warehouse robots that unlock the AI in inventory management strategies.
Advanced Development: Incorporates multi-layered goal planning
- Estimated Cost: $70,000 – $100,000
- Example: AI project managers that autonomously allocate resources based on deadlines and workload.
Utility-Based Agents
These agents make decisions based on utility functions, optimizing for maximum efficiency, profitability, or user satisfaction. The cost of AI agent development here can vary from –
Basic Development: Simple utility functions for decision-making
- Estimated Cost: $40,000 – $60,000
- Example: Building recommendation system engines.
Mid-Level Development: Multi-variable utility assessments
- Estimated Cost: $60,000 – $80,000
- Example: Personalized AI-driven marketing automation tools.
Advanced Development: Predictive analytics with complex real-time data processing
- Estimated Cost: $80,000 – $120,000
- Example: AI-driven financial advisors that adjust investment strategies based on market trends.
Learning Agents
These AI systems evolve, using experience to improve their performance. The cost of artificial intelligence agent here can range from –
Basic Development: Uses supervised learning for pattern recognition
- Estimated Cost: $50,000 – $70,000
- Example: AI email filters that improve spam detection over time.
Mid-Level Development: Implements reinforcement learning to improve decision-making
- Estimated Cost: $70,000 – $100,000
- Example: AI in stock trading that learns from past market fluctuations.
Advanced Development: Deep learning with autonomous adaptation and problem-solving.
- Estimated Cost: $100,000 – $150,000
- Example: AI self-driving car systems that continuously improve driving behavior.
Collaborative Agents
These systems are designed to work alongside humans or other AI agents and facilitate collaborative problem-solving. Here’s the custom AI agent software development cost for the collaborative category.
Basic Development: Basic task-sharing AI for human-AI collaboration
- Estimated Cost: $60,000 – $80,000
- Example: AI-powered document processing and collaboration assistants.
Mid-Level Development: Synchronizes with multiple systems for enhanced coordination.
- Estimated Cost: $80,000 – $120,000
- Example: AI-powered virtual team members that contribute to business meetings.
Advanced Development: Fully autonomous AI agents collaborating with humans
- Estimated Cost: $120,000 – $200,000
- Example: AI co-pilots that assist surgeons in real-time operations.
By understanding the different types and the cost to build AI agent software at various levels of complexity, businesses can make informed decisions about their AI investments. Whether looking for a simple automation tool or a highly advanced AI system, aligning costs with business objectives is key to maximizing ROI.
Factors Impacting the Cost to Develop an AI Agent
Developing an AI agent involves various factors that significantly influence the overall AI agent software development cost. Beyond the commonly cited aspects like complexity, customization, data requirements, system integration, model training, and maintenance, several additional elements can impact the financial investment required. Here’s an expanded overview of all those factors:
Development Approach
The strategy chosen to create an AI agent software can substantially affect costs:
- Building from Scratch: Crafting a custom AI model tailored to specific needs offers full control but demands extensive resources, leading to higher expenses.
- Utilizing Open-Source Models: Leveraging pre-trained models like GPT-4 or BERT can reduce development time and costs, though it may limit customization.
- AI-as-a-Service Platforms: Employing services from providers like AWS, Azure AI, or Google AI offers scalable solutions with subscription-based pricing, often resulting in cost savings.
Bottom Line: The choice between the custom process to make an AI agent software and leveraging existing platforms should align with the project’s specific requirements and budget constraints.
Data Processing and Storage Requirements
The volume and nature of data required for training and operating an AI agent influence storage and processing expenses:
- Small Datasets: Manageable data volumes may incur lower storage and processing costs.
- Large Datasets: Extensive data necessitates robust infrastructure, increasing expenses.
- Real-Time Data Processing: Systems requiring instantaneous data handling demand advanced computing capabilities, elevating costs.
- Storage Solutions: Choosing between on-premises storage and cloud-based options (e.g., AWS, Google Cloud) impacts cost and scalability.
Bottom Line: Aligning data strategies with business objectives is crucial for low artificial intelligence agent software development cost.
Deployment Costs
The environment in which the technology operates affects both the initial and ongoing cost to build artificial intelligence agent software:
- On-Premises Deployment: Requires significant investment in hardware and maintenance, offering greater control but at higher costs.
- Cloud Deployment: Provides scalability and flexibility with a pay-as-you-go model, often reducing upfront expenses.
- Hybrid Deployment: Combines both approaches, balancing control and scalability, but may introduce complexity and additional costs.
Key Insight: Selecting the appropriate deployment strategy is vital for balancing control, performance, and cost to build AI agent software.
Ongoing Maintenance and Upgrades
Continuous support is essential to ensure the AI agent’s effectiveness over time, along with balancing the cost to develop an AI agent:
- Bug Fixes and Patches: Regular updates are necessary to address issues and maintain security.
- AI Model Re-Training: Periodic updates to improve accuracy and adapt to new data trends are crucial.
- Scalability and Feature Enhancements: As user demand grows, expanding capabilities may require additional investment.
Bonus Read: How Much Does it Cost to Maintain a Software
Key Insight: Planning for ongoing maintenance is crucial to sustaining the AI agent’s performance and relevance.
Regulatory Compliance and Ethical Considerations
Adhering to legal standards and ethical guidelines can influence AI agent development cost:
- Data Privacy Regulations: Ensuring compliance with GDPR-compliant software or laws like CCPA may require additional resources.
- Ethical AI Practices: Implementing measures to prevent biases and ensure fairness can add to development efforts.
Critical Consideration: Proactively addressing regulatory and ethical aspects can mitigate risks and avoid potential fines.
User Experience Design
Creating an intuitive and engaging interface enhances user satisfaction but may increase development complexity and, in turn, the cost of AI agent development:
- User-Centric Design: Investing in UX design ensures the AI agent is accessible and meets user needs.
- Accessibility Features: Incorporating features for users with disabilities can broaden the user base but may require additional resources.
Critical consideration: Prioritizing UX design can lead to higher adoption rates and better overall performance.
Security Measures
Protecting the AI agent from potential threats is essential:
- Data Encryption: Implementing robust encryption technology benefits businesses by safeguarding sensitive information.
- Threat Detection Systems: Deploying advanced security measures helps in early identification of potential vulnerabilities.
Key Insight: Investing in security measures protects the organization and its users, preventing costly breaches.
Team Expertise and Location
The skill level and geographical location of the development team can influence the cost of artificial intelligence agent:
- Experienced Developers: Hiring seasoned professionals may increase upfront costs but can lead to more efficient development and higher-quality outcomes.
- Geographical Location: Development costs vary by region due to differences in labor rates and operational expenses.
Key Insight: Balancing expertise and location can optimize AI agent software development cost without compromising quality.
Time-to-Market Requirements
Accelerated development timelines can greatly impact the cost to develop AI agent software:
- Expedited Development: Rushed timelines may require additional resources, increasing costs.
- Standard Timelines: Allowing adequate time for development can optimize resource allocation and cost.
Bottom Line: Aligning development timelines with business goals and resource availability is crucial for cost management.
Vendor and Licensing Fees
Utilizing third-party tools or platforms can introduce additional artificial intelligence agent software development cost:
- Licensing Fees: Costs associated with using proprietary software or tools.
- Vendor Contracts: Engaging external vendors for specialized services can add to expenses.
Key Insight: Carefully evaluating vendor offerings and licensing agreements can prevent unforeseen costs.
When you build artificial intelligence agent software, you should know that it isn’t just about coding a smart system – it’s about creating an intricate process influenced by data needs, integration challenges, deployment choices, and long-term upkeep.
Each decision, from choosing a development approach to ensuring security and compliance, carries financial implications. Implications that operate on the golden rule that the more advanced and tailored the product, the higher the investment required to create an AI agent software.
The Extensive Process to Create an AI Agent Software
AI agent development involves meticulously planned steps, each contributing to the project’s overall cost. Below is an overview of these stages, accompanied by estimated cost ranges:
Identify Use Cases and Define Objectives
This initial phase involves understanding the specific problems the AI agent will address and setting clear objectives. Collaboration between stakeholders is required to align the AI solution with business goals.
Estimated Cost: $1,000 – $5,000
Note: The cost of AI agent development varies based on the project’s complexity and the level of stakeholder engagement required.
Design and Prototype
In this stage, AI solutions designers create wireframes and prototypes to visualize the AI agent’s functionality and user interface. This step helps refine ideas and gather early feedback.
Estimated Cost: $5,000 – $15,000
Note: Expenses depend on the design’s complexity and the tools used.
Data Collection and Preprocessing
Gathering relevant data and preparing it for training is crucial. This process includes data cleaning, normalization, and augmentation to ensure quality inputs for the AI model.
Estimated Cost: $10,000 – $50,000
Note: All the factors that affect AI agent development cost tend to escalate with the volume and complexity of data required.
AI Model Development and Training
Developers select appropriate algorithms and train the AI model using prepared data. This phase is resource-intensive, often requiring specialized hardware and expertise.
Estimated Cost: $10,000 – $100,000+
Note: AI agent development cost varies based on model complexity and computational resources needed.
Bonus Read: How to Build an Intelligent AI Model: An Enterprise Guide
Integration with Existing Systems
Automating the AI agent into current business systems ensures smooth operation and user acceptance. This step may involve API development and customization.
Estimated Cost: $10,000 – $30,000
Note: Costs depend on system compatibility and integration complexity.
Deployment
Launching the AI agent into the production environment involves setting up servers, configuring networks, and ensuring scalability.
Estimated Cost: $5,000 – $20,000
Note: The cost to make an AI agent software varies greatly based on deployment infrastructure and scale.
Bonus Read: AI Integration Examples: How Top Enterprises Use AI
Maintenance
The last stage of developing an AI agent software – Ongoing support includes monitoring performance, updating models, and addressing issues to ensure the AI agent’s longevity and effectiveness.
Estimated Annual Cost: $10,000 – $50,000+
Note: Costs of developing an AI agent depend on the frequency of updates and the need for continuous improvement.
Understanding these AI agent development stages and associated costs helps budget and plan development, ensuring alignment with business objectives and resource availability.
How to Reduce the AI Agent Development Cost?
Reducing the cost to develop AI agent isn’t just about cutting expenses; it’s about making smart, strategic choices that balance performance and affordability. Businesses can minimize the AI agent development cost without compromising functionality by leveraging pre-trained models to optimize deployment and maintenance.
Below are key strategies tailored specifically to AI agents, ensuring cost-effective development and long-term sustainability.
Use Pre-Trained AI Agents Instead of Building from Scratch
Instead of training an AI agent from the ground up, businesses can use pre-trained agents from platforms like OpenAI, Google Dialogflow, or AWS Lex. Fine-tuning these models to suit specific needs is significantly cheaper than developing a new AI model.
Choose the Right Model Complexity
Not every use case requires a complex AI agent with deep learning capabilities. Rule-based or retrieval-based agents can be sufficient for many applications and cost far less than generative AI models requiring extensive computational power.
Optimize Data Collection and Labeling Costs
Training an AI agent requires large volumes of labeled data, which can be expensive to source. Businesses can reduce costs by:
- Using synthetic data generation
- Leveraging existing datasets from open-source repositories
- Implementing semi-supervised or self-supervised learning techniques to mitigate manual labeling efforts
Reduce API Call Costs for AI Agents
AI models hosted on cloud platforms like OpenAI, Google Cloud AI, and Microsoft Azure charge per API call. Optimizing query structures, batching API calls, and using lower-tier models when possible can drastically reduce operational-level AI agent development costs.
Use Edge AI for Cost-Efficient Processing
Cloud-based AI agents incur ongoing costs for every interaction. Deploying lightweight AI agents on edge devices (local servers, IoT devices, or on-premise solutions) can minimize cloud costs and improve response time.
Bonus Read: On-Premise vs. Cloud: A Detailed Analysis
Implement Transfer Learning to Reduce Training Costs
Instead of training an AI agent from scratch, transfer learning allows businesses to build on existing AI models. This reduces computational costs and the need for high-end GPUs, significantly lowering development expenses.
Optimize AI Model Deployment with Efficient Infrastructure
Hosting an AI agent on high-end GPUs 24/7 can be costly. Instead, businesses can:
- Use model quantization to reduce computational needs
- Deploy AI models on cheaper cloud instances during off-peak hours
- Implement auto-scaling to ensure resources are used efficiently
Focus on Progressive Development Instead of a Full-Scale Launch
Many AI projects fail due to over-investment in features that users may not need. A phased approach reduces unnecessary development costs, starting with a Minimum Viable AI Agent (MVAA) and gradually adding capabilities based on user feedback.
Use Reinforcement Learning with Simulations to Cut Real-World Training Costs
Training in real-world scenarios can be expensive for AI agents that need decision-making capabilities. Instead, using simulated environments (such as OpenAI Gym or Unity ML-Agents) can significantly lower training costs while improving model accuracy.
Automate AI Model Monitoring and Maintenance
AI agents degrade over time due to data drift. Automating model retraining and performance monitoring using tools like MLflow, Weights & Biases, or Vertex AI reduces manual intervention costs while keeping the AI agent accurate.
Let’s create a roadmap that fits your budget
The efforts to build AI agent software don’t have to drain resources. Still, cost-efficiency requires strategic decisions at every stage, which we offer by suggesting businesses leverage pre-trained AI models instead of building from scratch, choose the right level of model complexity, and optimize data collection with synthetic data or self-supervised learning.
Why Choose Appinventiv for AI Agent Development?
Building AI agents that deliver tangible business value requires combining technical expertise, strategic cost management, and a forward-thinking approach.
At Appinventiv, we specialize in developing tailored AI services and solutions to diverse business needs, whether virtual assistants, automation bots, or advanced decision-making systems. Our development process ensures that every AI solution aligns with industry-specific challenges while optimizing development and operational costs.
Cost efficiency is at the core of our approach. By leveraging pre-trained models, optimizing cloud infrastructure, and implementing efficient AI training methods, we help businesses minimize expenses without compromising performance.
But AI development isn’t just about cost – scalability is crucial in ensuring long-term value. Our bespoke AI solutions are designed to evolve with business needs, enabling seamless upgrades and integrations without requiring extensive redevelopment.
Data strategy and security are equally critical. We assist businesses in optimizing data collection, preprocessing, and compliance, ensuring AI agents operate with high-quality data while adhering to industry regulations in the healthcare, finance, and retail sectors.
Our AI software development company follows a series of development strategies to maximize further ROI, including model quantization, efficient inference techniques, and edge AI deployment, reducing cloud costs while maintaining high-performance AI operations.
With a track record of successful AI implementations across industries, we empower startups and enterprises to harness AI without excessive financial risk. At Appinventiv, we develop AI agents and create intelligent, scalable, and cost-effective solutions that drive real business impact. Let’s build your AI-powered future – connect with our experts today.
FAQs
Q. How much time does it take to build an AI agent?
A. The development timeline for an AI agent depends on its complexity, data requirements, and integration needs.
- Basic AI agents (rule-based chatbots, simple automation tools) can be developed for 4-8 weeks.
- Mid-level AI agents (context-aware assistants, predictive models) typically require 3-6 months for training, testing, and deployment.
- Advanced AI agents (generative AI models, real-time decision-making systems) may take 6-12 months or longer, especially if they require custom training datasets and high-level optimizations.
The timeline can vary based on the training data available, the need for model fine-tuning, and the integration with existing business systems.
Q. How much does it cost to build an AI system?
A. The cost of developing an AI system varies widely based on its complexity, data needs, and deployment infrastructure:
- Basic AI agents: $10,000 – $50,000
- Mid-range AI agents: $50,000 – $250,000
- Advanced AI agents: $250,000 – $1M+
AI model training, cloud infrastructure, API costs, and ongoing maintenance can impact the final budget. Businesses can lower costs by leveraging pre-trained models and cloud-based AI services.
Q. How are AI agents built?
A. AI agents are developed through a structured process that includes:
- Defining Objectives – Identifying the use case, required functionalities, and expected outcomes.
- Data Collection & Processing – Gathering and preprocessing relevant datasets for AI model training.
- Model Selection & Training – Choosing the right AI model (machine learning, deep learning, NLP, etc.) and training it using labeled data.
- Integration with Business Systems – Connecting the AI agent to existing software, APIs, or enterprise applications.
- Testing & Refinement – Evaluating performance, addressing biases, and improving response accuracy.
- Deployment & Maintenance – Deploy the AI agent in a live environment and continuously monitor it for updates and improvements.
Each stage influences cost and development time, making strategic planning essential for efficiency.
Q. What are the challenges in developing artificial intelligence agent software?
A. AI agent development comes with several challenges, including:
- High Data Requirements – AI models need large datasets for accurate predictions, and acquiring quality data can be costly and time-consuming.
- Computational Costs – Training AI models, especially deep learning-based ones, requires expensive computing resources like GPUs and cloud services.
- Integration Complexity – AI agents must work seamlessly with existing business systems, which can require significant backend modifications.
- Ethical & Bias Concerns – AI models can inherit biases from training data, leading to inaccurate or unfair decision-making.
- Continuous Maintenance – AI agents need regular updates to stay relevant, prevent model drift, and improve performance over time.
Addressing these challenges early in development helps build a reliable, cost-efficient AI agent.
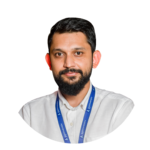
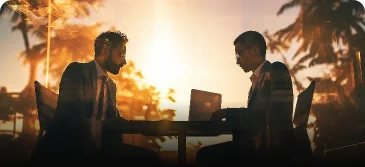
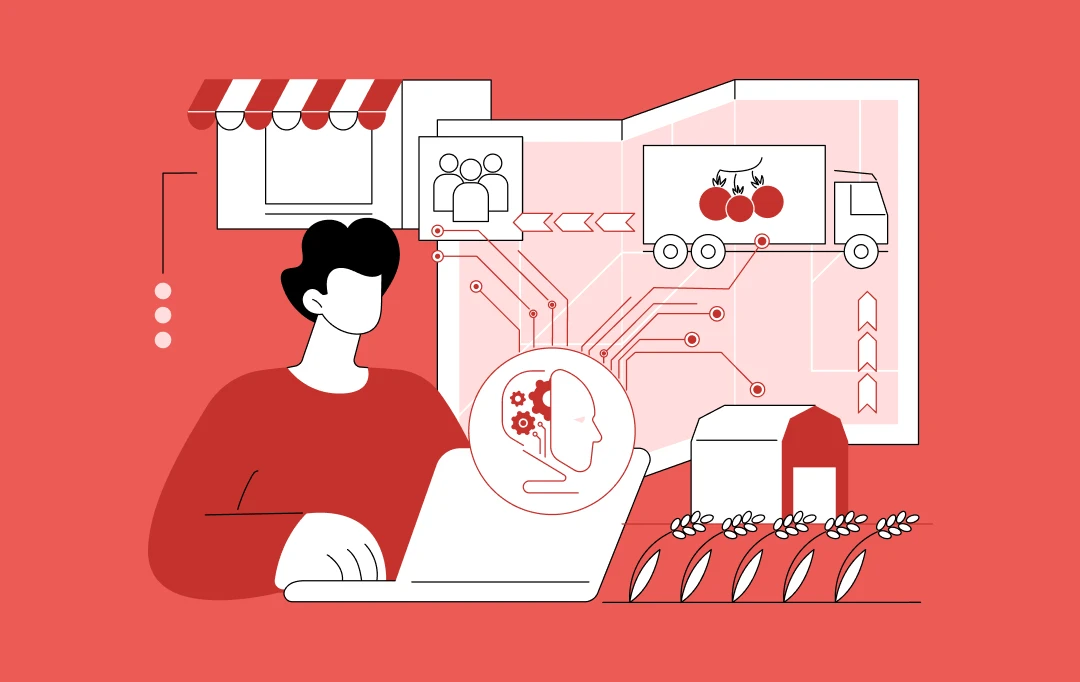
15 Use Cases and Real Examples of How AI is Transforming the FMCG Industry
Artificial Intelligence (AI) is no longer a concept for the future—it's quietly becoming the backbone of some of the most iconic consumer brands. In the fast-paced world of FMCG, where shelf space is fiercely contested and consumer preferences shift overnight, brands use smarter technologies to stay ahead, not in theory, but in practice. The Generative…
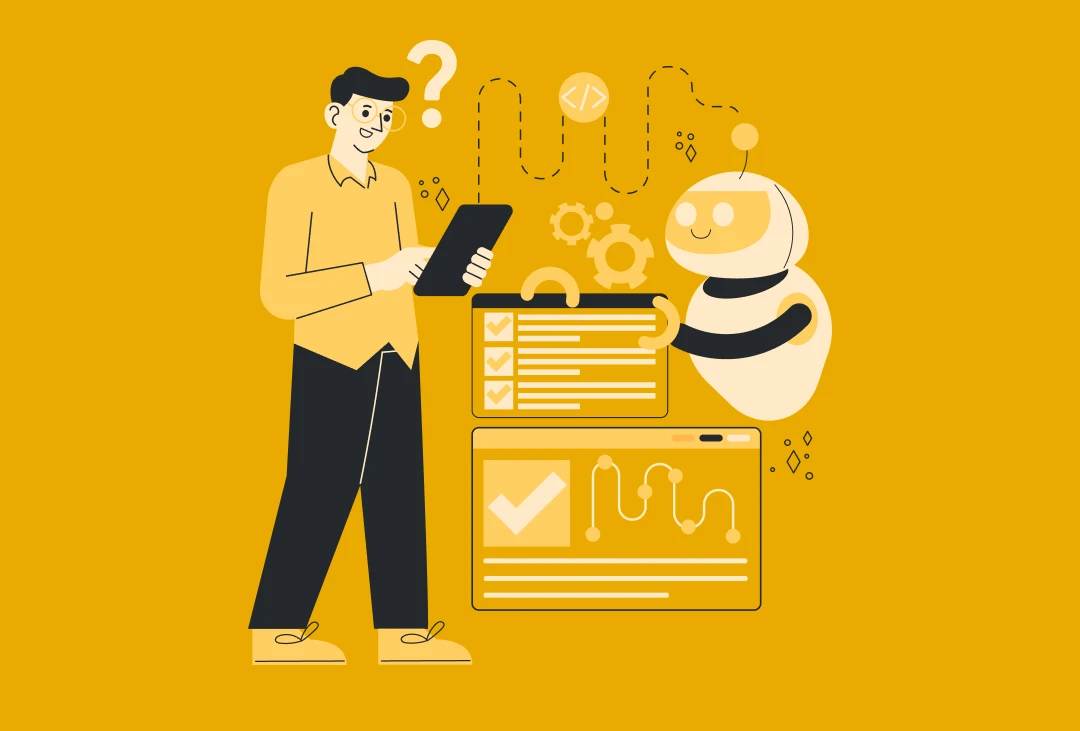
Why and How to Build an AI MVP of Your Product?
Mark had a breakthrough idea - an AI-driven hiring assistant that could predict a candidate’s success in a company based on past hiring data. The concept was solid, investors showed interest, and his team jumped into development. They worked tirelessly for the next 18 months: training complex models, refining algorithms, and building a polished, full-fledged…
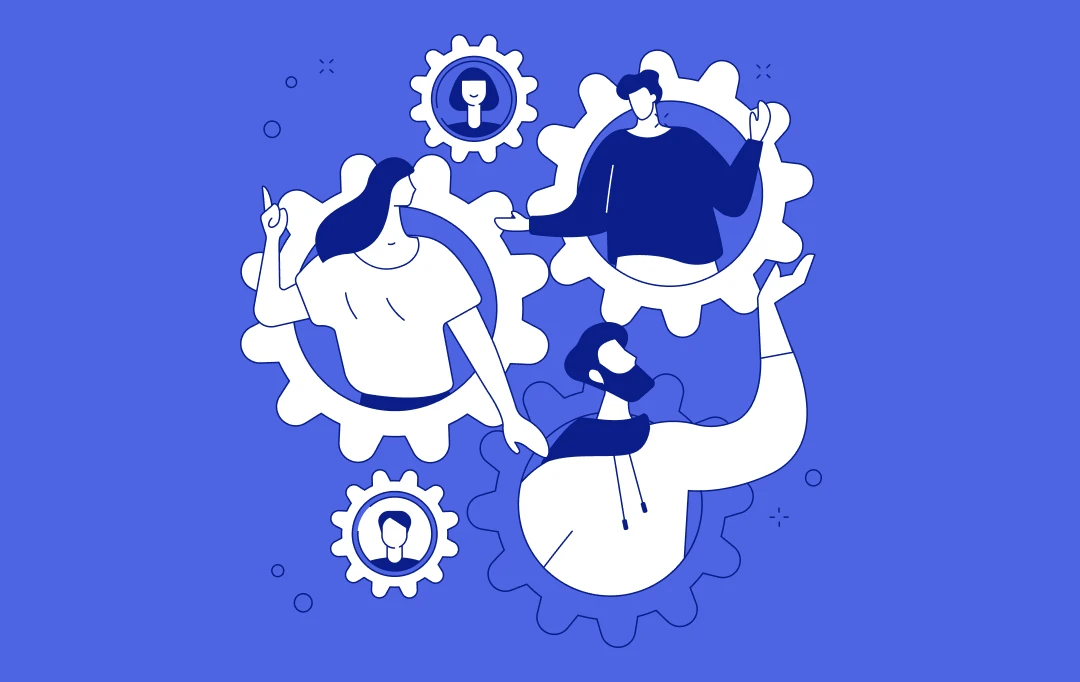
AI Integration Examples: How Leading Enterprises Are Transforming Operations with AI
Nearly 92% of companies invest in AI, but only 1% of leaders and CEOs consider themselves mature in deploying AI in their operations. This is a huge gap—who is responsible? Leaders and CEOs still treat AI as an experimental project. For years, 92% of businesses that are using AI have always associated the adoption of…