- Logistics Industry: Before vs. After the Inclusion of Machine Learning
- McKisney Survey Report on How ML in Logistics delivers revenue growth to businesses
- DHL’s Approach to Deploying ML in Logistics & Their Key Achievements
- 10 ML Use Cases in Logistics: With Real Examples
- Supplier Relationship Management
- Dynamic Freight Pricing
- Smart Inventory Management
- Fuel Consumption Modeling
- Package Condition Detection
- Intelligent Slotting in Warehouses
- Driver Behavior Monitoring
- Dynamic Routing
- Supply Chain Visibility
- Return Logistics Optimization
- Major Challenges in Implementing ML in the Logistics Industry: Past the USUAL HYPE
- Poor Data Quality
- Legacy IT Infrastructures
- Future of ML in Logistics
- Why Partner with Appinventiv for Your Logistics Solution Needs
- FAQs
The widespread adoption of ML has resulted in the explosion of data from sensors, customer interactions, and digital platforms across different domains. More and more businesses are leveraging machine learning in logistics to improve their strategic decision-making expertise centered around tailoring customer experience, improving productivity, increasing engagement, and preventing fraud.
According to the McKinsey Global Survey Report, 15% of respondents have successfully scaled automation across multiple parts of the business, and 36% said that ML algorithms had been deployed beyond the pilot stage.
From a “nice-to-have” approach to a “core” business strategy, the role of machine learning in logistics has empowered businesses to innovate, scale, and stay relevant in today’s competitive marketplace.
Logistics Industry: Before vs. After the Inclusion of Machine Learning
Aspects | Before | After |
---|---|---|
Manual Processes | Static systems, manual processes. Dependent on rule-based systems | Proactive & predictive operations. Customer satisfaction through data-driven decision making. |
Labor Intensive | Manual checks and handwritten records lead to human error. | Identifying patterns in product movement & predicting stock levels. Reduced reliance on manual checks |
Limited Visibility | Communication via phone calls, leading to delays and miscommunications | Analysing data from GPS, RFID, and other sensors, leading to real-time updates. Enhanced transparency |
“Without big data analytics, companies are blind and deaf, wandering out onto the web like a deer on a freeway.”
By Geoffrey Moore (Tech Author and Consultant)
One key factor driving ML in logistics is the growing demand for advanced AI technologies like ML. The global market for High-Tech Logistics was valued at US$50.2 billion in 2024 and is projected to reach US$96.1 billion by 2030, growing at a CAGR of 11.5% from 2024 to 2030. (Source: Research and Markets)
This blog will delve into applications and examples of machine learning in logistics, highlighting how this technology enhances current operations and paves the way for new capabilities. Let’s unpack the specific components of ML that are making a difference and discuss real-world applications that showcase how machine learning in the logistics industry streamlines operations while reducing cost.
Machine Learning in the Logistics Industry: What Statistics Reveal?
Machine learning in the Logistics Market statistics reveal many insights that help businesses understand the broad ML use cases in logistics and adopt the right strategies that lead to accurate and effective implementation of ML in logistics.
ML automates complete inventory management, logistics planning, potential fraud detection, and more. It allows for quicker and more data-driven decisions, improving the efficiency of overall logistics operations.
McKisney Survey Report on How ML in Logistics delivers revenue growth to businesses
According to McKinsey, larger companies with revenues over $500 million showed a high level of digital adoption in their survey. Lower-revenue companies tend to be less mature in their adoption, be it resource constraints, managerial complexities, or a more cautious investment approach.
- Shippers and service providers are MORE ambitious in adopting gen AI use cases.
- Nearly 46% of respondents pursued digital use cases for planning demand forecasting/logistics resource requirements.
- 50% of respondents utilized AI and ML for dynamic routing purposes.
- 49% of respondents used it for network optimization.
DHL’s Approach to Deploying ML in Logistics & Their Key Achievements
Conventionally, the DHL workflow was mainly driven by traditional logistics operators, who relied on manual processes and legacy IT systems. But now, the company has incorporated a suite of AI, leveraging ML algorithms, computer vision, and human expertise to improve its logistics operations, such as forecasting, tracking, routing, etc.
- Machine Learning: DHL incorporated ML to predict scenarios like traffic conditions, weather patterns, demand fluctuations, etc, to get insights and recommendations like best routes, schedules, and actions.
- Computer Vision: DHL utilizes computer vision in logistics to scan parcels, labels, and documents better and identify different types using glasses, smartphones, etc.
- Natural Language Processing (NLP): DHL deploys a Natural Language Processing (NLP) model to understand and respond to natural language queries, such as “Where is my package?” and “How do I change my delivery address?” It was trained on a large corpus of logistics-related text.
The Outcome
- DHL deployed 5000 Bots across multiple sites for optimization, increasing collection efficiency by 50% during the initial deployment phase. (DHL)
- DHL’s OptiCarton solution efficiently leverages AI/ML to optimize the filling volume of individual shipments, showing an approximate 50% savings of superfluous shipment space. (DHL)
- In 2024, DHL launched a venture into Generative AI.
- The first outcome was a sophisticated data cleansing tool that ensured DHL engineers had accurate data for designing logistics solutions.
- Secondly, it aimed to support processes in their legal and customer support teams. (DHL)
10 ML Use Cases in Logistics: With Real Examples
Machine learning is revamping the entire logistics industry by unlocking new efficiency and responsiveness levels. ML in logistics is no longer a concept, but a practical tool in delivering substantially profitable results. Read below to know more about how logistics companies have implemented ML into their operations, achieving great ROIs.
Supplier Relationship Management
Dealing with suppliers is considered a challenging part of AI-powered supply chain management. With machine learning in logistics, mutual management becomes easier due to proven and established practices. It helps analyze supplier performance using KPIs like Order Accuracy, Issue Responsiveness, On-time delivery rates, etc.
It also helps enhance supplier collaboration by sharing data-driven feedback with suppliers. A significant moat of ML in this instance is predictive analytics. Based on past behavior, ML models can forecast expected disruptions in the supply chain and external factors like route conditions, weather, and delivery delay reasons.
Real-Life Use Case of ML in Improving Supplier Relationship Management: P&G
P&G has strongly leaned toward integrating AI and ML into its procurement-side operations, focusing specifically on retail execution and supply chain.
Supply chain management with ML results in the inception of its global Supply Chain 3.0 Initiative, which has led to anticipated savings of $200 million to $300 million across various areas.
“It will enable us to serve our joint consumers better while streamlining the end-to-end supply chain, aiming to create a sustainable and expanding competitive advantage.”
By Jon Moeller (P&G’s President and CEO)
Dynamic Freight Pricing
We are familiar with Uber’s dynamic pricing model, where prices fluctuate based on traffic, demand, and driver availability in that particular region/area. In this aspect, logistics companies are becoming a treasure trove of machine learning in the logistics industry. Earlier, multiple companies gauged market volatility by paying higher prices for load covers, yet this can’t be a sustainable practice over the long term.
Dynamic freight pricing takes various factors (current fuel prices, capacity availability, freight volume, demand, and weather & market conditions) into account, revealing the complexity and sophistication of the dynamic pricing model.
Real-Life Use Case of ML in Establishing Dynamic Freight Pricing: Maersk
Customers can search for and get competitive rates online 24/7 through Maersk Spot. Their offline process involves 13 individual steps; Maersk Spot brought it down to 5 simple integrated steps.
In addition, the overall statistics improved drastically. Nearly a 75% improvement was measured when compared before the integration of Maersk Spot. It also brought down the rolling of shipments to just 1.5%. Maersk set a benchmark on how legacy shipment companies can modernize pricing strategies to stay relevant in the digital age.
Smart Inventory Management
Inventory management is a considerable aspect for SMEs because it directly impacts their operational efficiency and financial health. Predictive forecasts, powered by ML, optimize inventory by accurately predicting product demand. How?
The system integrates multiple components, such as data ingestion, storage, and pre-processing, to streamline demand predictions. Effective inventory management also relies on supervising an optimal stock balance. While overstocking ties up capital, understocking leads to sales losses and dissatisfied customers. Due to their multiple locations, large businesses are highly prone to inventory mismanagement.
ML solutions for logistics identify where to efficiently store inventory to meet dynamic needs, subject to shifting stock locations.
Real-Life Use Case of ML in Establishing Smart Inventory Management: Walmart
Walmart has demonstrated the best example of how efficiently an e-commerce company can use AI and machine learning in the logistics industry. They leveraged AI and ML to improve its inventory management systems.
Also Read: [10 Reasons Your Business Needs an Inventory Management Software]
By integrating historical data and predictive analytics, the company optimizes the supply flow of seasonal items across its distribution centers, fulfillment centers, and various other stores, guaranteeing that gifts and festive goods are timely available at competitive prices. Their AI algorithms strategize inventory based on regional differences and sales trends, ensuring availability where needed.
The enhancements in checkout processes have already increased speed and customer satisfaction by 25% and 15%, respectively.
Fuel Consumption Modeling
A study by Amirali Shateri and colleagues from the University of Derby explored the use of AI techniques to identify an optimal ML model for predicting fuel consumption in diesel engines. Various machine learning models were evaluated, such as Neural Networks, Random Forest Regression, and Gaussian Process Regression. The study showed that Gaussian Process Regression had a significant advantage in terms of predictive accuracy.
ML models can predict fuel consumption with high precision by analyzing large volumes of historical and real-time data (vehicle speed, engine load, terrain, and driver behavior).
Real-Life Use Case of ML in Fuel Consumption Modeling: UPS ORION Project
BSR’s case study on UPS’s implementation of ORION (On-Road Integrated Optimization and Navigation) is a classic example of UPS’s aim to implement tech-driven sustainability solutions. Once fully operational across their US routes, an estimated $300 million to $400 million in cost savings were idealized. By addressing specific change-management challenges, UPS ensured a smoother transition and successful integration of ORION into its operations.
Over 70% of routes were tested using this optimization, proving its effectiveness in real-world conditions. This created a milestone for other logistics companies to idealize and harmonize economic performance with environmental responsibility.
Package Condition Detection
Machine learning in logistics is increasingly used to detect package conditions to ensure goods remain intact throughout the shipping process. The supply chain system relies on algorithms to identify features, events, and situations that deviate from the norm, functioning as potential clues to likeable scenarios like fraud, theft, and structural or functional flaws. IoT-enabled sensors collect temperature, vibration, and impact data, which proves helpful in determining whether a package has been mishandled or exposed to damaging conditions.
Pattern Recognition (PR) comes into the picture considering the package detection situation. PR is an algorithm (a predictive ML model) that identifies the pattern in historical data (training data) and then utilizes this trained data to detect changes in regular patterns.
Real-Life Use Case of ML in Package Condition Detection: FedEx SenseAware ID
September 2020 saw the launch of SenseAware ID, a sensor-based logistics device that enhances package tracking and monitoring precision. This new technology aimed to provide real-time updates on packages’ locations within the FedEx Express Network.
The initial rollout of SenseAware ID focused solely on First Overnight shipments within the U.S. domestic network. In addition, the company has launched the FedEx Surround monitoring and intervention tool, which is integrated with SenseAware ID. Packages are tracked hundreds of times versus dozens of times compared to traditional package scanning protocols, thus providing unprecedented real-time data about the shipment’s location.
Intelligent Slotting in Warehouses
Along with the obvious use cases of machine learning in the logistics industry, there will always be new approaches to use it as it progresses. Intelligent Slotting in Warehouses is one of them. Traditional slotting methods are highly labor-intensive and costly due to manual data collection. In contrast, dynamic slotting (powered by AI) is emerging. It allows real-time adaptations of inventory positions based on evolving trends and sales patterns.
This feature is critical in e-commerce, which requires variable, changing inventory. The system aims to achieve quicker order fulfillment times and improved customer satisfaction. The ultimate result is a more agile warehouse ready to meet changing demands.
Real-Life Use Case of ML in Intelligent Slotting in Warehouses: Tandy Brands
Leading leather accessories distributor Tandy Brands transformed its warehouse operations through OptiSlot DC. The tool applied ML algorithms to identify ideal rack configurations for hundreds and thousands of SKUs, resulting in faster warehouse layout decisions. This optimized slot utilization freed up space for other SKUs. Its rule-based logic helped maintain 65% of daily unit volume in 20% of the pick area.
With data-driven insights, uniform racking efficiency increased significantly. Four-shelf racks were eliminated in favor of five-shelf racks, thereby maximizing storage within the same floor space.
Driver Behavior Monitoring
For logistics companies, driver behavior is one critical factor contributing to road accidents, emphasizing the need to integrate machine learning in the supply chain to analyze driver behavior to improve driving patterns. According to ScienceDirect, ML application in this aspect complements its sensor data with weather and traffic information sources from various public APIs, further enriching the dataset for better analysis.
ML algorithms generally collect and interpret data from various sources, like GPS, gyroscopes, accelerometers, dashcams, etc., to assess how drivers operate a vehicle. Over time, fleet managers can use this data effectively to offer targeted coaching, incentivize safe driving, and reduce the risk of accidents.
Real-Life Use Case of ML in Analysing Driver Behavior Monitoring: 3 Men Movers
In 2023, 3 Men Movers, a Houston-based moving company, showcased a good example of the successful integration of ML to improve safety and reduce costs. AI-powered cabin cameras were able to identify driver real-time behaviors with almost 91% accuracy. As a result, 80% of driver distractions were prevented, followed by a 4.5% drop in accident rates in 3 months of system deployment.
Furthermore, AI-based routing further helped in avoiding traffic congestion and high crime areas, improving delivery efficiency and driver safety. [Source: Business Insider]
Dynamic Routing
Route optimization or dynamic routing is reaching new heights, primarily facilitated by machine learning. This AI subset enables algorithms to analyze datasets collected from various sources, both historical and in real time, allowing for improved decision-making and adaptability to changing scenarios. By leveraging these dynamic data sources like traffic patterns, weather forecasts, and, importantly, social media insights, these algorithms can autonomously adjust routes to overcome challenges like road closures and traffic jams.
Machine learning for logistics optimizes routes and includes a feedback mechanism that allows algorithms to learn from operational performance. This iterative learning contributes to an evolving system that becomes more effective over time.
Real-Life Use Case of ML in Dynamic Routing: DPD France
In 2022, DPD France leveraged machine learning for logistics to enhance its delivery operations while elevating customer satisfaction. Together, they implemented a system that optimizes delivery sectorization and route planning.
According to the DPD, the solution saw a 40% difference in volume between the beginning and end of the week. Their Predict home delivery service achieved a 4% improvement in time slot compliance.
Supply Chain Visibility
Traditional supply chains normally experience delays, data silos, and its transparency limits. However, integration of machine learning in the supply chain helps monitor supplier performance through IoT sensors, RFID tags, GPS, etc., to provide a unified view of the supply chain, from the supplier to the end customer. Supply chain visibility is a broad term that involves smaller factors, like risk behavior, traffic patterns, delivery optimization, etc.
Thus, with shared ML-powered dashboards and insights from suppliers, carriers, and partners, companies foster transparency and coordinated response across the entire supply chain. This integration also facilitates feedback loops between predictive models and operational processes. As ML algorithms learn from new feedback, the predictions refine over time.
Real-Life Use Case of Machine Learning in the Supply Chain Visibility: IBM
IBM’s supply chain identified crucial risk categories that required multiple enhancements in their ability to identify, manage, and mitigate disruptions in their supply chain operations. Their “Transparent Supply Chain” initiative was launched to integrate AI and ML into a control tower solution to enhance warehouse visibility and operational efficiency. The results were:
- Inventory levels were reduced to 18%
- Critical supply chain disruption management time went from 18-21 days to hours.
- Achieved more than 95% of serviceable targets.
- Expedited costs reduced by 52%
One significant benefit emerged during the COVID-19 pandemic. The company maintained a 100% order fulfillment rate, thereby demonstrating the effectiveness of its cognitive supply chain.
Return Logistics Optimization
Machine learning in logistics is a powerful tool in optimizing return logistics, thus becoming crucial to supply chain management. It helps analyze return predictions using classification models based on historical return data. The algorithm uses customer data analysis to understand customer return patterns using ML techniques like clustering and natural language processing.
The combined benefit comes as a refined market strategy and improved product design. In addition, some goods categories naturally have comparatively high return rates. ML models help identify the need gaps in those categories, maximizing the optimum decision accuracy on item disposition.
Real-Life Use Case of ML in Return-Logistics Optimization: Zalando
Leading European online fashion retailer Zalando focuses on revolutionizing supply chain management with ML and has effectively utilized AI to counter this business aspect. Their ML model uses historical and real-time data like purchase history, product type, size, and prices, and builds classification models to assign “return probability” to each order. The overall impact is that it allows the adoption of a dynamic strategy.
One notable example is “offering free returns only for low-risk orders”. It collectively helped in inventory planning and customer segmentation. Another use case came out as their “Size Recommendation Engine”. It used data on customer body shape and past order history to provide fit product recommendations, increasing the likelihood of customers selecting the right size.
Major Challenges in Implementing ML in the Logistics Industry: Past the USUAL HYPE
The above info reveals how ML integration is a significant boon for the logistics industry. However, the journey is not without its limitations. One and by far the biggest challenges that arise are:
Challenge | Problem | Solutions |
---|---|---|
Poor Data Quality | Inconsistent, siloed data from GPS, dashcams, etc., limits ML effectiveness. | Build a unified, high-quality data infrastructure with intelligent governance, validation, and synthetic data generation. |
Legacy IT Infrastructures | Outdated systems hinder ML adoption, causing 50% failure rates despite high potential efficiency gains. | Shift to a future-ready mindset, use APIs/microservices for integration, and pilot ML in low-risk, high-impact areas. |
Poor Data Quality
Various ML models collect and analyze a high volume of data regarding traffic patterns and driver behaviors through GPS, dashcams, etc., to better understand existing data. However, the data collected is not always accurate. The majority of logistics companies have to deal with inconsistent and siloed data, which is a significant limitation on their effectiveness.
It is crucial to ensure that data feeding into ML models is not only about abundance but also variation and representational differences. ScienceDirect reveals how various ML approaches have been developed to improve modeling capabilities. Still, data challenges continue to be the main barrier to the role of machine learning in logistics across diverse supply chains.
Solution:
Creating a unified and high-quality data infrastructure is the sole solution to the following challenges of inaccurate and inconsistent data. This high-quality data must be further paired with intelligent data governance and other augmentation strategies. In addition, data validation is crucial. Checking for any potential mishap at the point of ingestion is a good strategy. For example, reporting anomalies like GPS glitches and duplicate logs can be important steps in this regard. Furthermore, generating synthetic databases specific to traffic scenarios and weather conditions can help supplement rare events.
Legacy IT Infrastructures
It’s true that system modernization takes time and is another significant barrier to the widespread adoption of ML in supply chains. The mentality of businesses to consider “If it’s not broken, why fix it?” is one roadblock to the diverse adoption.
Most companies fail to realize how their inability to change their legacy IT systems for a one-time significant upfront cost would lead them to the top ranks of supply chains by improving their operational efficiencies to nearly 90%. Due to legacy IT systems, businesses suffer from a 50% failure rate, yet they fail to recognize it as THE MAJOR and SOLE barrier to their improvement efficacy.
Though initial integration of AI and machine learning for logistics would cost those companies around a value of $1.3 million to $10 million on average, they would cover these costs in 1-2 years, if not months, by improving their internal supply chain visibility problems.
Solution:
In this regard, shifting from “if it’s not broken” to a “becoming future-ready” mindset can help. On an objective front, going for the entire replacement of IT legacy systems can be avoided, and you can start with wrapper solutions like APIs or microservices. This can help in allowing modern tools (powered by ML and AI) to function without entirely replacing your core IT system. Moreover, you can also start with piloting ML in high-impact and low-risk areas, such as in demand forecasting and route optimization.
Future of ML in Logistics
The future of ML in logistics provides businesses with a one-man wrecking crew that nails demand forecasts, sniffs out equipment breakdowns before they hit, dynamically optimizes routes, and smartly manages stock levels to reduce waste.
- Supply chain operations have been significantly transformed with the adoption of. As machine learning models become more advanced, real-time optimization capabilities in the above scenarios will be more prevalent and effective.
- With time, the logistics industry will shift from reactive to proactive decision-making, thus boosting market resilience and customer satisfaction.
- Businesses that adopt ML in their logistics will thrive on the agility to keep pace with volatile, fast-paced markets, thereby gaining operational excellence.
Why Partner with Appinventiv for Your Logistics Solution Needs
Appinventiv, a trusted logistics software development company, combines deep domain expertise with our scalable technology solutions, specifically tailored for the supply chain industry. Our team has hands-on experience delivering bespoke machine learning development services for logistics businesses. These AI/ML-powered solutions can range from real-time fleet tracking to:
- Route Optimization
- Streamlined Warehouse Operations
- Enhanced Supply Chain Control
- Seamless Integration with Existing CRMs
We understand that the logistics industry is currently at a pivotal point. As a logistics company looking forward to the shift from traditional operations to real-time data-driven management, you need tech-driven logistics solutions. And that’s what we offer. We leverage AI and ML algorithms and integrate them into logistics workflows for efficient operations.
We have worked with over 1500 clients in different domains. Read their testimonials to understand how we have showcased our scalability, reliability, and cultural adaptability expertise.
FAQs
Q. What is Machine Learning in Logistics?
A. ML in logistics refers to the application of data-driven algorithms in the logistics supply chain to improve the complexity and variability of modern supply chains. ML is used in demand forecasting, warehouse automation, and predicting potential supply chain disruptions. Multiple historical and real-time data sets are recognized and analyzed via algorithms and further processed to improve operational performance in numerous business aspects like delivery, safety, and supply chain visibility.
Q. Can small or mid-sized logistics companies benefit from ML?
A. Though large organizations experience a wide range of ROIs due to their scale and variability in operations, SMEs can benefit from the niche use of ML applications like demand forecasting or local route optimization to stand out from their competitors locally. This gives the company a competitive edge, potentially increasing customer retention.
Q. How is machine learning used in logistics?
A. Machine learning is extensively used in logistics to optimize operations, enhance efficiency, and reduce costs. It powers demand forecasting by analyzing historical data, market trends, and external factors like weather or holidays to predict inventory needs accurately.
Q. Can ML integration in logistics help maintain a sustainable future?
A. ML helps optimize fuel consumption by selectively suggesting dynamic routes based on traffic, road, and weather conditions. It also helps in minimizing packaging space, leading to less wastage. In addition, ML algorithms can predict vehicle maintenance based on routes and fuel consumption patterns, thereby suggesting timely vehicle service. All these factors collectively result in maintaining sustainability.
Q. Are ML solutions for logistics secure?
A. Reputable ML solutions for logistics incorporate robust security measures, including data encryption and compliance with industry standards, to protect sensitive supply chain information.
Q. What are the major benefits of machine learning in logistics?
A. ML in logistics offers transformative benefits for logistics companies in terms of reduced operational costs, streamlined load distribution, and improved decision-making. Enhanced supply chain visibility and predictive maintenance surface among the top benefits of machine learning in logistics, followed by:
- Personalized Customer Experience (predicting delivery times)
- Warehouse Automation (automation in inventory management, product picking)
- Demand Forecasting (reduced overstocking, improved inventory planning)
- Fraud Detection (detecting unusual patterns in shipping, payments, etc.)
Q. What are the primary machine learning technologies used in logistics?
A. Machine learning technologies used in logistics include predictive analytics for demand forecasting, natural language processing (NLP) for processing shipping documents, computer vision for warehouse automation, and reinforcement learning for optimizing delivery routes. These technologies enhance efficiency, reduce costs, and improve decision-making.
Q. What does machine learning contribute to logistics?
A. Machine learning contributes to logistics by optimizing supply chain operations, predicting demand, improving route planning, and enhancing inventory management. It analyzes vast datasets to identify patterns, forecast delays, and automate decision-making, leading to cost savings, faster deliveries, and increased efficiency.
Q. What are some examples of ML in logistics?
A. Machine learning (ML) is transforming logistics with applications like:
- Demand Forecasting: ML models analyze historical data to predict demand, optimizing inventory levels.
- Route Optimization: Algorithms find the most efficient delivery routes, reducing fuel costs and time.
- Warehouse Automation: ML powers robotic systems for sorting and picking, improving efficiency.
- Predictive Maintenance: ML predicts vehicle or equipment failures, minimizing downtime.
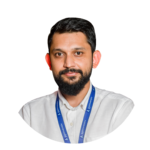
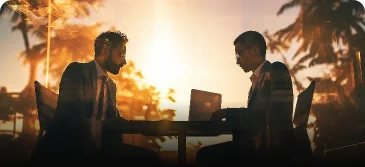
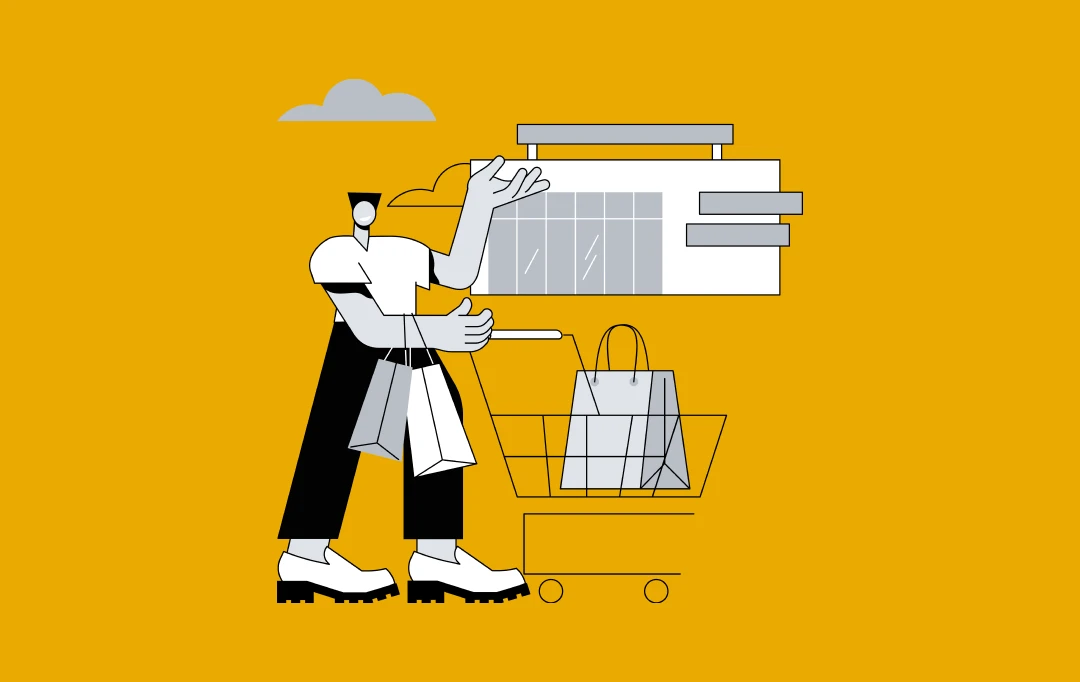
How Machine Learning in Retail is Redefining the Sector - Key Business Opportunities and Challenge
In the last few years, the retail industry has faced some critical challenges like the global pandemic — COVID-19, supply chain disruptions, curfews, energy crunches, sustainability pressures, cybersecurity threats, eCommerce apocalypse, and so on. Despite these obstacles, the industry has shown remarkable resilience, transforming its operations to meet the demands of a rapidly changing retail…
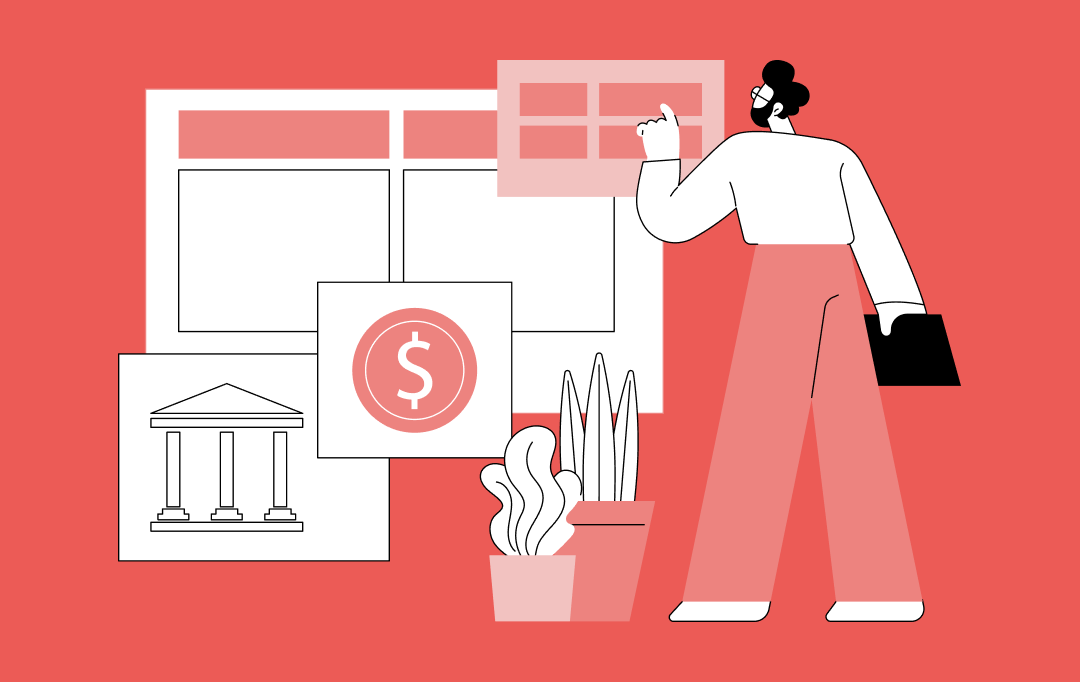
Machine Learning in Banking - Use Cases and Implementation Process
The speed at which fintech transformation is happening is nothing short of a never-seen-before revolution. With the advent of technologies like blockchain, artificial intelligence, metaverse, and edge computing, the fintech space - as we know it - has drastically changed. This transformation's intrinsic change can be seen in how comfortable users have gotten with on-the-move…
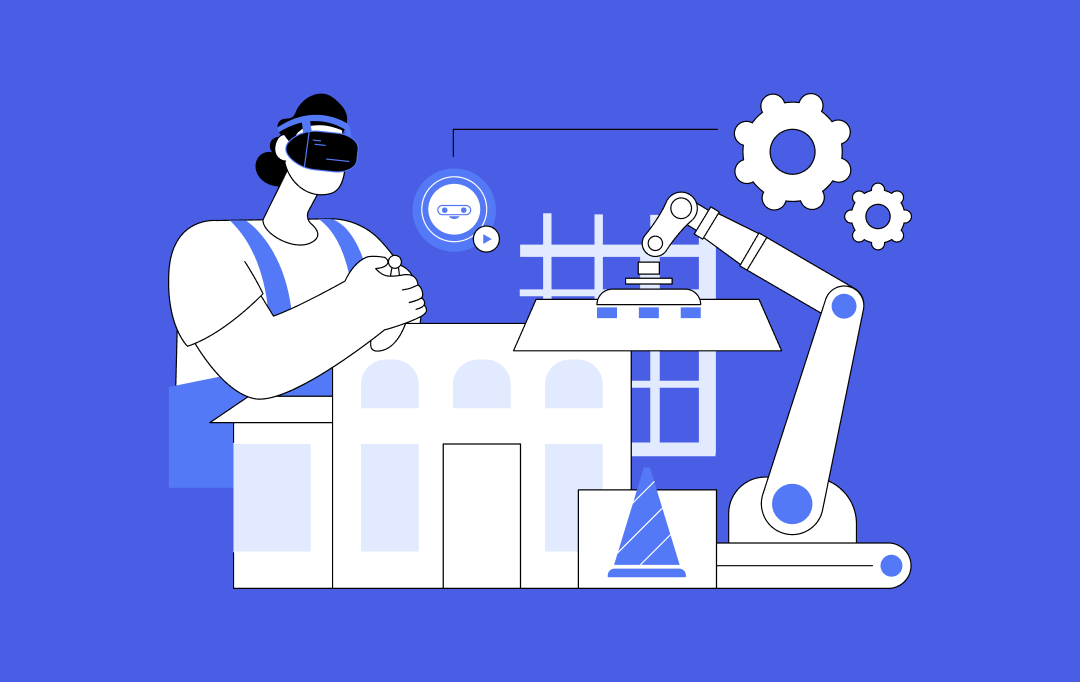
10 Use Cases and Examples of How Machine Learning is Used in Manufacturing
The world is entering the fifth industrial revolution—Industry 5.0—which is giving rise to smart manufacturing that uses artificial intelligence (AI) and machine learning (ML) technologies. Machine learning in manufacturing is not just a buzzword—it is a game-changer. According to MarketsAndMarkets, the Industry 5.0 market is projected to reach $255.7 billion in value by 2029, and…