- Understanding the Core Components of AI Applications
- Core Components of AI
- Benefits of Building an AI App for Businesses
- Intellectual Property Creation
- Control Over Data
- Adaptability and Agility
- Cost Efficiency in the Long Run
- Enhanced Security Measures
- Future-Proofing the Business
- How to build an AI App - A Step-by-Step Guide
- Identify Problems and Define Objectives
- Choose the AI Model
- Choose the Right Tech Stack
- Collect and Prepare Data
- Train the AI Model
- Design the UI/UX
- Develop the App
- Integrate the AI Model into the App
- Test the AI App
- Deploy and Maintain
- Game-Changing Features of an AI App
- Personalized Recommendation
- Chatbots and Voice Assistants
- Auto-Correction and Completion
- Predictive Analytics
- Health Monitoring
- Visual Search
- Language Translation
- Gesture Recognition
- Facial Recognition
- Image and Sticker Generation
- Text to Video Creation
- Sentiment Analysis
- Security and Compliance
- Real-Time Data Processing
- How Much Does It Cost to Build an AI App?
- Factors Affecting the Cost to Build an AI App
- Future Trends in AI Application Development
- AI and IoT Convergence
- Enhanced Personalization
- Integration of Generative AI
- Explainable AI and Responsible AI
- Increased Focus on Edge AI
- Autonomous AI Agents
- No-Code and Low-code AI App Development
- Human-AI Collaboration
- Integration of RAG in AI
- Quantum Computing in AI
- How to Build an App with AI: Navigating Challenges and Finding Solutions
- Data Quality and Quantity
- Integration with Existing Systems
- Ethical and Privacy Concerns
- Skill Gap and Talent Acquisition
- Cost of Implementation
- Managing Expectations
- Algorithmic Bias and Fairness
- Scaling AI Solutions
- Technology Obsolescence
- Industry-Wise Use Cases of an AI Application
- Healthcare — Predictive Analytics for Patient Care
- Transportation — Autonomous Vehicles
- Real Estate — Property Valuation and Market Analysis
- Retail — Personalized Shopping Experiences
- Finance — Budget Management
- Manufacturing — Predictive Maintenance
- How can Appinventiv Help You Build an AI App That Makes an Impact?
- FAQs
Artificial intelligence (AI) apps have become inseparable from the modern business world, redefining operations and driving innovation across various industries. According to a report by Investment News, the mobile AI app sector generated $2 billion in 2024.
Furthermore, the market is expected to grow to $18.8 billion by 2028. In June 2024 alone, an estimated 230 million people used AI apps, highlighting their widespread adoption and transformative potential in our personal and professional lives.
In this era of swift digital transformation, AI has become a pivotal force reshaping how companies operate and compete. Organizations can streamline processes, enhance decision-making, and uncover new revenue opportunities by harnessing the power of machine learning, predictive analytics, and automation.
This surge in AI adoption underscores the importance of building an AI app that is both scalable and adaptable to evolving business needs, ultimately helping enterprises thrive in today’s rapidly changing market.
Beyond consumer conveniences, AI apps drive innovation in nearly every industry, from healthcare and finance to retail and manufacturing. Voice assistants like Siri, Alexa, and Google Assistant illustrate how AI can reshape user engagement, while platforms like Netflix and Spotify leverage sophisticated algorithms to personalize experiences.
Moreover, generative AI models like ChatGPT and Gemini AI continually push the boundaries of productivity and creativity, opening up unprecedented opportunities for businesses to stand out in today’s competitive marketplace.
Businesses leveraging AI into their applications are gaining a competitive edge, setting new innovation and customer engagement standards. However, building an app with artificial intelligence is not easy, leaving businesses wondering how to create an AI app that can make a real impact and drive the intended outcome.
Well, worry not! This comprehensive guide will help you understand how to make an AI app, address all your AI dilemmas, and help you navigate the process from start to finish. It covers transformative applications, examples, development steps, key features, associated costs, and much more, providing you with the in-depth knowledge essential to build an app with AI.
Let us build a future-proof app that elevates your ops and drives remarkable growth.
Understanding the Core Components of AI Applications
AI, in its most basic form, refers to the emulation of human intelligence in machines, allowing them to think and learn like humans. Thus, building AI applications involves creating a computer program capable of performing a multitude of tasks that typically require human intelligence. Reasoning, understanding natural language, analyzing sentiments, learning from experience, detecting anomalies, recognizing patterns, and making decisions are some of the most notable tasks performed by AI apps.
To gain an in-depth understanding of how to create an artificial intelligence app, it is crucial to understand the core components of artificial intelligence and their notable applications in various scenarios.
Core Components of AI
AI is a multifaceted field that encompasses various components and elements. Understanding the working mechanism of these components will give you a clear picture of how to build a custom AI application and how they will work. Here are the different integral elements of AI:
Machine Learning (ML)
Machine Learning is a core component of AI that allows systems to learn from data and enhance their performance over time without explicit programming. It involves algorithms that can analyze and interpret complex data patterns to make predictions or decisions. Its working mechanism includes:
- Supervised Learning: Learning from labeled data to make predictions, such as detecting spam emails.
- Unsupervised Learning: Identifying patterns in unlabeled data. It is used for clustering and association tasks, such as customer segmentation.
- Reinforcement Learning: Interacting with an environment and receiving feedback in the form of rewards or penalties. It is commonly used in robotics and game AI.
Neural Networks
Neural networks, a branch of AI inspired by neurology, simulate the human brain by mimicking its vast network of neurons. In a simple term, neural networks are computational models that combine cognitive science with machines to perform tasks like recognizing patterns and making decisions. Its working mechanism includes:
- Artificial Neural Networks (ANNs): Basic neural networks used for simple pattern recognition tasks, such as image and speech recognition.
- Convolutional Neural Networks (CNNs): Specialized for processing grid-like data such as images and videos, essential in facial recognition and self-driving cars.
- Recurrent Neural Networks (RNNs): Designed to handle sequential data, used in applications like language modeling and time-series forecasting.
Deep Learning
Deep Learning is basically a subset of machine learning that uses neural networks with many layers (deep neural networks). It excels in learning from large amounts of unstructured data. Its working mechanism includes:
- Speech Recognition: Transcribing spoken language into text with high accuracy.
- Image and Video Processing: Enhancing and recognizing patterns in visual content.
- Natural Language Understanding: Improving the accuracy of NLP tasks such as translation and sentiment analysis.
Also Read: Cost of developing an AI Voice and TTS app like Speechify
Natural Language Processing (NLP)
NLP facilitates the interaction between computers and humans in natural language. It enables machines to understand, interpret, and generate human language. Its working mechanism includes:
- Text Analysis: Extracting meaningful information from text data, such as identifying customer sentiment from reviews.
- Machine Translation: Automatically translating text from one language to another, such as Google Translate.
- Speech Recognition: Converting spoken language into text, used in intelligent voice assistants like Siri, Alexa, and Tootle.
Computer Vision
Computer Vision enables machines to interpret and make decisions based on visual inputs from the world. It involves image processing and pattern recognition technologies to understand and analyze images and videos. Its working mechanism includes:
- Image Recognition: Identifying objects, individuals, and scenes in images, such as tagging photos on social media.
- Object Detection: Locating and identifying multiple objects within an image as seen in autonomous vehicles and security surveillance.
- Image Segmentation: Computer vision in healthcare helps in dividing an image into segments for easier analysis. This aids in advanced medical imaging and object recognition.
Robotics
Robotics combines AI with mechanical engineering to create machines capable of performing tasks autonomously or semi-autonomously with minimal human intervention. These robots can be used in manufacturing, healthcare, agriculture, and logistics to improve efficiency and accuracy. Its working mechanism includes:
- Robotic Process Automation (RPA): Automating repetitive tasks in business processes like data entry and customer service.
- Autonomous Robots: Robots that can perform tasks without human intervention, such as AI drones and robotic vacuum cleaners.
- Collaborative Robots (Cobots): Robots designed to work alongside humans in environments like manufacturing and healthcare.
Expert Systems
Expert systems emulate the decision-making abilities of a human expert. They use a combination of knowledge and rules to solve specific problems in fields such as medical diagnosis, financial forecasting, and customer support. Its working mechanism includes:
- Knowledge Base: Containing a repository of specialized information, facts, and rules about a particular domain, such as a medical database for diagnosis.
- Inference Engine: Applying logical rules to the knowledge base to draw conclusions or make decisions.
- User Interface: Allowing users to interact with the system, input data and receive guidance or solutions.
Fuzzy Logic
Fuzzy Logic is a method of reasoning that deals with approximate rather than fixed and exact reasoning. It is used in systems that need to make decisions based on imprecise or uncertain information. Its working mechanism includes:
- Control Systems: Managing complex systems like climate control in buildings.
- Pattern Recognition: Identifying patterns in data where traditional binary logic falls short.
Understanding these elements of AI provides a comprehensive view of how AI systems function, how various technologies are used to perform multiple tasks, and, most importantly, how to build an AI app that exceeds expectations.
Benefits of Building an AI App for Businesses
Building an AI app can benefit businesses looking to innovate and streamline their operations. These applications enhance efficiency and provide significant competitive advantages in today’s fast-paced market environment.
Intellectual Property Creation
Building an AI app can contribute to a company’s intellectual property assets, providing long-term value. Owning the IP gives a competitive edge and opens up additional revenue streams such as licensing or selling the technology.
Control Over Data
By developing an AI app, businesses maintain complete control over their data ecosystems. This control is crucial for complying with data protection regulations and utilizing proprietary data without compromising security or privacy.
Adaptability and Agility
Having your own AI app enables quicker adaptations to changes in the business environment or market. This agility ensures businesses can respond to emerging trends or operational challenges more effectively.
Cost Efficiency in the Long Run
While the initial development cost might be high, owning an AI app can lead to significant cost savings over time by automating tasks, optimizing resources, and reducing reliance on third-party software solutions.
Enhanced Security Measures
Custom AI apps can be designed with advanced security features tailored to the specific risks faced by the business. This can provide stronger protection of business data and operations than off-the-shelf software might offer.
Future-Proofing the Business
Investing in AI app development prepares businesses for the future. As AI technology evolves, having a dedicated AI app allows for seamless integration of new functionalities and technologies, keeping the company at the forefront of innovation. For instance, if you wish to launch an AI-powered dating app that continuously refines match suggestions based on user behavior, or develop a smart personal finance app that analyzes spending patterns in real-time and offers tailored insights, an AI-first approach enables constant evolution. This ensures your business remains adaptable, competitive, and ready to embrace emerging trends as they shape the market.
How to build an AI App – A Step-by-Step Guide
Building AI applications is a complex and challenging process requiring advanced technologies such as deep learning, machine learning, natural language processing (NLP), data science, computer vision, robotics, and more.
However, following the streamlined steps to build an AI app, you can transform your innovative concept into a robust, real-world AI application that drives transformative results.
Identify Problems and Define Objectives
The first step in developing an AI app is identifying the specific problem you want to solve. This involves understanding the needs and pain points of your target audience. Once you know your target market’s problem, define your AI app’s objectives, and determine how the app will add value to your users and address their pain points.
Choose the AI Model
You can consider choosing AI models like BERT, GPT-3.5, T5, LLaMA, Gemini, PaLM, Mistral, Orca, InstructGPT, Flan, RoBERTa, etc., based on the specific news of your AI platform. Each of these models offers unique features and functionalities, ensuring the success of your AI project.
So, choose the one that aligns with your objectives, or consider building a custom model if your requirements are highly specialized. Here is a table defining the use cases of some popular AI models:
AI Model | Use Cases |
---|---|
BERT | Ideal for tasks such as named entity recognition, sentiment analysis, and word classification, providing deep contextual understanding. |
T5 | Versatile model used for translation, text summarization, and answering questions by converting tasks into a text-to-text format. |
GPT-3.5 | With its advanced language capabilities, thai model is an excellent choice for generating content, and providing coding assistance |
Mistral | Specializes in understanding and summarizing documents, enhancing text processing efficiency. |
PaLM | Effective for complex task reasoning, coding, and both text and word classification, as well as question answering. |
InstructGPT | Focuses on task-based instructions and predicting user intent, improving interactions with AI systems. |
RoBERTa | Enhances text generation and captures long-range dependencies and contextual nuances in language. |
XLM | Provides robust multilingual capabilities |
XLNet | Optimized for sentiment analysis, question answering, and natural language understanding with an autoregressive approach. |
Turing NLG | Designed for creating chatbots and conversational AI applications, generating natural and engaging dialogues. |
Phi | Compact and efficient, specializing in Python coding |
Alpaca | Excels in text generation, Q&A, summarization, and various other NLP tasks. |
Bloom | Strong in QA, text generation, and generating embeddings for diverse applications. |
Vicuna | Useful for text generation, answering questions, and summarizing content, along with other NLP tasks. |
Choose the Right Tech Stack
When considering how to build an AI app for Android or iOS, you must choose the right AI tech stack that is best suited for your app’s requirements. Consider using the right mobile frameworks, cloud services, and AI platforms like Google Cloud AI, Amazon Web Services (AWS), or Microsoft Azure to leverage their robust infrastructure and AI capabilities.
You may like reading: AWS Vs Azure Vs Google Cloud – Which cloud platform should you choose for your enterprise?
Collect and Prepare Data
Data is the backbone of any AI application. Thus, you need to invest significant time and effort in collecting relevant data from diverse sources. Cleanse and preprocess the collected data to ensure only high-quality data is used to train your AI models.
Train the AI Model
Once you have collected and prepared the data, the next step is to train the model using this data and optimize its performance. Training involves feeding the model large datasets and adjusting its parameters to improve accuracy and efficiency. Popular frameworks for training AI models include TensorFlow, PyTorch, Keras, and Hugging Face Transformers.
Related Article: How to Build an Intelligent AI Model: An Enterprise Guide
Design the UI/UX
The next step is to craft a user-friendly and intuitive interface that is easy to navigate and can capture attention. A well-crafted UI/UX not only enhances user engagement but also increases retention and conversion rates.
Develop the App
With your data and design ready, it is time to develop an AI app and bring your vision to reality. At this stage, your AI app development company focuses on both frontend and backend development. The frontend involves creating a user-friendly interface that allows users to interact seamlessly with your app. The backend development encompasses building and integrating the server-side components, including the AI model, database management, and security measures.
Integrate the AI Model into the App
Now, it is time to integrate the trained model into the app’s architecture, enabling it to perform its intended functions in real-time. This process includes connecting the model with the app’s frontend and backend components, ensuring that data flows seamlessly between the user interface and the AI system.
Test the AI App
Before you deploy your app to the targeted platform, you must conduct thorough testing to ensure that it functions optimally and meets the defined goals. Test the app at different parameters to ensure its quality, usability, security, and overall functionality. This includes unit testing, integration testing, User Acceptance Testing (UAT), performance testing and security testing. This step is essential to identifying and fixing any issues before the app is released.
Deploy and Maintain
After the AI app development process marks an end and is checked for quality, it is deployed to the desired platforms, such as iOS, Android, or the web. After deployment, continuously monitor and maintain the AI app to keep it running smoothly per changing user needs and technological advancements.
After looking into the steps to build an AI app, let us move ahead and look into the features of an AI app in detail below.
Partner with us to develop AI applications that drive efficiency and innovation
Game-Changing Features of an AI App
Building AI applications requires incorporating features that can significantly enhance user experience, drive engagement, and streamline operations. While the specific features of an AI app can vary based on the industry and project requirements, certain capabilities are universally valuable. Here are some common AI app development features that will make your apps stand out in today’s competitive market.
Personalized Recommendation
An AI-powered personalized recommendation system analyzes user behavior, preferences, and past interactions to suggest tailored content, products, or services. This feature enhances user engagement, leading to increased user retention and higher conversion rates.
Chatbots and Voice Assistants
AI-powered chatbots and voice assistants provide instant, 24/7 customer support. They can efficiently handle a wide range of queries, freeing up human resources for more complex, value-added tasks.
Auto-Correction and Completion
The auto-correction and text completion feature assists users by automatically correcting spelling errors and predicting the next word or phrase. These functionalities improve typing speed, efficiency, and accuracy, making communication faster and reducing errors. This feature is especially beneficial in social media messaging apps and word processors.
Predictive Analytics
Predictive analytics in AI apps analyzes historical data and predicts future outcomes, helping businesses anticipate trends, optimize strategies, and improve performance. This feature is invaluable for data-driven decision-making in various industries, including finance, healthcare, retail, and marketing.
Health Monitoring
To build an AI app that offers it all, integrating features like health monitoring helps businesses to track and analyze health-related data, such as heart rate, physical activity, and sleep patterns. This paves the way for better insights and alerts, helping users identify health issues early before they become severe and maintain their well-being, thereby improving patient care.
Visual Search
Visual search enables users to search for their desired product using images rather than text. This feature utilizes computer vision technology to detect pictures, allowing users to find products, information, or similar images quickly and accurately. It is particularly useful in the retail and eCommerce sectors, enhancing user engagement and the shopping experience.
Language Translation
Language translation translates text or speech from one language to another in real-time. This feature breaks down language barriers, facilitating smooth communication and interaction in a globalized world. This functionality is essential for international business, travel, and hospitality sectors, facilitating multilingual customer support. To make an AI app that stands out, companies must consider integrating real-time, multi-language translation features that can significantly boost global communication and market reach.
Gesture Recognition
The gesture recognition feature interprets human gestures, allowing users to interact with devices through movements. This feature enhances user experience by providing a more natural and intuitive way of interacting with technology. It is used in gaming, virtual reality, and smart home applications.
Facial Recognition
Facial recognition helps identify and verify individuals based on their facial features. This technique is widely used for security purposes, such as unlocking devices, authentication in apps, and surveillance. Industries such as finance, healthcare, and law enforcement can particularly leverage this feature to prevent data theft and fraudulent transactions.
Image and Sticker Generation
AI generates custom images and stickers based on user input or predefined templates. This feature enhances creativity and personalization in applications related to social media, design, and digital content creation.
[Also Read: How Much Does It Cost to Build an AI Art Generator App Like Imagine]
Text to Video Creation
AI converts written content into engaging video formats, incorporating visuals, animations, and voiceovers. This capability streamlines the content creation process, making it easier to produce professional-quality videos from text scripts easier with minimal effort.
Related Article: What is the Cost to Develop an AI Platform like Sora
Sentiment Analysis
Sentiment analysis is a core feature to make an AI app that determines the emotional tone behind a piece of text. This feature is valuable for understanding customer feedback, monitoring social media, and improving customer service. By analyzing sentiments, businesses can gain insights into customer emotions and tailor their marketing strategies accordingly.
Security and Compliance
Ensuring security and privacy is paramount for any application, and AI apps are no exception. Robust security measures, such as data encryption and user authentication, protect sensitive information from unauthorized access and cyber threats. Additionally, compliance with industry regulations, such as GDPR and HIPAA, helps ensure that AI apps meet legal and ethical standards while protecting user privacy.
Real-Time Data Processing
Real-time data processing allows AI apps to analyze and act on data as it is generated. This feature is critical for applications that require immediate responses, such as financial trading, fraud detection, and live customer support. It enhances the app’s responsiveness and provides up-to-date insights, improving user experience and operational efficiency.
By focusing on these must-have features, you can understand the answer to the question- how to create an AI app- that meets the highest standards of innovation, security, and usability in today’s AI-driven age.
How Much Does It Cost to Build an AI App?
There is no one-size-fits-all formula to quote the exact cost to make an AI app; it varies widely depending on various factors. The factors affecting AI app development cost include but are not limited to the project’s complexity, features, quality and quantity of data, chosen tech-stack, platform compatibility, location of the AI development company, and so on.
On average, building an AI app can range from $30,000 to $300,000 or more. However, this is a rough estimate; the final cost can increase or decrease depending on your unique project requirements.
Also Read: Hiring Offshore AI Engineers in New York – A Step-by-Step Process and Costs
Here is a table outlining the estimated timeline and cost of building an AI app, depending on the project’s complexity and features.
App Complexity | Average Timeline for AI App Development | Average Cost of AI App Creation | Examples |
---|---|---|---|
Simple AI app with basic features | 4-6 months | $30,000-$50,000 | Chatbot and virtual assistant for customer service |
Medium AI app with moderate features | 4-9 months | $50,000-$120,000 | Financial literacy app like Edfundo |
Highly complex AI app with advanced features | 9 months to 1 year or more | $120,000-$300,000 or more | Healthcare diagnostic apps with real-time communication, like YouComm |
Factors Affecting the Cost to Build an AI App
The cost to build an AI app can vary widely depending on several key factors. Understanding these elements can help businesses better plan and budget for their AI projects, ensuring they align with financial constraints and strategic goals. Here are some of the major factors affecting AI app development cost:
Complexity of Features
The more complex the AI features, the higher the development cost. Advanced functionalities like natural language processing, machine learning algorithms, or computer vision require more time and expertise to develop, thus increasing the project’s expense.
Data Management Needs
AI applications depend heavily on data. The cost of AI app creation will escalate if the app requires extensive data collection, cleaning, integration, and management. Secure handling and storage of data also add to the development expenses.
Integration with Existing Systems
Integrating AI with current business systems and software can be complex and add to the overall AI app development cost. The more legacy systems an AI app needs to interact with, the more expensive and time-consuming the integration will be.
Choice of Technology and Tools
The technologies and tools selected to build the AI app can significantly impact costs. Proprietary solutions might offer robust features but at a higher price, while open-source tools can reduce expenses but may require additional customization.
Development Team Expertise
The skill level and experience of the development team are crucial. Hiring top-tier AI developers or specialists can drive up costs to create an AI app, but their expertise can result in a more successful and efficient app.
Regulatory Compliance
AI apps must comply with strict regulations for certain industries like finance or healthcare. Ensuring compliance can involve additional layers of security and validation, thereby increasing the overall development cost.
Ongoing Maintenance and Updates
AI apps require continuous updates and maintenance to stay effective and secure. The cost of regular updates, training the AI models with new data, and maintaining system performance over time should be factored into the total cost.
Testing and Quality Assurance
Rigorous testing is essential to ensure the AI app functions correctly and meets all requirements. Comprehensive testing can add to the overall cost of building an app with AI but is critical to prevent failures after deployment.
Discuss your project idea with our AI team now
Future Trends in AI Application Development
AI app development is rapidly evolving due to changing user expectations and technological advancements. Here are some key trends shaping the future of AI applications:
AI and IoT Convergence
The fusion of AI with the Internet of Things (IoT) is creating smarter, more connected environments. This integration enhances data analysis from various devices, leading to more responsive and efficient AI systems.
Enhanced Personalization
AI is increasingly tailored to individual user preferences, providing highly personalized and relevant recommendations, making services feel more intuitive and user-centric.
Integration of Generative AI
The rise of generative AI technologies allows for the creation of custom content, including text, images, and videos, facilitating more dynamic user experiences.
Explainable AI and Responsible AI
As AI systems become more complex, there is a growing emphasis on explainable and responsible AI development. Explainable AI ensures users can trust and comprehend AI decisions, while responsible AI focuses on ethical considerations.
Increased Focus on Edge AI
Edge AI is shifting processing power from the cloud to local devices. This trend is crucial for applications requiring immediate responses, such as autonomous vehicles and smart sensors.
Autonomous AI Agents
The development of AI agents that can operate independently is advancing, leading to more sophisticated automated systems capable of handling complex tasks with minimal human intervention.
No-Code and Low-code AI App Development
Platforms allowing users to build AI apps with minimal coding are gaining traction. No-code and low-code trend empowers organizations to create and deploy AI and computer vision solutions without extensive technical expertise.
Human-AI Collaboration
With AI tools designed to enhance human capabilities rather than replacing them, humans and AI collaboration leads to more effective decision-making and creative problem-solving.
Integration of RAG in AI
Retrieval Augmented Generation (RAG) is enhancing AI’s ability to provide accurate and contextually relevant information by combining retrieval of existing data with generative capabilities. This trend improves the relevance and reliability of AI responses.
Quantum Computing in AI
Quantum computing revolutionizes AI by solving complex problems much faster than traditional computers. While still in its early stages, this AI trend is poised to advance AI capabilities in areas like optimization and simulation.
How to Build an App with AI: Navigating Challenges and Finding Solutions
The consideration to build an AI app is a rewarding endeavor. Still, it comes with challenges that need to be overcome efficiently to realize the full potential of AI applications. Here are some of the most common hurdles you need to overcome when considering how to develop an AI application.
Data Quality and Quantity
Challenge: High-quality, relevant data is the lifeblood of AI model development. Insufficient or poor-quality data can lead to inaccurate predictions, biases, and subpar performance.
Solution: Invest significant effort and time in data collection and preprocessing to ensure your dataset is comprehensive and clean.
Integration with Existing Systems
Challenge: Integrating AI applications with existing infrastructure can be complex and may cause compatibility issues.
Solution: Design your AI components with modularity, scalability, and flexibility in mind. Regularly test and validate the integrated system to address compatibility issues early.
Ethical and Privacy Concerns
Challenge: AI applications are made to handle sensitive user information and business data, which may raise data breaches, privacy, and ethical concerns.
Solution: Implement robust data security measures and comply with relevant regulations, such as GDPR and CCPA, to protect user privacy and sensitive data.
Also Read: Explainable AI benefits every AI enthusiast should know
Skill Gap and Talent Acquisition
Challenge: AI app creation requires a specific set of highly specialized skills and currently in short supply. Finding and retaining the right talent can be challenging and expensive.
Solution: Partnering with a dedicated app development firm with expertise in AI apps can help bridge this skill gap. Such firms bring seasoned professionals who can deliver high-quality AI solutions efficiently, allowing your business to leverage advanced AI capabilities without the overhead of recruiting and training specialized staff.
Cost of Implementation
Challenge: The initial cost of AI application development and deployment can be prohibitively high, especially for startups and small businesses.
Solution: Consider cloud-based AI services that offer scalable pricing models. Applying for grants and innovation credits can also help manage costs.
Managing Expectations
Challenge: There is often a mismatch between stakeholder expectations and the practical outcomes AI can deliver, especially in the early stages of implementation.
Solution: Set realistic goals for AI projects and keep stakeholders informed throughout the development process. Pilot projects can help demonstrate tangible benefits and refine objectives before full-scale deployment.
Algorithmic Bias and Fairness
Challenge: AI systems can easily become biased based on the data they are trained on, leading to unfair outcomes for certain user groups.
Solution: Use diverse datasets to train AI models and minimize bias. Implementing AI ethics guidelines and regularly auditing AI systems for bias can also ensure fairness in AI operations.
Scaling AI Solutions
Challenge: As businesses grow, scaling AI applications to meet increased demands without losing performance can be difficult.
Solution: Design AI systems with scalability in mind from the very beginning. Utilize cloud infrastructures that dynamically adjust resources based on the app’s needs.
Technology Obsolescence
Challenge: The rapid pace of technological advancement in AI can quickly render systems obsolete, posing a challenge for long-term investment in AI technologies.
Solution: Use a flexible development approach that allows easy updates and integration of new AI technologies. Stay ahead of AI trends and developments to anticipate and prepare for technological shifts.
Now that we know how to use AI to build an app and ensure its future growth, let’s uncover the various applications of AI, transforming businesses across industries.
Industry-Wise Use Cases of an AI Application
The applications of AI in businesses are innumerable and invaluable. Whatever you imagine, AI can achieve it. Here are some compelling applications and examples of AI across various industries, showcasing how this cutting-edge technology is driving efficiency and fostering innovation.
Healthcare — Predictive Analytics for Patient Care
AI applications in healthcare are revolutionizing patient care by enabling predictive analytics that forecast patient outcomes and improve care management. These systems analyze vast amounts of data to predict disease progression and suggest personalized treatment plans. Additionally, AI-powered diagnostic tools analyze medical images to detect diseases like cancer at an early stage.
Example: Health at Scale uses predictive analytics to enhance patient care by forecasting potential health issues before they arise. It helps healthcare providers proactively manage chronic conditions and tailor preventive care strategies, resulting in more personalized and effective treatment plans.
Transportation — Autonomous Vehicles
AI is at the core of developing autonomous vehicles, enabling them to navigate and drive safely on the road without human intervention. These self-driving vehicles use computer vision, machine learning, automotive analytics, and sensor data to make decisions and operate efficiently.
Example: Waymo uses AI in its range of self-driving cars to interpret sensor data, recognize objects, and make driving decisions, demonstrating the potential of AI in transforming transportation.
Also Read: AI in Transportation: Benefits, Use Cases, and Examples
Real Estate — Property Valuation and Market Analysis
AI enhances real estate by providing accurate property valuations and market analysis, helping buyers, sellers, and real estate professionals make informed decisions. AI apps analyze data from multiple sources, including historical sales, property features, and market trends, to estimate the right property price and forecast real estate trends.
Example: HouseEazy, a real estate platform built by Appinventiv, leverages AI to offer precise property valuations and market insights. By analyzing vast amounts of real estate data, including property attributes and market dynamics, HouseEazy provides users with accurate home valuations and investment recommendations, helping them make informed decisions.
Retail — Personalized Shopping Experiences
AI in the retail and eCommerce industry uses AI to create personalized shopping experiences for customers. AI in eCommerce apps like Target, Walmart, Amazon, etc. analyzes users’ browsing behavior, purchase history, and preferences to recommend products tailored to their tastes.
Example: Amazon’s recommendation engine uses AI to suggest products based on users’ browsing and purchase history, increasing customer engagement and driving sales.
Finance — Budget Management
AI in finance plays a pivotal role in offering personalized financial advice and detecting fraudulent activities by analyzing transaction patterns and identifying anomalies in real-time. Additionally, AI-powered applications help transform budget management by automating tasks such as budgeting, expense tracking, and investment analysis, helping users make informed decisions.
Example: Mudra partnered with Appinventiv to build a budget management app that uses AI to help millennials manage their finances efficiently. By analyzing spending patterns and financial behavior, Mudra keeps tabs on the users’ expenses and provides personalized budgeting advice, helping users set savings goals.
Manufacturing — Predictive Maintenance
AI in manufacturing uses predictive maintenance to anticipate equipment failures and schedule timely repairs. This is one of the most integral use cases of an AI app in manufacturing that helps minimize downtime and maintenance costs.
Example: General Electric (GE) uses AI in its Predix platform to predict when industrial machinery needs maintenance. By analyzing sensor data and operational metrics, Predix helps manufacturers maintain equipment efficiency and avoid costly breakdowns.
The range of roles AI applications can play in business is vast and continually expanding. In simple terms, anything a human can do, AI apps can do better and faster—and in many cases, they already surpass human capabilities.
How can Appinventiv Help You Build an AI App That Makes an Impact?
When evaluating how to build an app with AI or how to integrate AI into an app, partnering with a seasoned AI app development company like Appinventiv can make all the difference. We possess an in-depth understanding of emerging technologies and an unwavering commitment to innovation. From initial planning to deployment and beyond, our team of 1600+ tech evangelists brings a wealth of knowledge and expertise to the table, ensuring your AI app not only meets but exceeds industry standards.
We combine technical prowess with strategic insight, ensuring that every aspect of your AI app is tailored to align with your specific business goals. Whether you are aiming to enhance customer engagement, streamline operations, or unlock new business opportunities, our custom AI services will stand by you through every step of the digital transformation journey in this AI-driven era.
Here are the examples of our some successful AI-driven projects:
- Tootle: We developed an interactive intelligent agent – Tootle, that enhances user interactions through advanced AI, improving user engagement and operational efficiency.
- JobGet: Our experts leveraged AI to build JobGet that streamline the job search process and match candidates with suitable job opportunities.
- Chat & More: This advanced AI chatbot solution provides personalized customer service and engagement.
- HouseEazy: We have also developed an AI-driven real estate app – HouseEazy, that automates property price predictions based on parameters like location, number of bedrooms, and other features.
What are you still waiting for? Partner with us now and witness transforming your AI vision into a groundbreaking reality. With Appinventiv, you don’t just build an AI app; you build a state-of-the-art solution that stands out in this digitally growing era.
FAQs
Q. What are the benefits of AI application development?
A. There are numerous benefits of AI application development, such as::
- Enhanced Automation: One of the most known benefits of AI application development is the automation of repetitive tasks and processes, reducing manual errors.
- Personalized User Experiences: AI enables tailored interactions and recommendations, adapting the products and services to individual user preferences and behaviors.
- Increased Efficiency: AI-driven applications optimize operational workflows and processes across departments, resulting in faster and more accurate outcomes.
- Improved Decision-Making: By providing data-driven insights and predictions, AI apps support more informed decision-making for businesses.
- Predictive Insights: AI analyzes market trends and patterns to predict future events, offering valuable foresight for demand forecasting and planning.
Q. How to manage privacy, security and compliance challenges in AI development?
A. Managing privacy, security, and compliance during the AI app development process involves several key practices, such as:
For Privacy Challenge
- Use data anonymization to protect personal information.
- Implement differential privacy to analyze data without compromising individual privacy.
- Apply secure multi-party computation for collaborative environments.
For Security Challenge
- Employ robust authentication and access controls.
- Conduct regular security audits to identify and address vulnerabilities.
- Incorporate adversarial training to enhance model resilience against attacks.
For Compliance Challenge
- Perform regular compliance reviews to stay updated with regulations.
- Develop and adhere to ethical AI frameworks.
- Ensure transparency and explainability in AI systems to meet regulatory requirements.
Q. What is the cost and timeline to build an AI application?
A. The cost and timeline to make an AI application varies depending on various factors including the project’s complexity, platform compatibility, chosen tech stack, data requirements, expertise of AI app development company and so on.
On average, a simple AI app with basic features typically costs between $30,000 and $50,000 and takes 4-6 months to develop, while a medium-complexity app costs around $50,000 and $120,000 and takes 4-9 months to complete. Highly complex AI applications with advanced functionalities may cost between $120,000 and $300,000 or more and require around 9 months to over a year, depending on your unique project requirements.
Get in touch with us to get a more precise estimate for the AI application development timeline.
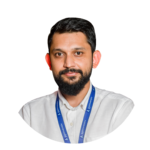
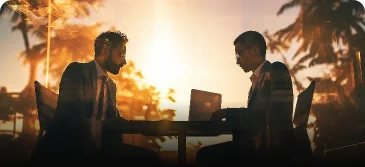
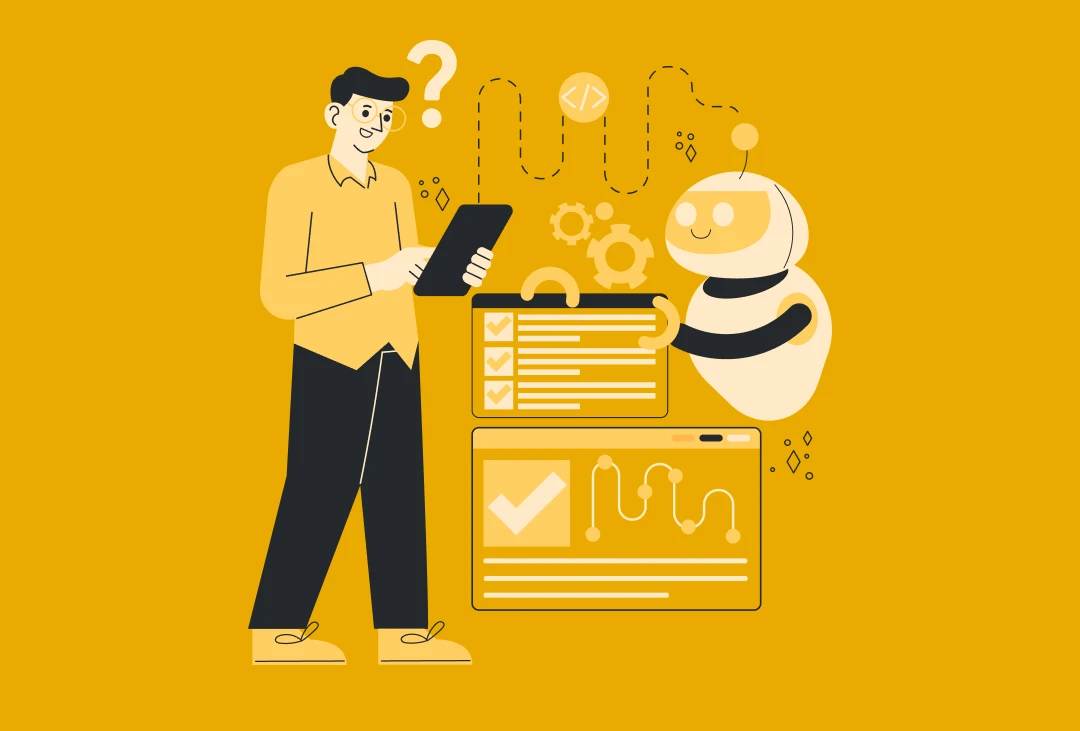
Why and How to Build an AI MVP of Your Product?
Mark had a breakthrough idea - an AI-driven hiring assistant that could predict a candidate’s success in a company based on past hiring data. The concept was solid, investors showed interest, and his team jumped into development. They worked tirelessly for the next 18 months: training complex models, refining algorithms, and building a polished, full-fledged…
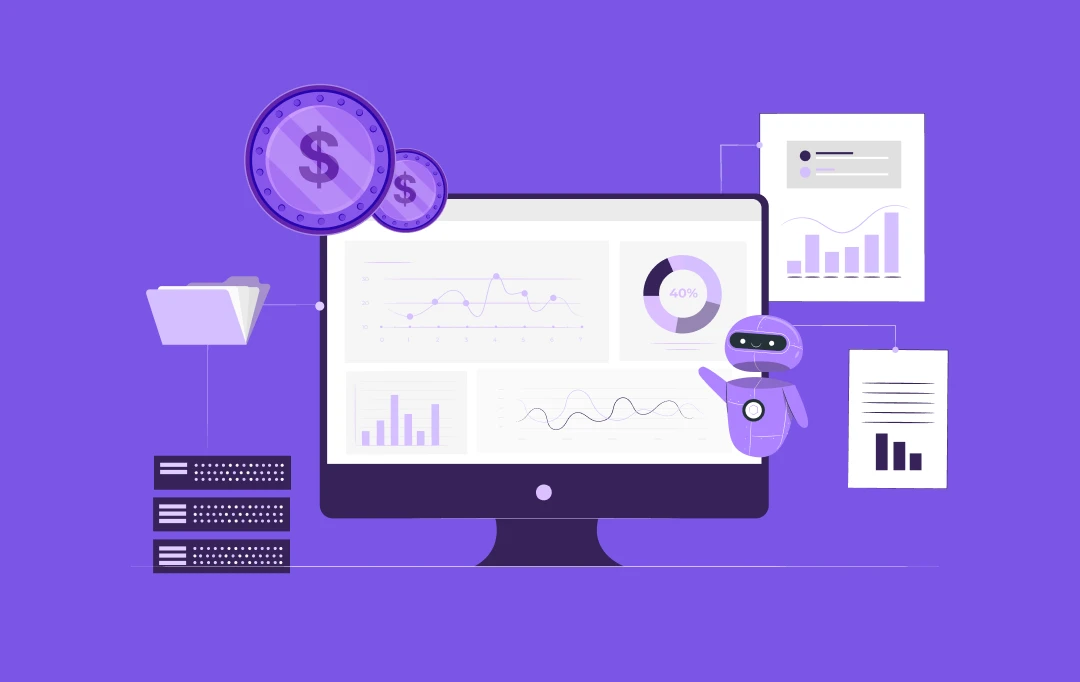
How Much Does AI Agent Software Development Cost?
AI agents are no longer a buzzword. It's a business advantage. Companies use AI agents to automate customer support, streamline operations, and make smarter real-time decisions. The results? Faster response times, lower costs, and improved customer experiences. But while the benefits are clear, one question often holds businesses back: How much does it cost to…
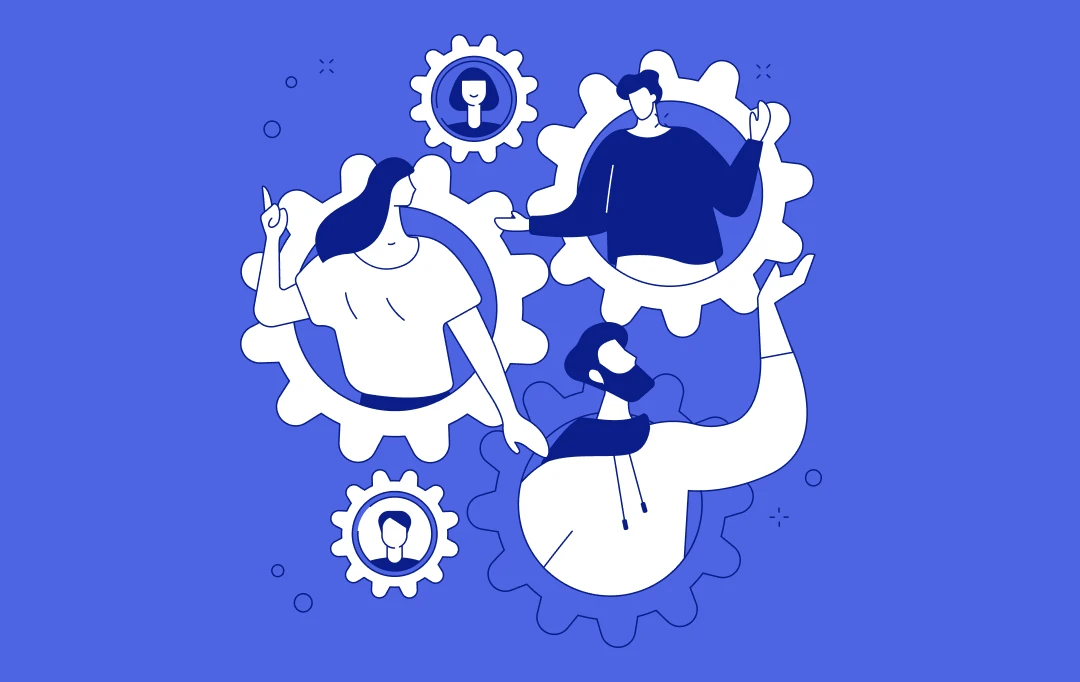
AI Integration Examples: How Leading Enterprises Are Transforming Operations with AI
Nearly 92% of companies invest in AI, but only 1% of leaders and CEOs consider themselves mature in deploying AI in their operations. This is a huge gap—who is responsible? Leaders and CEOs still treat AI as an experimental project. For years, 92% of businesses that are using AI have always associated the adoption of…