- Understanding the Role of Data Analytics in Supply Chain Management With Examples
- 1. Predictive Analytics
- 2. Descriptive Analytics
- 3. Prescriptive Analytics
- 4. Cognitive Analytics
- Establish Goals
- Collect and Organize Data
- Data Preparation and Cleaning
- AI Algorithm Selection
- Choose AI Technologies
- Data Modelling
- Integrate with Existing Systems
- Test and Validate
- Pilot Testing and Deployment
- Continuous Improvement
- Top 5 AI and Analytics-Enabled Use Cases to Control Supply Chain Disruption
- 1. Demand Forecasting in Warehouse Supply and Demand Management
- 2. AI for Vehicle Maintenance Recommendations
- 3. AI in Adding Portability to Supply Chain Loading Process
- 4. AI for Cost-Saving and Revenue Boost in Supply Chain
- 5. Data Analytics-Based Strategic Sourcing in Supply Chain
- Benefits of AI in Supply Chain
- Streamlined Warehouse Operations
- Minimized Errors and Waste
- Accurate Inventory Tracking
- Enhanced Safety Standards
- Precise Demand Forecasting
- Deliveries That Run on Time
- Challenges in Implementing AI in Supply Chains and Solutions to Overcome Them
- 1. Data Quality and Availability
- 2. Infrastructure and Technology
- 3. Vendor Choice and Collaboration
- Understanding the Future of AI in supply chain: Best Supply Chain Predictions for 2023 and Beyond
- Make Your Supply Chain Future-Ready With Appinventiv
- FAQs
While several industries are still struggling to overcome the post-pandemic effects, there are a few industries, like supply chain, that took the opportunity to adopt these modern technologies at a large scale.
With the potential to revolutionize processes, decision-making, and overall efficiency, AI is one of the top advanced technologies that businesses must utilize to stay ahead of the curve. Data from Statista shows that AI for the supply chain solution market has resulted in better inventory management, smart manufacturing, dynamic logistic systems, and real-time delivery controls, which has led to its widescale adoption.
The main objective of using AI in the supply chain and logistics is to increase efficiency and productivity. This introduction of AI in supply chain management has led to more sustainability, making every enterprise wonder if digital transformation can benefit their supply chain business.
A recent study conducted by McKinsey says that implementing AI in logistics and supply chain management has led to significant improvements. This demonstrates the potential of artificial intelligence in supply chain management to revolutionize the industry and its importance in the modern business landscape. This blog will help you understand what AI and data analytics in the supply chain can do for your business.
So, let’s move ahead and look at how AI for the supply chain impacts businesses. In addition to this, we will also take a look at how integrating AI development services for your enterprise will bring the workforce, machines, and software into action.
Understanding the Role of Data Analytics in Supply Chain Management With Examples
Using AI and data analytics for supply chain using advanced algorithms and machine learning techniques, businesses can extract valuable insights that help in making informed decisions. Enterprises that use supply chain data analytics solutions can aim to make the supply chain business more:
- Instrumented – machine-generated data flowing out of IoT devices.
- Intelligent – more accurate and competent assumptions with the help of data analytics and modeling.
- Interconnected – extensive connectivity for better decision-making.
The supply chain data analytics solutions help optimize the workflow where large amounts of data can provide forecasting, identify inefficiencies and drive innovation. Here are some of the top supply chain data analytics examples that you can follow to make insightful data-driven decisions for your supply chain business.
1. Predictive Analytics
Predictive analytics is a technique that leverages the power of statistical modeling and regression analysis to identify and understand trends from historical data in order to make predictions about future trends.
AI in logistics and supply chain helps companies predict the most likely future outcome and its business implications. For instance, using predictive analytics to mitigate risks and disruptions.
2. Descriptive Analytics
Descriptive analytics is a form of data mining that involves the analysis of large datasets to identify patterns and generate summaries that allow users to gain insight into a given situation. This type of analytics utilizes historical data to uncover trends and draw conclusions that can be used to inform decision-making.
Descriptive analytics is another example that can help you understand the importance of data analytics in the supply chain. This helps provide visibility and certainty to all kinds of internal and external data across the supply chain management.
3. Prescriptive Analytics
Prescriptive analytics is a powerful tool for supply chain operations, allowing for the exploration of how specific changes will affect outcomes. By implementing artificial intelligence for supply chain, potential improvements can be identified and recommended, providing a valuable resource for optimizing supply chain operations.
This includes collaborating with logistic partners to reduce time and effort for maximum business value. One popular reference is the use of SRM (Supplier relationship management) as a prescriptive analytic approach.
4. Cognitive Analytics
If you wish to understand advanced analytics in supply chain management, cognitive analytics is the way to go. This is best used in enhancing customer experience and relationships. The feedback data received through AI-driven systems is analyzed and executed in reports and dashboards to answer complex questions.
This application of AI in supply chain can automatically allow your business to pursue breakthrough ideas and provide better customer needs and demands. If you still haven’t decided on embracing the use of analytics in the supply chain for your business, our next point of discussion is for you.
Also Read: How AI in Manufacturing is Revolutionizing the Industry: Key Use Cases and Examples
Key Steps to Optimize Data Analytics and Artificial Intelligence in Supply Chain
Depending on each organization’s unique needs, available resources, and industrial environment, the implementation journey for AI/ML in supply chain may differ. However, here are some of the common steps that a supply chain AI solutions provider would follow to successfully implement AI in supply chain.
Establish Goals
A reputed artificial intelligence services company like Appinventiv first begins with establishing the goals you hope to accomplish by integrating data analytics and AI in supply chain. The AI/ML experts, after discussion with the stakeholder, determine which particular fields, such as demand forecasting, inventory optimization, route optimization, or risk management, AI can be utilized in.
Collect and Organize Data
Next, the experts gather pertinent information from various sources throughout your supply chain, such as previous sales data, customer information, inventory records, logistical information, and external data sources, such as market trends and weather patterns, and organize them.
Data Preparation and Cleaning
Raw data frequently has errors, inconsistencies, or missing values. The data must be cleansed and prepared before AI algorithms can examine it efficiently. This entails activities including eliminating duplicates, fixing mistakes, addressing missing data, and formatting the data appropriately.
AI Algorithm Selection
In this stage, the experts choose the right AI algorithms to address certain supply chain challenges based on the outlined objectives. Regression, classification, clustering, or deep learning methods for complicated pattern identification may be used in this case.
Choose AI Technologies
In this stage, the supply chain data analytics software development experts would help you to choose the AI tools and methods compatible with your goals and available data. This could involve identifying the right AI technologies like robotic process automation, computer vision, natural language processing, machine learning, or predictive analytics.
Data Modelling
Data modeling is a crucial process that requires the careful selection of the right set of machine learning algorithms. Our team of data scientists experiments with various data sources by transforming them and constructing features that can best explain the variability in the data. This means that your organization can leverage the power of algorithms such as Seq-Seq and Auto-Encoders to generate forecasts.
However, it is important to note that all AI algorithms are based on specific mathematical assumptions. Therefore, it is crucial to prepare the data in a certain way to cater to these assumptions.
Integrate with Existing Systems
Now, the experts would integrate AI capabilities into the infrastructure and technologies that now run your supply chain. In order to do this, enterprise resource planning (ERP), warehouse management (WMS), transportation management (TMS), or other pertinent software may need to be linked with AI models. The experts would ensure that the systems’ integration is seamless and permits data transfer.
Test and Validate
In this stage, the experts put your AI models and linked systems through thorough testing and validation. By contrasting forecasts or suggestions with actual results, you may confirm the precision, dependability, and performance of the AI algorithms. Depending on the testing outcomes, the QA experts iterate and improve the models.
Pilot Testing and Deployment
It is highly recommended to conduct pilot testing and deployment on a smaller scale before implementing AI solutions across the entire supply chain. This approach allows for effective evaluation of the AI system, identification of any issues or areas of improvement, and fine-tuning of the algorithms.
By doing so, AI/ML experts ensure the success of your AI for the supply chain optimization and implementation. They take the necessary steps to pilot-test your AI for the supply chain solution and reap the benefits of a streamlined supply chain.
Continuous Improvement
Supply chain AI implementation is a continuous process. Evaluate how artificial intelligence in supply chain is transforming your business processes over time. Make necessary changes in your AI supply chain management to increase productivity, accuracy, and decision-making. Keep abreast of current AI breakthroughs and look at supply chain innovation and optimization prospects.
Top 5 AI and Analytics-Enabled Use Cases to Control Supply Chain Disruption
It’s time for modern supply chain enterprises to empower their business with reliable and automated data visual analytics platforms. You can refer to these AI use cases in supply chain to minimize the supply chain disruption and make the most out of your business.
1. Demand Forecasting in Warehouse Supply and Demand Management
This data-rich modeling method is by far the best use case of data science and AI for demand forecasts that empower warehouse employees to make more informed decisions on inventory stocking.
2. AI for Vehicle Maintenance Recommendations
IoT device data is generated from in-transit vehicles to deliver real-time insights on the longevity of the transport vehicles. The machine learning systems integrated into the vehicles make maintenance recommendations and failure predictions based on past data. This will allow you to take fleeting vehicles out of the chain before the performance issue causes any kind of delay in the deliveries.
3. AI in Adding Portability to Supply Chain Loading Process
Supply chain management comes with a great deal of detail-oriented analysis, including how shipments and goods are loaded and unloaded from the shipping containers. Both data modeling and AI precision are needed to determine the most efficient ways to get the goods on and off the containers.
Modern supply chain companies use a combination of software, hardware, and supply chain data analytics to get hands-on real-time visibility into the loading process. The gathered data can also be used to design less risky and quick process protocols to manage parcels.
4. AI for Cost-Saving and Revenue Boost in Supply Chain
When it comes to the ultimate cost-saving, AI and analytics solutions are the most effective options to negotiate better shipping and procurement rates, pinpoint changes in the supply chain profit process and manage courier contracts. You can assess a centralized database that takes virtually every aspect of the supply chain to deliver financial decision-making.
In general, advanced analytics in supply chain management is paving the way for new innovations where platforms are used for mining and analyzing cost-effective revenue-building standards. A Bloomberg report suggests that in the past two years, the overall cost in the supply chain has reduced to 12% leading to profits.
5. Data Analytics-Based Strategic Sourcing in Supply Chain
The most underrated application of AI in supply chain industry is the identification of critical suppliers and strategic partners. This helps you standardize lower-cost alternatives and predicate supply performance indicators for compliance.
Let’s now move on to discuss the challenges of leveraging artificial intelligence in supply chain management.
Benefits of AI in Supply Chain
The integration of AI in the supply chain brings numerous benefits to businesses, helping them enhance productivity and agility. Here are some of the most common benefits of AI in supply chain:
Streamlined Warehouse Operations
AI in the supply chain makes warehouse operations faster and more efficient by automating tasks like sorting and tracking goods. This helps speed up workflows and enhances productivity.
Minimized Errors and Waste
By leveraging advanced analytics and machine learning algorithms, AI minimizes human errors in operations and reduces material wastage. It results in a more sustainable and profitable supply chain.
Accurate Inventory Tracking
AI provides a crystal-clear view of inventory levels in real time. It helps businesses stay safe from overstock or stockout situations. AI in inventory management is all about keeping things balanced and customers happy.
Enhanced Safety Standards
Artificial intelligence in the supply chain also helps enhance workers’ safety. How? By monitoring conditions and predicting risks in real time, AI prevents feasible accidents. It ensures both employees and goods are handled with care.
Precise Demand Forecasting
AI doesn’t just look at what has happened in the past; it also anticipates future trends. This capability of AI helps businesses prepare for sudden demand shifts and keep operations running smoothly.
Related Article: AI-Based Demand Forecasting: Optimizing Supply Chains
Deliveries That Run on Time
AI takes the guesswork out of logistics by optimizing routes and predicting potential delays. AI in logistics prioritizes shipments based on order volumes, contractual deadlines, delivery promises, and product availability. It ensures goods reach their destination on time.
Challenges in Implementing AI in Supply Chains and Solutions to Overcome Them
Adding AI to the supply chain into the current business systems presents a number of challenges. The following are some typical challenges and solutions for them:
1. Data Quality and Availability
Challenge: For AI algorithms to produce correct findings, a significant amount of high-quality data must be made available. However, it may be difficult to effectively implement AI models due to the possibility that supply chain data is inconsistent, incomplete, or of low quality.
Solution: To increase data quality, spend money on data cleaning and validation procedures. Implement data governance procedures to guarantee the accuracy and consistency of your data. To increase data availability, look into partnerships and other data sources.
2. Infrastructure and Technology
Challenge: Technical issues may arise when integrating AI into current supply chain systems. The seamless integration of artificial intelligence in supply chain might be hampered by outdated systems, incompatible platforms, and inadequate IT infrastructure.
Solution: Conduct a thorough technology audit to pinpoint any gaps and requirements for integrating AI. Upgrade your infrastructure and think about implementing cloud-based solutions for scalability. Work together with technology partners and IT teams to guarantee seamless integration and reduce disruptions.
3. Vendor Choice and Collaboration
Challenge: To ensure compatibility, dependability, and long-term partnerships, working with external vendors, AI solution providers, or technological partners involves rigorous selection and review.
Solution: Select respectable and trustworthy partners that offer scalable generative AI services by conducting in-depth research, due diligence, and vendor reviews. Ensure your requirements and expectations are crystal clear, and create effective communication routes. Maintain a feedback loop and engage in continuous communication to address any problems or issues that may come up.
Organizations may overcome barriers to deploying AI in supply chain and realize its promise for enhanced efficiency, decision-making, and competitiveness by proactively addressing these issues and putting the recommended techniques into practice.
Understanding the Future of AI in supply chain: Best Supply Chain Predictions for 2023 and Beyond
The integration of AI in supply chain has truly revolutionized the way businesses operate. And the exciting part is that this is just the beginning. As we look ahead to the future of AI in logistics and supply chain, we see a world of possibilities.
- With predictive analytics, companies can now accurately forecast demand, optimize inventory levels, and improve efficiency.
- Autonomous vehicles and drones are taking logistics to the next level with faster deliveries and enhanced safety measures.
- AI-driven robotics in warehouses are boosting productivity and reducing costs.
By embracing artificial intelligence in logistics and the supply chain, businesses can drive operational efficiencies, enhance customer experiences, and gain a competitive edge in the global marketplace.
Now that you have looked at the various future predictions for the use of AI in supply chain let us move ahead and discuss how Appinventiv’s AI development services can streamline your logistics and supply chain business.
Make Your Supply Chain Future-Ready With Appinventiv
The benefits of AI in supply chain management are indispensable. Being a commonplace technology enterprises of all sizes are now embracing the applications of artificial intelligence in logistics and supply chain. Given the current scenario, every supply chain business needs to be critically integrated with supply chain AI solutions for optimization.
Therefore, If your supply chain business lacks the above-discussed modern solutions, it’s high time to choose Appinventiv’s data analytics services to help you derive valuable insights from your business data for maximum scalability and performance. We can empower your business with the best possible services, from supply chain analytics and data visualization to data warehousing and business intelligence solutions.
Get in touch with our team of developers to explore and deep dive into the benefits of AI for your supply chain business.
FAQs
Q. What role does analytics play in supply chain optimization?
A. Analytics is essential to supply chain optimization because it helps decision-makers use data and insights to make better decisions and increase efficiency. The following are some essential ways that analytics aids supply chain optimization:
- Demand forecasting
- Inventory management
- Supply network optimization
- Risk management
- Performance measurement
Q. How AI and machine learning are applied in the supply chain?
A. AI and ML are applied in the supply chain ecosystem with the help of advanced algorithms. The role of artificial intelligence in supply chain solutions will be to enhance the quality of data and offer you a wholly redefined overview of the warehouse and supply chain. It can further help you predict the demands and help in restoring the optimal stock levels promptly. A dedicated AI development services organization like Appinventiv can help you integrate AI/ML in your supply chain management software effectively.
Q. What are some examples of AI and analytics in the supply chain?
A. Here are some of the top supply chain data analytics examples that help to drive optimization, aid in the decision-making process, and improve efficiency:
- Demand forecasting
- Warehouse automation
- Route optimization
- Supply chain risk management
- Predictive maintenance
- Supplier management
- Real-time supply chain visibility
Q. How is AI being used in supply chain?
A. AI is transforming supply chains by improving demand forecasting, optimizing inventory control, and streamlining logistics operations. It leverages machine learning to anticipate trends and automate procurement processes. Advanced analytics enhance route planning and cut operational costs, while AI-driven automation in warehouses and real-time data analysis increase overall efficiency and precision in supply chain management.
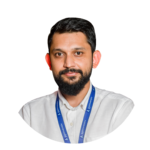
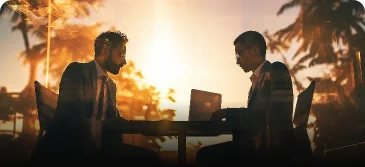
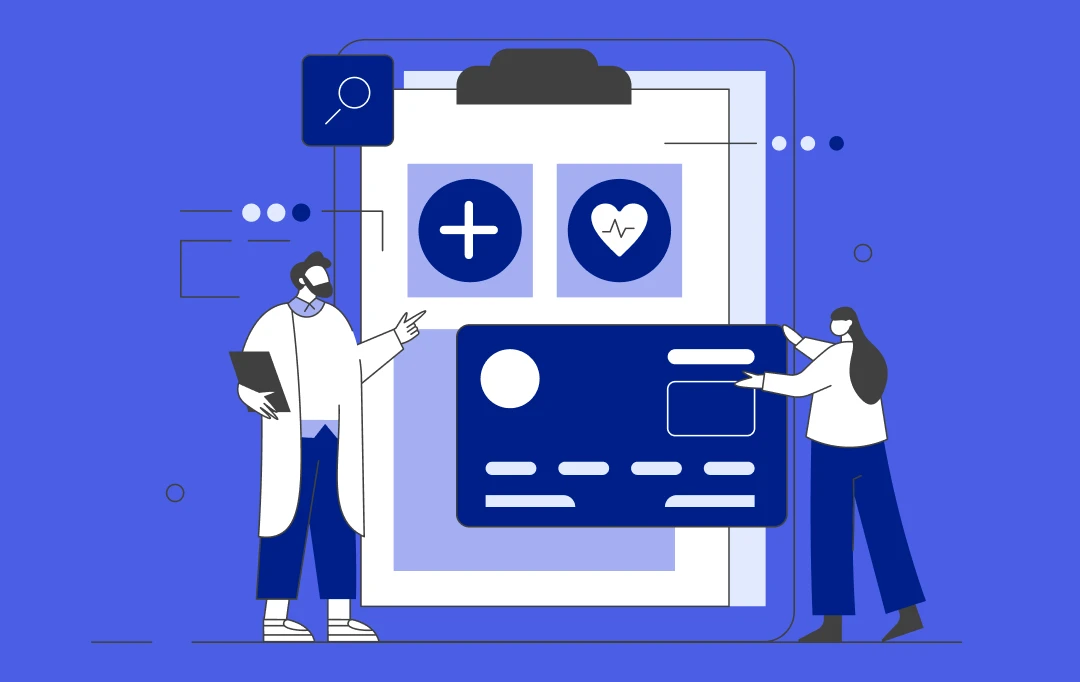
10 Ways AI is Transforming the Healthcare Sector in Australia
Key takeaways: Generative AI could add $13 billion annually to Australia’s healthcare sector by 2030. AI is already transforming operations across diagnostics, admin tasks, and patient care. Healthcare in remote areas is improving with AI-powered virtual assistants and monitoring tools. AI can help address workforce shortages by automating routine tasks and supporting clinical decisions. Major…
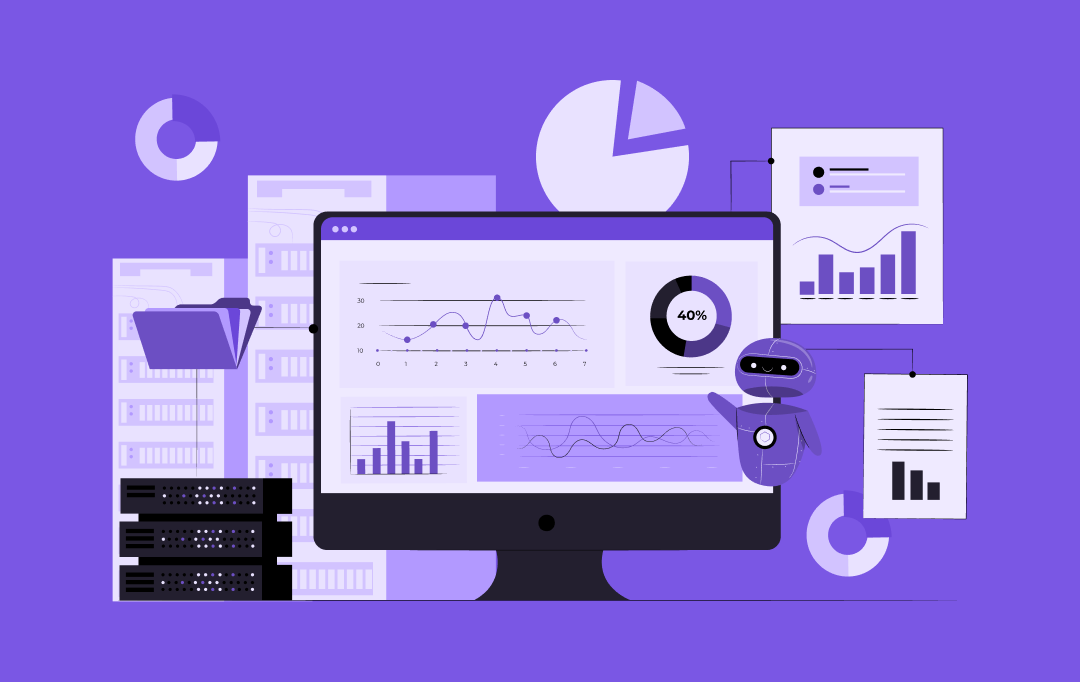
Exploring the Power of AI in Creating Dynamic & Interactive Data Visualizations
Key takeaways: AI transforms data visualization by providing automated insights, interactive dashboards, and NLP, thereby tackling revenue loss and improving data quality. Key features include predictive analytics, real-time data integration, and user-friendly visualizations, enabling faster and more informed decisions. Top 2025 tools, such as Tableau, Power BI, and Julius AI, offer NLP and real-time analytics…
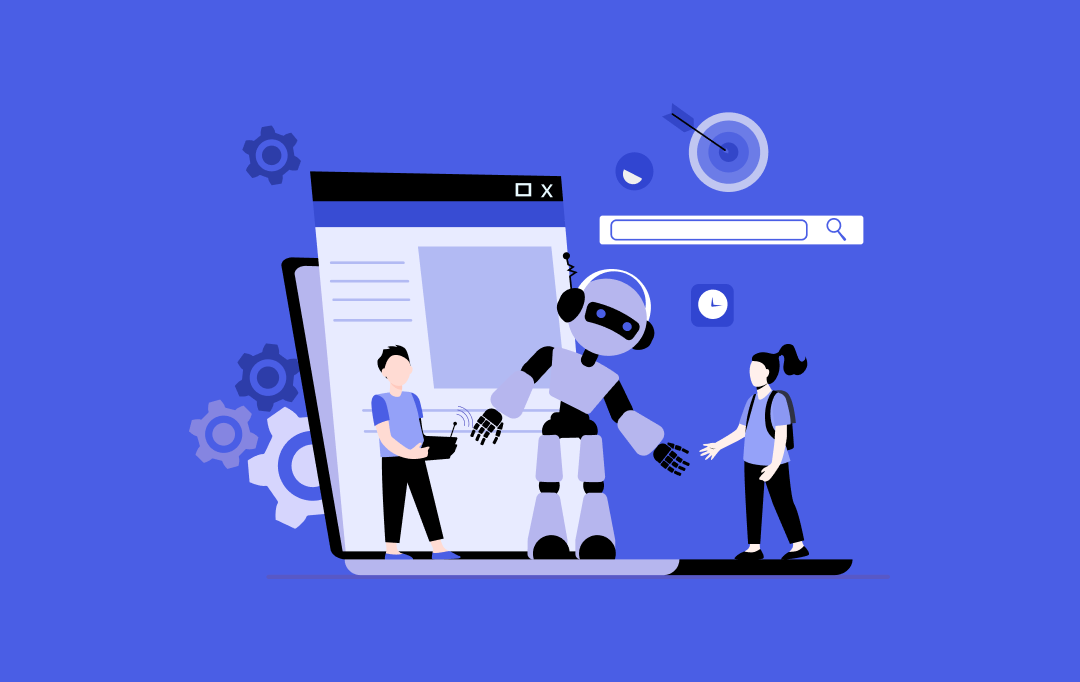
Practical Applications of AI as a Service for Your Business
Key takeaways: AIaaS enables fast, infrastructure-free AI adoption. Used across industries for automation and insights. Real-world examples show proven business impact. Pilot-first approach ensures smooth implementation. Key challenges include data, cost, and scaling. Trends include ethical AI, edge, and SME growth Imagine being able to cut down your operational costs while simultaneously improving decision-making and…