- How Is Data Analytics Used in Banking?
- Cross-Team Execution: How Analytics Powers Everyday Banking Tasks
- How Banks Use Different Types of Data Analytics
- How Banks Use Analytics Frameworks to Make Smarter Decisions
- Functional Applications of Big Data Analytics in Banking
- Risk Management
- Fraud Detection
- Loan Approval & Credit Scoring
- Personalized Marketing
- Customer Retention
- Compliance
- Customer Segmentation
- Industry-Specific Use Cases of Data Analytics in the Banking Industry
- Data Analytics in Retail Banking
- Data Analytics in Investment Banking
- Data Analytics in Digital Banking
- How Appinventiv Helped a Leading Global Bank with a Custom AI-Powered Data Analytics Solution
- Major Challenges of Data Analytics in the Banking Industry
- Strategic Integration of Data Analytics for Smarter Decision-Making
- Cloud-Based Models
- Embedded AI
- Real-Time Dashboards
- API Integrations
- How Appinventiv Helps Banks Harness Analytics to Innovate, Scale, and Thrive
- FAQs
The use of data analytics in banking is transforming how this data is interpreted and applied. Banks are using this data to make decisions that matter—fraud checks, loan approvals, or marketing campaigns.
The focus has shifted: from storing data to using it smartly, and from pushing products to understanding people—being customer-centric.
This shift is also reflected in the market. In 2024, the global data analytics in banking industry was valued at $11.55 billion. It’s projected to reach $39.16 billion by 2032 (Market Research Future).
But how exactly are banks applying these capabilities? And what does integrating data analytics in the banking sector look like in day-to-day operations?
Real-time analytics helps cut delays, reduce risk, and improve customer outcomes.
How Is Data Analytics Used in Banking?
First, let’s take a strategic look at what data analytics is in practice. How is data analytics in banking really used?
Modern banks apply structured analytics to turn everyday data—from account usage to external signals—into actionable insights across teams.
This includes internal data like account activity, support logs, or payment behaviour. It also brings in external signals—location patterns, credit history, and even social media feedback. What matters is how everything connects to build a 360-degree view of the customer and the business.
Here’s what that shift looks like:
Old Setup | Modern Banking Analytics |
---|---|
Siloed reporting by department | Unified insights across teams |
Data pulled monthly or weekly | Real-time, always-on analytics |
Limited to internal data | Blended with third-party and behavioral data |
Used mainly for reporting | Embedded into decisions across the business |
This shift is part of a larger trend—one where digital transformation in banking is reshaping how data, decisions, and customer expectations align.
What Are the Major Benefits of Data Analytics in the Banking Sector?
- Faster credit decisions using live income and spending insights
- Early fraud detection by spotting unusual activity in real time
- Higher product engagement through personalized offers
- Stronger compliance with automated KYC/AML tracking
- Better retention by identifying at-risk customers sooner
Cross-Team Execution: How Analytics Powers Everyday Banking Tasks
Analytics is built into how teams work. Risk teams cut delinquencies, product heads improve ROI, and marketing teams run sharper campaigns. The impact is real, measurable, and baked into everyday execution.
Here’s how different banking roles are using data to drive results:
Role | What They Use | What It Solves |
---|---|---|
Risk Manager | Scoring models, early-warning dashboards | Catches risk signals early—like sudden spending changes or regional default spikes—before they become losses. |
Product Head | Usage trends, lifetime value metrics | Reveals which products bring returns, which users drive growth, and where to double down. |
Compliance Lead | KYC alerts, AML monitoring, audit automation | Keeps checks running in real time and logs every step—so nothing falls through the cracks. |
Marketing Team | Segmentation data, churn triggers, response insights | Helps focus spend on the right users with offers they’re more likely to act on. |
CTO/CIO | Tech performance dashboards, integration tracking | Highlights system bottlenecks, gaps in analytics coverage, and where to streamline infrastructure. |
How Banks Use Different Types of Data Analytics
There’s no single way banks use data. It depends on the strategic objectives being pursued. That’s why data-led banking usually falls into a few clear types—each with a specific role in how decisions get made across departments.
Type | What It Does | How Banks Use It |
---|---|---|
Descriptive Analytics | Looks at what already happened | Tracks transaction trends, branch performance, or churn over time—common in data analytics in retail banking and data analytics use cases in banking. |
Diagnostic Analytics | Explains why something happened | Used across the data-led banking sector to understand service drop-offs or product issues. |
Predictive Analytics | Identifies what’s likely to happen next | Powers big data analytics in banking to flag fraud, predict loan defaults, or assess credit risk. |
Prescriptive Analytics | Recommends what action to take based on patterns | Applied in data analytics for banking to support decisions in pricing, loan approvals, and campaign planning. |
These types of banking data analytics aren’t used in isolation. Most banks combine them based on the situation. That’s where the real benefits of data analytics in banking become evident—especially in dynamic areas like digital or investment banking.
As for the features of data analytics in banking industry, here’s what modern banking analytics platforms typically include:
- Real-time dashboards for immediate decision support
- Integration with internal systems like CRM and core banking
- Access to structured and unstructured data from multiple sources
- Automated reporting to meet compliance standards
- Role-based insights for product, risk, operations, and marketing teams
So how are banks using this in real scenarios?
That’s what we’ll cover in the next section. But before that, it’s important to understand how banks leverage analytics frameworks to transform raw data into actionable insights.
How Banks Use Analytics Frameworks to Make Smarter Decisions
Raw data holds little value without the structure to operationalize it. That’s why banks turn to proven analytics frameworks that bring order to large datasets and give teams a clear direction—across credit, risk, marketing, and beyond.
These structured systems help banks move faster—with precision—across risk, marketing, and product decisions.
Framework | What It Helps With | Examples of Banking Analytics |
---|---|---|
360-Degree Customer View | Personalization & cross-sell | Uses banking CRM, app usage, and transaction history to recommend the right product. |
Next Best Offer (NBO) | Campaign targeting | Identifies the most relevant offer based on spending patterns and engagement. |
Behavioral Segmentation | Customer retention | Clusters users by habits to predict churn or upsell opportunities. |
Propensity Models | Risk scoring & upsell | Predicts the chance of product adoption, repayment, or default. |
Functional Applications of Big Data Analytics in Banking
The use of data analytics in banking is most visible in day-to-day tasks. A sudden spike in loan defaults. Unusual login activity. A drop in card usage. Analytics helps teams catch these changes early.
Here’s how data analytics plays out across banking functions:
The infographic shows how different banking functions—like risk management, fraud detection, and marketing—use various types of banking data analytics (descriptive, diagnostic, predictive, prescriptive).
Risk Management
Let’s say a customer’s payment pattern changes. Or loan defaults rise in a region. Risk teams catch these signals through banking analytics. They use transaction data, loan history, and even market trends to manage exposure. This is common in both retail and investment banking, where timing and accuracy matter.
Real Example – JPMorgan Chase: JPMorgan Chase uses a platform called Athena to manage risk across its trading and investment operations. The system runs about 5 billion calculations a day and completes 10,000 batch jobs daily. It helps teams track market positions, measure exposure, and act quickly when needed. Athena combines pricing, trade management, and analytics in one system to support real-time decision-making.
The bank also uses machine learning within Athena to improve how it predicts and handles risk. These models study past data and market trends to flag potential issues early. This allows JPMorgan to adjust faster and limit risk more effectively.
Fraud Detection
If someone logs in from a new device and transfers money out of pattern—it gets flagged. That’s big data analytics at work. One global bank used AI-driven models to improve fraud detection. The result? ATM service levels went up by 92%. That’s how fast systems can react when they’re set up right.
Real Example – Yapı Kredi Bank: Yapı Kredi uses AI to scan over 40 million transactions every day. Its fraud detection system flags around 500 suspicious activities in real time, with a response speed of just 4 milliseconds. The bank built over 1,000 detection rules and trained custom machine learning models to catch fraud early—even across new channels like crypto and open banking.
In seven years, Yapı Kredi reduced fraud losses by 98.7% and cut false positives from 200:1 to 10:1. It now holds one of Turkey’s lowest card fraud ratios—50% lower than the industry average. In 2025, Yapı Kredi won the FICO® Decision Award for its results.
Loan Approval & Credit Scoring
Approvals no longer rely on credit scores. With data-led banking and financial services, banks look at income flow, spending habits, and employment status. These models help teams decide faster and with fewer delays.
Real Example – Visa/Uplinq Partnership: Visa worked with Uplinq to help banks approve more small business loans using AI. The system looked beyond credit scores. It analyzed income trends, local market data, and customer behavior. This gave lenders a fuller view of each applicant and helped them make faster decisions.
Banks cut underwriting costs by 50% and reduced credit losses 15 times. Profitability in small business lending tripled. With Uplinq’s system, banks approved more loans without adding risk.
Personalized Marketing
Ever wondered how a bank knows when to offer a loan or new card? That’s banking data analytics doing the work behind the scenes. Banks track product usage, spending habits, and channel preferences to group users by behavior. These segments help teams deliver offers that are timely, relevant, and more likely to convert.
Real Example – JPMorgan Chase: In 2024, JPMorgan Chase launched Chase Media Solutions. This new platform helps brands reach 80 million US customers through personalized, cashback offers. It uses Chase’s own transaction data to target users based on how they spend.
During the pilot, Chase ran 30-day campaigns for brands like Air Canada and Whataburger. These campaigns aimed to boost sales and bring in new customers. Air Canada saw strong results. Their team said the targeted offer from Chase worked better than expected. It increased both awareness and revenue. The campaign showed how smart targeting can make a real impact.
Customer Retention
If a customer logs in less, stops using their card, or pulls money from accounts, that’s a sign. Data analytics for banking tracks these changes.
Real Example – Equity Bank Rwanda: Equity Bank Rwanda focused on three things—service quality, pricing, and product variety. The bank trained staff to respond quickly to complaints. It followed up to make sure issues were resolved. Mobile tools like EazzyPay helped customers bank faster and more easily.
A 2021 study showed strong results. These retention strategies explained 65.3% of the change in customer loyalty. More customers stayed because the service met their needs. The bank turned better service into higher satisfaction and steady growth.
Compliance
Regulations keep shifting, and banks have to keep up. With data analytics in the banking sector, teams automate KYC, monitor AML, and generate audit-ready reports. Analytics also strengthens cybersecurity by tracking anomalies and building fraud prevention layers.
Real Example – MUFG Bank: MUFG Bank adopted Databricks to unify its AI model development and strengthen internal compliance systems. The platform helps 30,000 employees access and analyze data faster. It also supports fraud detection, AI-driven investigations, and risk monitoring across business units.
Databricks’ governance tools help MUFG meet strict compliance requirements. The platform supports secure data handling, real-time analytics, and automated tracking—which allows the bank to improve control while reducing delays in reporting and audits.
Customer Segmentation
Some customers use cards daily, while others prefer net banking. Some respond to emails, and others ignore them. Banks use analytics to group users based on actual behavior—how they spend, where they log in, and what products they use. These segments help teams target the right users with the right actions.
Real Example – ABN AMRO: ABN AMRO built a 360-degree view of customer behavior to improve targeting and engagement. The bank tracks how users interact with tools like mobile banking and the Tikkie app. Based on these patterns—login frequency, spending habits, and response to offers—customers are grouped into actionable segments. These insights help teams deliver more relevant messages and services.
In 2024, ABN AMRO launched new AI features like a chatbot for Tikkie and a voicebot for credit card support. These tools addressed common user needs and made digital support more accessible. The bank also doubled its “Help with Banking” advisors to guide customers who prefer human support. These changes improved the overall digital experience and boosted satisfaction scores.
As a result, ABN AMRO saw strong business growth. It crossed 10 million active users on Tikkie and increased fee income by over 7%. Many businesses started using Tikkie for invoicing, strengthening the bank’s position in digital payments.
If your analytics strategy is slowing you down, we can empower you to build integrated, real-time systems that deliver actionable insights—fast and at scale
Industry-Specific Use Cases of Data Analytics in the Banking Industry
The use of big data analytics in banking shifts depending on the focus—retail, investment, or digital—and each brings its own priorities and data needs.
Here’s how banking analytics looks in action across these domains:
Data Analytics in Retail Banking
Retail banks deal with scale—millions of accounts, high transaction volume, and a need to stay relevant to individual customers.
- Customers say tailored experiences affect their banking loyalty (Accenture, 2025)
- Data analytics in retail banking is used to segment users by behavior, detect churn early, and deliver timely product offers
- Declining logins, reduced usage, or missed payments are all tracked to prompt re-engagement
Data Analytics in Investment Banking
Timing and precision matter more than volume here. Most decisions are driven by predictive models that run on deep data.
- Data analytics in investment banking is used for trade simulations, risk scoring, and market modeling
- Banks pull from real-time feeds, internal research, and external financial indicators to run what-if scenarios
- Investment banks using generative AI could boost front-office productivity by 27–35% by 2026. (Deloitte)
Data Analytics in Digital Banking
This is where real-time decisions meet everyday user behavior
- More than 74% of banking activity now happens on mobile (Statista, 2023)
- Data analytics in digital banking tracks taps, swipes, and drop-offs to guide credit offers, trigger fraud alerts, and shape product flows
- Behavioral data feeds into dynamic pricing, smart onboarding, and customer support routing
How Appinventiv Helped a Leading Global Bank with a Custom AI-Powered Data Analytics Solution
A leading European bank partnered with Appinventiv to build a custom AI platform that could improve customer retention, automate support, and optimize ATM operations. The solution was delivered in 10 weeks and integrated into the bank’s daily systems.
Key functional outcomes:
- 20% increase in customer retention
Appinventiv built a churn prediction model that ranked home loan customers by risk and gave reasons behind the scores. This helped teams intervene early and reduce customer loss. - 92% improvement in ATM uptime
Using 10 million+ data points, the platform forecasted cash needs for each ATM, helping the bank restock machines efficiently and avoid downtime. - 20% drop in customer support costs
A multilingual chatbot was deployed to handle over half of routine service requests—freeing up staff for more complex issues. - Faster service recovery
AI models monitored performance in real time and flagged system issues before they affected customers.
This project shows how banks can apply FinTech data analytics to improve operations at scale.
Major Challenges of Data Analytics in the Banking Industry
Even the most data-focused banks run into roadblocks. Some are technical. Others are structural. Here’s what teams often deal with—and what’s working to move past it.
Challenges of Data Analytics in Banking Industry | What’s Really Happening | What’s Working |
---|---|---|
Legacy systems | Core platforms are too old to support modern analytics | Add cloud-native layers that pull data without disrupting the core |
Data silos | Teams store info in different systems, making it hard to connect the dots | Use APIs or build centralized data hubs so insights are shared—not scattered |
Regulatory complexity | Teams are unsure what’s allowed—especially with privacy laws and cross-border data | Set up compliance-first models with clear tracking, consent, and anonymization |
Talent gaps | Banks don’t always have enough internal analytics skills to scale what they’ve started | Upskill internal teams and partner with banking analytics companies that |
Vendor lock-in | Hidden vendor lock-ins and legacy tech often inflate the cost of data analytics in banking. Explore banking app development costs to plan budgets better. | Use open frameworks and build models that can move across platforms if needed |
These are the most common challenges of data analytics in the banking industry—but none are deal-breakers. With the right approach, they’re solvable.
For banks without internal analytics teams, outsourcing is a strong option. See how fintech firms are outsourcing analytics effectively.
See how Appinventiv can help your bank overcome analytics friction at scale
Strategic Integration of Data Analytics for Smarter Decision-Making
It’s one thing to collect data. The real shift happens when teams start using it in real time—across risk, lending, service, and product.
That’s where data analytics in the banking industry moves from reporting to decision-making. Explore how AI is transforming core banking operations.
Banks are investing in infrastructure that allows insight to flow—fast, clean, and across departments. Here’s how it’s coming together:
Cloud-Based Models
Banks need scale. Cloud platforms give them room to process large volumes of data without delays.
Instead of building everything in-house, teams now plug into cloud environments that handle storage, computing, and analytics in one place. It’s quicker to launch, easier to scale, and more efficient for everyday use.
Embedded AI
AI has moved from isolated pilots into daily workflows.
It now scores loan applications, flags fraud patterns, and recommends next steps for service or product teams. These aren’t separate tools—they sit inside banking systems, built to support decisions in the moment.
Real-Time Dashboards
Dashboards have evolved. They’re no longer about monthly summaries. Teams track activity as it happens.
A branch lead watches transaction spikes. A credit team monitors early signs of default. A marketing lead sees how a campaign is performing right now.
It brings everyone closer to what’s actually happening—without waiting for reports.
API Integrations
Successful adoption depends on seamless interoperability between systems. APIs are helping banks pull data from multiple systems—CRM, mobile apps, fraud engines—and push it into one analytics layer.
That’s how integrating data analytics in banking sector starts to feel seamless.
How Appinventiv Helps Banks Harness Analytics to Innovate, Scale, and Thrive
Banks are done waiting on scattered reports and siloed insights. They want systems that give answers when decisions are being made.
Appinventiv helps banking teams connect the dots—across legacy infrastructure, product systems, and customer data.
500+ legacy systems modernized
Banks often start with older tech that can’t scale. We help upgrade these systems to cloud-first models that support real-time analytics without slowing teams down.
3000+ solutions delivered across industries
We’ve delivered solutions that address core operational challenges. Our data science and analytics services are created to fit real banking use cases.
1600+ data and tech experts
Our cross-functional teams bring analytics, engineering, and product together—so banks don’t have to manage 10 vendors to get one system working.
35+ industries mastered
We work across banking, finance, and investment workflows. Our banking software development services are built to meet compliance, speed, and integration needs.
If your banking team is sitting on more data than decisions, it’s time to talk
FAQs
Q. What tools support big data analytics in the banking industry?
A. Banks use tools like Tableau, Power BI, and cloud-based platforms to process and visualize data. These tools help teams track patterns and make faster, smarter decisions. Many banks also build custom analytics platforms tailored to their systems, workflows, and compliance requirements.
Q. How does big data analytics in banking improve decision-making?
A. It helps identify fraud, assess credit risk, and personalize services. With access to real-time data, banks can act faster and serve customers more effectively.
Q. What should banks look for in a banking data analytics company?
A. Look for a provider with proven results, secure infrastructure, and the ability to integrate with core banking software. Custom solutions and industry experience matter, too.
Q. How does big data analytics in banking and financial services help with compliance?
A. It automates fraud detection, flags risky transactions, and generates reports for KYC and AML checks. This makes compliance faster, easier, and more accurate.
Q. How can mid-sized banks get started with data analytics?
A. They can start with cloud-based analytics tools, connect them to core systems, and track key business metrics. A phased approach makes it easier to scale as they grow.
Q. What are the key benefits of data analytics in banking?
A. It helps banks make quicker decisions, lower risk, improve customer targeting, and stay compliant. Teams get clear insights to act faster and deliver better results.
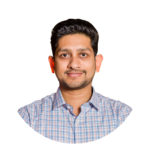
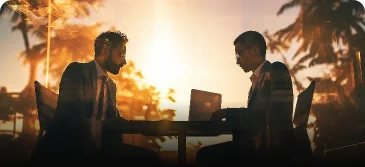
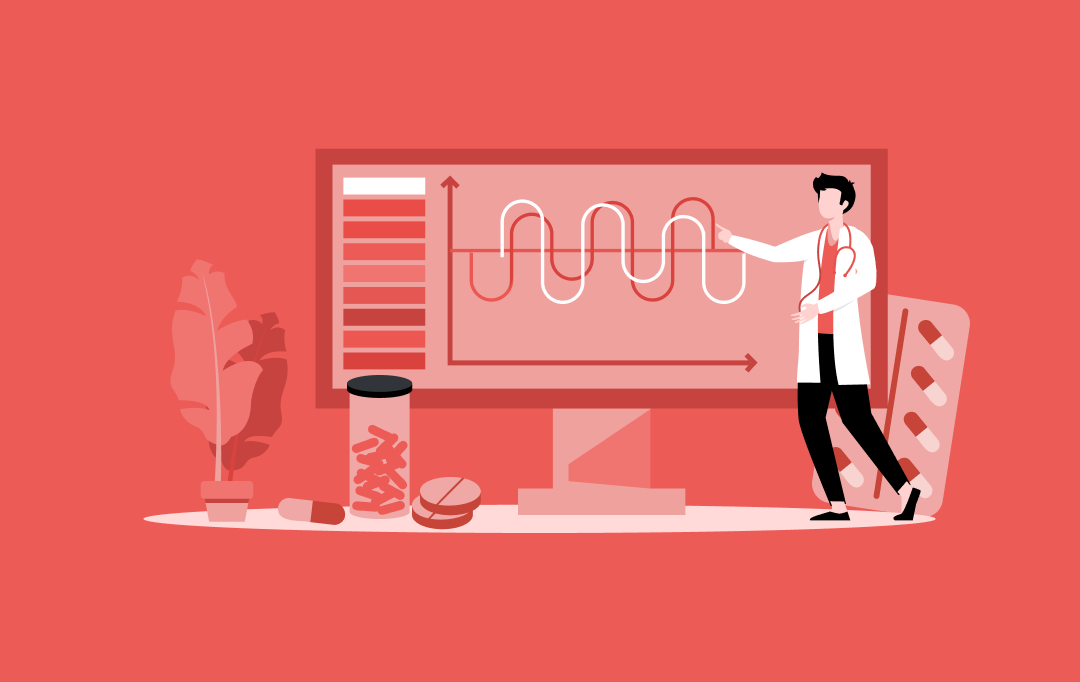
10 Use Cases and Examples of How Pharmacy Data Analytics is Transforming Operations and CX
Data has become the currency of modern business, and the pharmacy sector is no exception. From inventory optimization to regulatory compliance, pharmacies today face many challenges that demand precision and agility. Well, pharma data analytics is solving all these conundrums as the main lead, a transformative approach that now empowers businesses to get actionable insights…
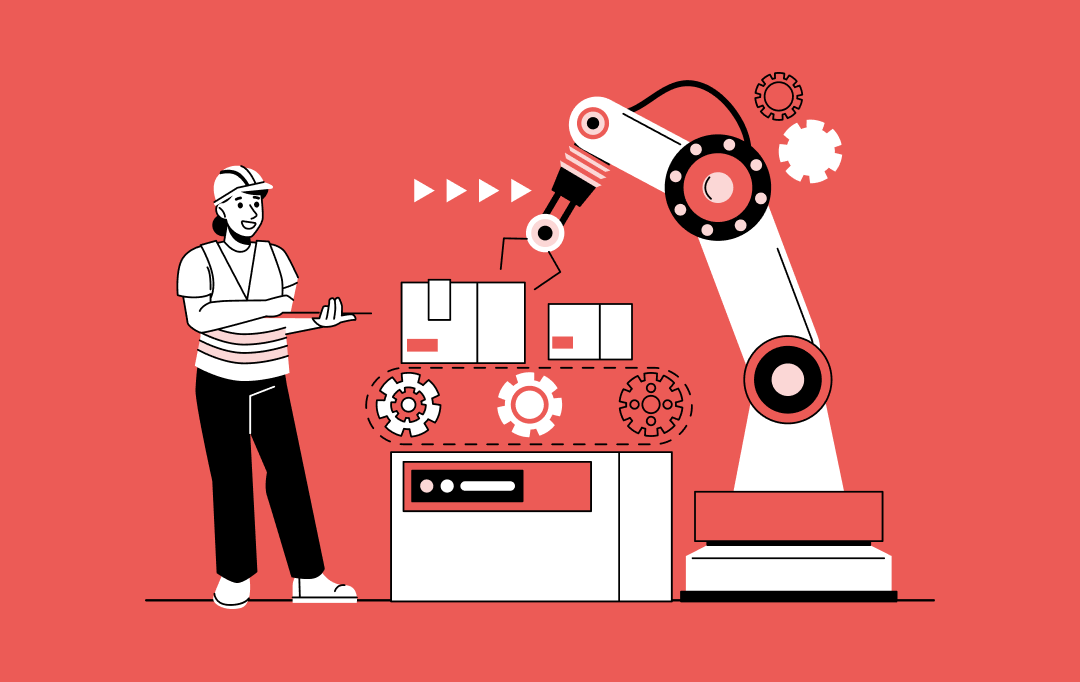
10 Use Cases and Benefits of Predictive Analytics in Manufacturing
Are you trying to stay in today’s manufacturing game with that old-school data analytics approach? Not gonna happen, buddy! Think about that old-school grind: manufacturing plant techies had to hustle around, scribbling notes and logging maintenance records by hand. All that manual intervention and hassle led to nothing more than messy data and bad calls.…
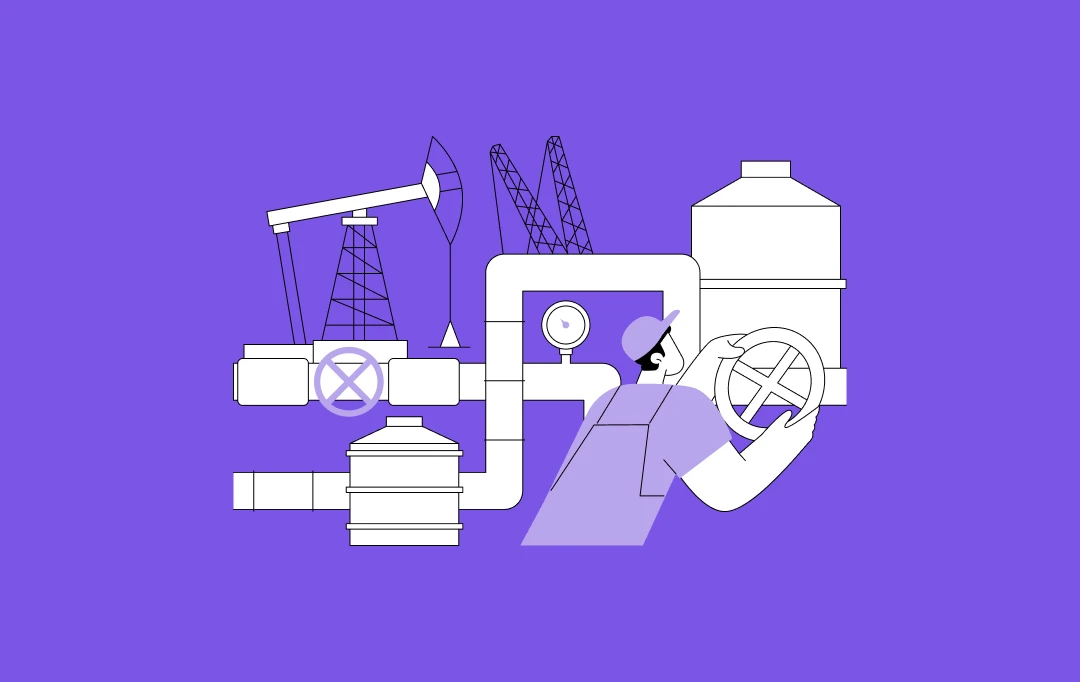
10 Use Cases and Benefits of Adopting Predictive Analytics in the Oil and Gas Industry
The oil and gas industry has always been at the forefront of technological advancements, constantly seeking innovative solutions to improve efficiency and maximize output. It is where predictive analytics emerges as a game-changing solution, transforming how companies manage operations, optimize production, and mitigate risks. In the oil and gas sector, where even the smallest oversight…