- Understanding AI Model Collapse
- How Does AI Model Collapse Happen?
- Loss of Rare Events
- Amplification of Biases
- Narrowing of Generative Capabilities
- Functional Approximation Error
- Implications of Model Collapse: Why AI Model Stability Matters?
- AI Model Collapse Prevention: Understanding the AI Model Collapse Solutions
- Diverse Training Data
- Regularly Refresh Synthetic Data
- Augment Synthetic Data
- Monitoring and Regular Evaluation
- Fine-Tuning
- Bias and Fairness Analysis
- Feedback Loops
- How can Appinventiv Help with Risk Mitigation in AI Models?
- FAQs
Artificial Intelligence (AI) has significantly transformed our everyday lives by suggesting personalized content on streaming platforms and enabling digital assistants on smartphones. Now, these advancements are made possible by sophisticated AI models that learn from vast amounts of data.
As per various reports, AI-generated content is becoming increasingly prevalent on the internet, potentially comprising up to 90% of online information in the coming years.
With such an influx of information, one can easily say that in today’s data-rich world, AI faces a unique challenge, and that is choking on its abundance of data.
The reports further suggest that the significant amount of this AI-generated content can overwhelm people with excessive information, making it difficult for them to determine what is trustworthy and human-generated. In addition, there are concerns about the potential loss of jobs in creative fields such as art, journalism, and writing, all because AI is becoming more capable of producing content traditionally created by humans.
Coming to the AI systems themselves, there are emerging issues like “Model Collapse,” which refers to a problem where AI models that are trained on large datasets produce lower-quality outputs by prioritizing common word choices over creative alternatives. “Model Autophagy Disorder” or “Habsburg AI” is another concern where AI systems excessively trained on the outputs of other AI models can exhibit undesirable features or may have biases.
These challenges can potentially harm the quality and reliability of AI-generated content, destroying the trust in such systems and worsening the information overload.
Our blog will help you understand everything related to addressing AI model collapse prevention. As the generative AI revolution progresses, it brings forth significant challenges and uncertainties for the online information landscape. So, let’s dive into the details head-on.
Understanding AI Model Collapse
In machine learning, “model collapse” refers to a situation where the AI model fails to provide a variety of useful outputs. Instead, it produces a narrow set of repetitive or low-quality results. This issue can occur in various models, but it’s often observed during training complex models like generative adversarial networks (GANs). Model collapse can hamper the model’s ability to generate diverse and valuable outputs, impacting its overall performance.
Let’s illustrate a model collapse example. Imagine a highly enthusiastic art student representing our AI model, which is tasked with creating paintings of zebras. In the beginning, their artwork is impressive and distinctly resembles zebras. However, their paintings gradually lose their resemblance to zebras as they continue, and the quality declines. This is similar to “model collapse” in machine learning, where the AI model, like our art student, initially performs well but then struggles to maintain the essential characteristics it was designed to perform.
As per the recent advancements in AI, researchers have become very interested in using artificial or synthetic data to train new AI models when it comes to generating images and text. However, a concept called ‘Model Autophagy Disorder’ (MAD) compares this process to a self-destructive loop.
Unless we keep adding fresh real-world data regularly, the quality and variety of the AI models we create using synthetic data could worsen over time. So, it’s essential to strike a balance between synthetic and real data to keep AI models performing well.
This balance is crucial to prevent a decline in the quality and diversity of the models as they continue to learn. Finding out how to effectively use synthetic data for AI model collapse prevention is an ongoing challenge when it comes to the evolution of generative AI and the use of synthetic data.
According to The New Yorker, if ChatGPT is considered a compact version of the internet, similar to that of a JPEG file compressing a photograph, then training the future chatbots on the results of ChatGPT’s is digitally equivalent to repeatedly making photocopies of photocopies just like the old days. Simply, the image quality is bound to get worse with each iteration.
Thus, to overcome this challenge, organizations need to focus on refining their approaches to ensure these generative AI products continue to provide accurate responses in this digital landscape.
[Also Read: Responsible AI – Addressing Adoption Challenges With Guiding Principles and Strategies]
How Does AI Model Collapse Happen?
Model collapse occurs when new AI models are trained using data generated by older models. These new models rely on the patterns seen in the generated data. Model collapse is rooted in the idea that generative models tend to repeat patterns they have already learned, and there’s a limit to the information they can extract from these patterns.
In cases of model collapse, events that are likely to happen are exaggerated, while less likely events are underestimated. Over multiple generations, likely events dominate the data, and the less common but still crucial parts of the data, called tails, diminish. These tails are essential to maintaining the accuracy and diversity of the model’s outputs. As generations progress, errors conquer the data, and the model increasingly misinterprets it.
According to the research, there are two types of model collapse: early and late. Early model collapse involves the model losing information about rare events. In late-model collapse, the model blurs distinct patterns in the data, resulting in outputs that have little resemblance to the original data.
Let us look at multiple reasons for AI model collapse in detail below:
Loss of Rare Events
When AI models are repeatedly trained on data generated by their previous versions, they try to focus on common patterns and forget rare events. This phenomenon is similar to the models losing their long-term memory. Rare events often hold significant importance, such as identifying anomalies in manufacturing processes or detecting fraudulent transactions. For example, when it comes to fraud detection, specific language patterns may signal fraudulent behavior, making it crucial to retain and learn these rare patterns.
Amplification of Biases
Each training iteration on AI-generated data can amplify the existing biases in the training data. Since the model’s output usually reflects the data it was trained on, any biases within that data can be exaggerated with time. This can lead to bias amplification in various AI applications. For instance, the results can lead to issues like discrimination, racial bias, and biased social media content. Thus, implementing controls to detect and mitigate bias is extremely essential.
Narrowing of Generative Capabilities
As AI models continue learning from their generated data, their generative capabilities can narrow. The model becomes rather influenced by its own interpretations of reality, producing increasingly similar content that lacks diversity and representation of rare events. This can lead to a loss of originality. For instance, when it comes to Large Language Models (LLMs), the variation imparts each writer or artist with their distinct tone and style.
Research simply suggests that if fresh data is not added regularly during the training process, future AI models could end up becoming less accurate or producing less varied results over time.
Functional Approximation Error
A functional approximation error can occur when the function approximators used in the model are not expressive enough. While this error can be mitigated by employing more expressive models, it can also introduce noise and lead to overfitting. Striking the right balance between the model expressiveness and noise control is crucial to prevent these errors.
Implications of Model Collapse: Why AI Model Stability Matters?
Model collapse can ultimately impact the quality, reliability, and fairness of AI-generated content, which can further pose several risks to organizations. Let us look at the implication of model collapse in detail below:
Quality and Reliability
As AI models degenerate in their learning, the content they generate becomes less reliable, and their quality degrades. This happens when the models detach from the original data distribution and rely more on their own interpretations of reality. For instance, an AI model designed for news generation may produce inaccurate or even completely fabricated news articles.
Fairness and Representation
Model collapse is also a cause for concern when it comes to fairness and representation of the generated content. When models forget rare events and limit their generative abilities, content related to less common topics may be inadequately represented. This leads to biases, stereotypes, and the exclusion of certain perspectives.
Ethical Concerns
Model collapse poses significant ethical concerns, specifically when AI-generated content has the power to influence decision-making. The consequences of model collapse include the spread of biased and inaccurate content, which can significantly impact people’s lives, opinions, and access to opportunities.
Economic and Social Impact
On an economic and social scale, model collapse can influence trust and adoption of AI technologies. If AI-generated content cannot be relied upon, businesses and consumers may hesitate to embrace these technologies. This can have economic implications, and trust in AI technologies can hamper consequently.
AI Hallucination
AI hallucination is when AI models create imaginative or unrealistic content that doesn’t align with facts or is coherent in any way. This can result in inaccurate information, potentially causing misinformation or confusion. It’s significantly problematic in applications like generating news, diagnosing medical conditions, or creating legal documents where accuracy and reliability are extremely vital.
Let us explain the context with an AI hallucination example. Suppose there is an AI model that is trained to generate pictures of animals. Now, upon requesting a picture of an animal, the model might produce an image of a “zebroid,” a hybrid between a zebra and a horse. While this image may feel visually realistic, it is vital to understand that it is only a creation of the AI model’s imagination, as no such animal exists in the real world.
[Also Read: Harnessing the Power of AI for Enhanced Risk Management in Business]
AI Model Collapse Prevention: Understanding the AI Model Collapse Solutions
To ensure the AI model stability and reliability, it is essential to explore strategies and best practices for effectively addressing AI model collapse prevention. Thus, it is recommended to partner with a dedicated AI development firm like Appinventiv that can provide expertise and guidance in implementing these preventive measures while ensuring your AI systems consistently deliver high-quality results.
Diverse Training Data
For effectively addressing AI model collapse and preventing undesired outputs, it is crucial to curate a training dataset that includes a variety of data sources and types. This dataset should consist of both synthetic data generated by the model and real-world data that accurately represent the complexities of the problem. It is important to regularly update this dataset with new and relevant information. The model is exposed to a wide range of patterns by incorporating diverse training data. This helps in preventing data stagnation.
Regularly Refresh Synthetic Data
Model collapse is a risk when AI models rely heavily on their own generated data. For effective risk mitigation in AI, it is important to regularly introduce new, authentic, real-world data into the training pipeline. This practice ensures the model remains adaptive and avoids getting stuck in a repetitive loop. This can help in generating diverse and relevant outputs.
Augment Synthetic Data
Enhancing synthetic data through data augmentation techniques is a proven method to prevent model collapse. These techniques introduce variability into the synthetic data using the natural variations in real-world data. Adding controlled noise to the generated data encourages the model to learn a wider range of patterns, reducing the chances of generating repetitive outputs.
Monitoring and Regular Evaluation
Regularly monitoring and evaluating AI model performance is crucial for early detection of model collapse. Implementing an MLOps framework ensures ongoing monitoring and alignment with the goals of an organization, thereby enabling timely interventions and adjustments.
[Also Read: How to Avoid Compliance Violations While Developing AI Products]
Fine-Tuning
It is important to consider implementing fine-tuning strategies to maintain model stability and prevent collapse. These strategies for preventing AI model failure enable the model to adapt to new data while preserving its previous knowledge.
Bias and Fairness Analysis
Rigorous bias and fairness analysis are crucial in preventing model collapse and ethical issues. It is essential to identify and address biases in the model’s outputs. You can maintain reliable and unbiased model outputs by actively addressing these concerns.
Feedback Loops
Implementing feedback loops that incorporate user feedback is crucial in preventing model collapse. By consistently gathering user insights, informed adjustments can be made to the model’s outputs. This refinement process guarantees that the model remains relevant, reliable, and aligned with user expectations.
How can Appinventiv Help with Risk Mitigation in AI Models?
In the evolving landscape of AI, the challenges posed by model collapse have been a concern for both tech giants and innovators alike. The long-term deterioration of language model datasets and the manipulation of content have left their mark on this digital ecosystem.
As AI advances, it is vital to differentiate between artificially generated data and human-generated content. The line between genuine content and what’s generated by a machine is becoming increasingly blurred.
Now, amidst these challenges and preventing AI model failure, partnering with a dedicated AI development company like Appinventiv can provide you with much-needed solace. With expertise in AI model development and a dedicated commitment to ethical AI practices, we can help you navigate the complexities of AI while ensuring the reliability and integrity of your AI systems.
Our experts can work with you in addressing AI model collapse prevention effectively, promote transparency, and build a future with authentic content that doesn’t compromise the authenticity of human-generated content.
We understand that training AI models with fresh, diverse data is essential to prevent model degradation. AI model evaluation is a pivotal step in our model development process that employs metrics to assess performance, pinpoint weaknesses, and ensure effective future predictions.
Our expert team can help ensure that your AI systems continue to learn and adapt to the evolving digital landscape. Get in touch with our experts to mitigate the risks associated with model collapse and ensure their effectiveness.
FAQs
Q. What is AI model collapse?
A. AI Model collapse in machine learning refers to the AI model’s failure to produce a diverse range of useful outputs. Instead, it generates repetitive or low-quality results. This problem can occur in different types of models, but it is particularly observed during the training of complex models like generative adversarial networks (GANs).
Q. What are the common causes of AI model collapse?
A. Common causes of AI model collapse include loss of rare events, amplification of biases, narrowing of generative capabilities, functional approximation errors, etc. These factors can lead to models producing suboptimal outputs.
Q. How can I prevent AI model collapse?
A. For effective AI model collapse prevention, using different and real-world-like training data is vital, continuously monitoring and evaluating data, fixing any biases, and implementing rigorous testing and quality control. Partnering with the AI experts at Appinventiv can offer you valuable insights and solutions to mitigate model collapse risks.
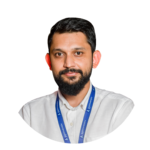
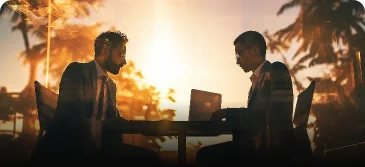
- In just 2 mins you will get a response
- Your idea is 100% protected by our Non Disclosure Agreement.
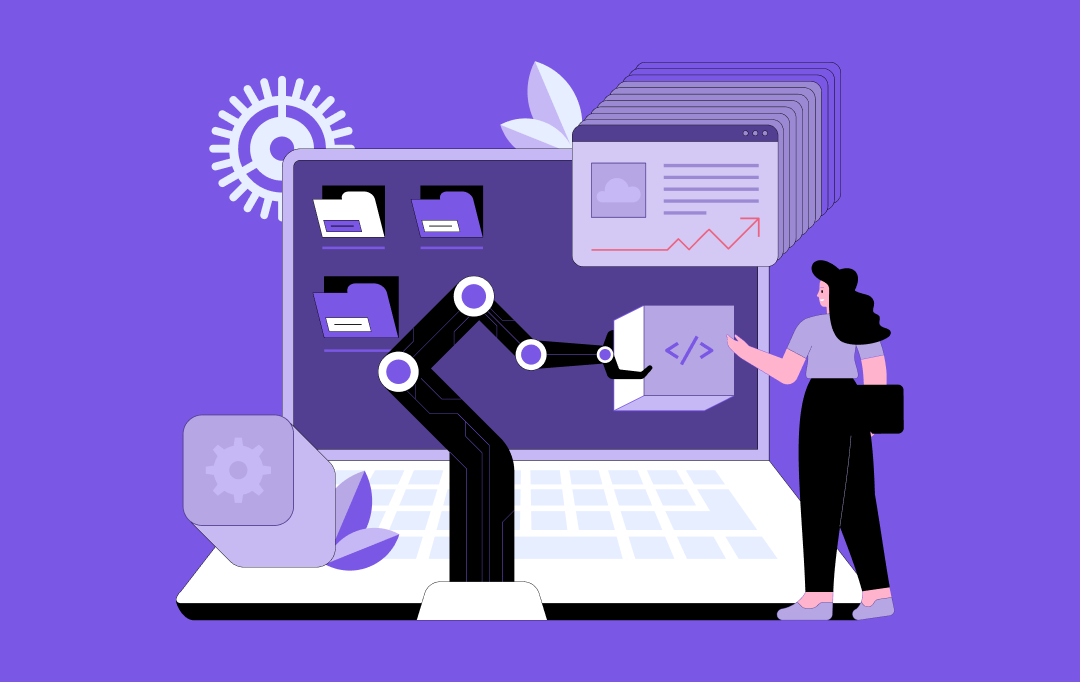
Integrating AI into ERP Systems - Benefits, Process, and Costs
Key takeaways: Market Growth: The global AI in ERP market is projected to reach USD 46.5 billion by 2033, highlighting its rapid growth trajectory. Automation: AI automates tasks like invoice processing and inventory management, cutting costs by up to 25% using RPA and OCR. Use Cases: AI enhances ERP systems with capabilities such as demand forecasting, predictive maintenance,…
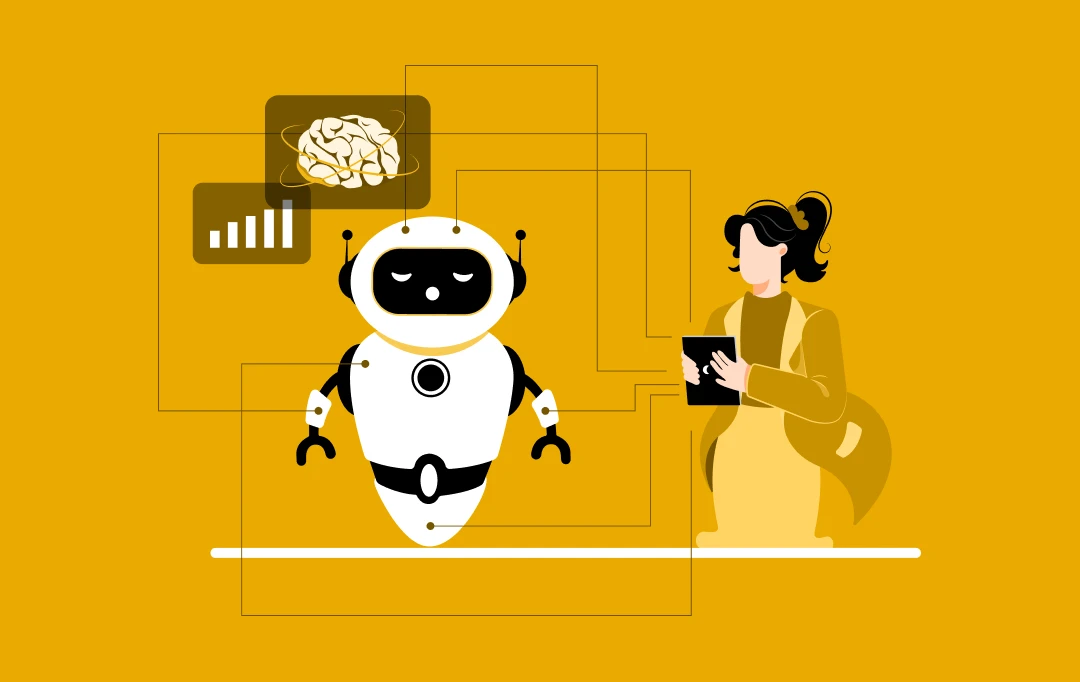
How Agentic AI is Addressing Enterprise Challenges and Driving Business Growth in Australia
Key takeaways: Agentic AI drives business growth by automating tasks, improving efficiency, and making independent decisions. Australian enterprises benefit from AI in overcoming data integration challenges, enhancing productivity, and optimizing cybersecurity. Industries such as Healthcare, Retail, and Finance are experiencing tangible improvements in customer experience and operational efficiency thanks to Agentic AI. Scalability and cost…
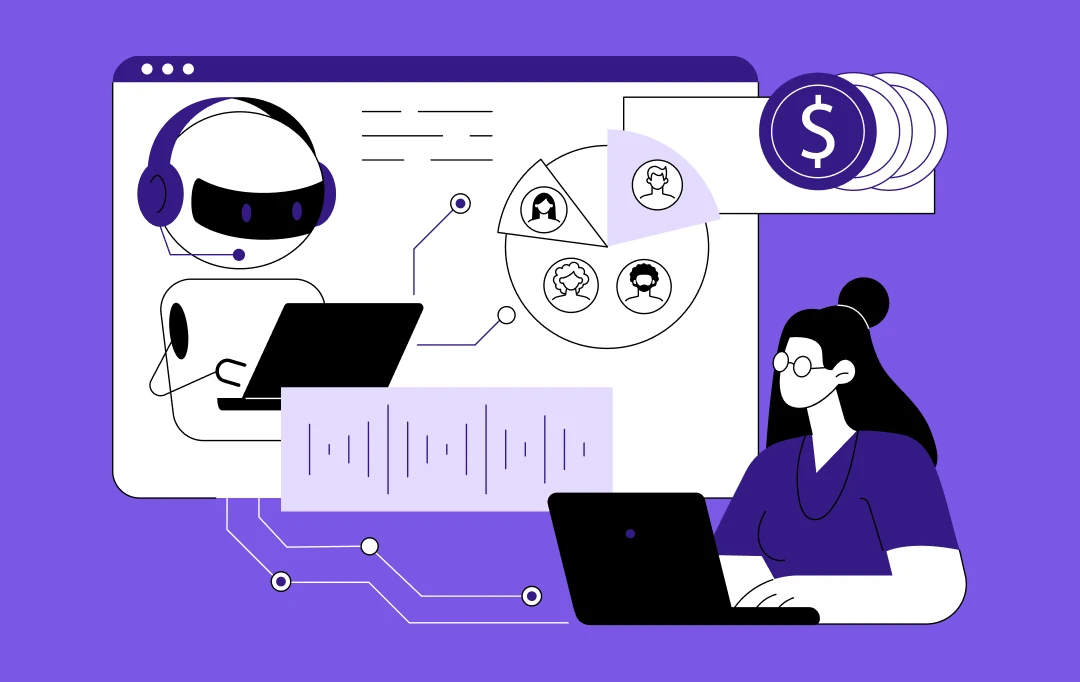
Essential Questions Every Leader Should Ask Before Investing in Custom AI for Business
Key takeaways: Businesses should ask how AI solutions align with their long-term goals and deliver measurable outcomes that address specific business needs, such as improving efficiency or personalizing CX. Before investing in custom AI, businesses must inquire about the costs of developing, implementing, and maintaining custom AI solutions, as well as projected ROI. It's essential…