- What is Predictive Analytics in Manufacturing? The Maturity of Conventional Analytics
- 10 Use Cases of Predictive Analytics in Manufacturing
- Demand Forecasting
- Predictive Maintenance in Manufacturing
- Inventory management
- Workforce Management
- Product development strategy optimization
- Material Procurement
- Manufacturing Execution Systems
- Supply Chain Management
- Energy Optimization
- Marketing and sales optimization
- Benefits of Predictive Analytics in Manufacturing
- 1. Real-Time Monitoring & Alerts
- 2. Reduce Downtime
- 3. Cost Saving
- What are the Challenges of Predictive Analytics in Manufacturing?
- Data Quality and Availability
- High Implementation Costs
- Data Security and Privacy Concerns
- What is the Future of Predictive Analytics in the Manufacturing Industry?
- How Appinventiv’s Data Analytics Consulting Service Boosts Predictive Analytics for Manufacturers
- 1. Customized Data Processing Approach
- 2. Constant Improvement With Real-Time Monitoring & Alerts
- 3. Minimize Downtime With Predictive Maintenance
- 4. Supply Chain Optimization
- How Appinventiv Helped Build a Customer-Centric Data Analytics Platform for a US-Based Company
- FAQs
Are you trying to stay in today’s manufacturing game with that old-school data analytics approach? Not gonna happen, buddy!
Think about that old-school grind: manufacturing plant techies had to hustle around, scribbling notes and logging maintenance records by hand. All that manual intervention and hassle led to nothing more than messy data and bad calls.
How you scoop up and break down your data could be a win-win. Yes! These two are the differentiators that always put you ahead of the pack. With the proliferation of data science and AI analytics, this data analytics gamut has become faster, safer, and easier to manage.
Alright, enough talk—let’s dive into a broad spectrum – predictive analytics for manufacturing and see how it improves decision-making to augment the manufacturing game while increasing productivity and efficiency.
What is Predictive Analytics in Manufacturing? The Maturity of Conventional Analytics
Stemming from the root of advanced analytics, Predictive Analytics is the robust technique for analyzing data based on historical facts and experience to predict more distant future trends and events. The outcomes forecast potential scenarios that help manufacturers gain a crystal ball and stay ahead of the curve.
Data analytics is said to be the backbone of marketing strategy. Still, with the inception of AI, data analytics has become more advanced—predictive analytics. With their advanced functionalities, predictive analytics in the manufacturing industry have changed the game for ages, helping manufacturers make sense of data and make informed business decisions.
But here’s the kicker—why are the old-school analytics approaches suddenly losing their charm? Why are manufacturers kicking these traditional approaches to the curb?
It’s all because of one thing: predictive analytics—the brainy sibling of conventional analytics.
Conventional Analytics – Figuring out what went down and why it went sideways. Predictive Analytics – Giving the lowdown on what’s coming next. |
---|
Consequently, with the conventional analytics approach, manufacturers are usually stuck in the reactive zone and attempt to fix problems after they blow up. With the predictive analytics approach, they can stay ahead of the curve and resolve issues before they become major problems.
Say you’ve got a production line that randomly falters. With a conventional approach, you’d dig through past data to identify the usual suspects and then figure out what went sideways. But by the time you get there, you’ve already wasted a chunk of your precious time and cash goodbye.
That’s why manufacturers are doubling down on predictive analytics—to assist them in spotting trends before they blow up, dodging risks, and capitalizing on new opportunities before the competition even sees them coming.
If you’re thinking just a few manufacturers are toying with this high-tech stuff, you’re way off, buddy! Tons of companies are hopping on the predictive bandwagon. And a few of them are crushing it so well that they’re scoring killer results.
10 Use Cases of Predictive Analytics in Manufacturing
Thanks to the AI boom, the manufacturing landscape has transformatively leveled up. Manufacturing is now all about cranking out stuff smartly instead of just cluelessly pumping out a load of things. That’s why manufacturers are now deploying predictive analytics. It dishes out influential insights and smooth processes and backs up smart, data-driven decisions to make boss moves that exponentially skyrocket revenue and profits.
However, the real game-changer is predictive analytics use cases and how you implement predictive analytics in manufacturing. This makes your data crunching super sharp. There are tons of predictive analytics applications in manufacturing. Let’s dive into 7 use cases of predictive analytics in manufacturing that will take manufacturing ops to a new level. Also, dive into the real-life predictive analytics examples in manufacturing.
Demand Forecasting
Looking to have a big shot in the manufacturing game? You gotta stay ahead of the curve. Think of — knowing what your customers want before they even do. That’s exactly what predictive analytics do for you. Equipped with heavy-duty algorithms, it smartly digs into past data, spots market trends, and scoops out all external factors that impact product demand. You can nail product demand like a pro with all these spot-on predictions.
How do Data Science & Predictive Analytics Forecast Demand?
Data Science and Analytics help enhance predictive analytics of demand forecasting by building on its solid framework, using large datasets, advanced analytical techniques, and insights in real-time.
Real-Life Example: Nike
Nike’s got the whole game on lock when deploying predictive analytics to its best to forecast worldwide demand for their kicks. Besides guessing—they’re breaking it down with AI, from analyzing past sales to local tastes to fashion vibes. By reading the data like a boss, Nike streamlines production for every market, keeping ops tight and avoiding overproduction for low-demand regions.
Predictive Maintenance in Manufacturing
No more waiting around until your machine decides to bite the dust—fix it before it starts thinking about tapping out! Predictive Analytics takes all that guessing out of maintenance, enabling you to keep checking when your machine’s up ahead of time. However, you’re dodging surprise breakdowns, cutting down on repairing costs and getting the maintenance on point without last-minute scrambles.
How does Predictive Analytics Work For Predictive Maintenance?
Predictive analytics for predictive maintenance (PdM) is a boon for the manufacturing industry. By tapping into real-time data and advanced-level algorithms, you can smartly optimize the performance of your machines and spot the issues before they snowball into a big mess. However, predictive analytics sidestep downtime costs and keep your production line rolling smoothly. Here’s a brief overview of how it works:
- Data Collection via IoT Sensors: Predictive analytics in manufacturing operations is like a health tracker for your machines. Machines powered with IoT sensors monitor everything—pressure, vibrations, temperature, etc. These sensors constantly pull in data, giving you a 360-degree view of your equipment’s operation.
- Data Analysis via Machine Learning Algorithms: How you analyze your collected data is a dominant factor in spot-on predictive maintenance in manufacturing. By leveraging the machine learning algorithms, you can track patterns and faults that may indicate potential failures before they escalate. This constant evaluation gives room for timely alerts for continuous machinery improvements.
- Maintenance Scheduling: Say goodbye to the old-school maintenance method – reactive maintenance based on fixed schedules with predictive maintenance. It is advisable to schedule maintenance from time to time based on the gathered insights. This lifecycle prediction enables you to estimate the machine’s useful life and plan maintenance or replacement strategically.
Real-Life Example: Airbus
Airbus’s dedicated platform, Skywise, employs predictive analytics to monitor aircraft components and constantly forecast maintenance needs. This has helped Airbus slash unplanned maintenance by 50 percent while reducing downtime costs and improving safety.
Inventory management
Have nightmares of a warehouse full of unsold goods or running out of stock in high demand? Wake up! Thankfully, it’s a dream. When your daddy is at your back—Predictive Analytics, say goodbye to such nightmares. AI-powered analytics study all past inventory data, supply patterns, demand predictions, and supplier lead times, enabling you to strategically plan and optimize the stock level.
How Predictive Analytics Work to Streamline & Optimize Inventory Level?
Predictive analytics in manufacturing operations is like a crystal ball—it analyzes all the past and present data to spot future trends, behaviors, and even what’s going to go down next.?? And when it comes to managing inventory smartly, it’s a total game-changer.
The insights help keep your ops running smoothly while ensuring you aren’t buried in extra stock or left hanging with empty shelves. Here’s a brief overview of how predictive analytics tools for manufacturing work to optimize inventory levels.
Real-Life Example: Zara
This fast-fashion giant optimizes inventory management through predictive analytics and real-time data science. They deploy RFID tags on every item to track the stock availability in stores and from the warehouse.
Also Read: How RFID Tags Work in Healthcare Industry
This data gathered is transferred to a centralized processing center that constantly monitors inventory levels and sales patterns across the outlets. With this, Zara smartly adjusts the inventory based on demand forecasts. As a result, Zara sells over 11,000 items yearly, which is a significantly higher achievement.
Also Read: How can Data Analytics Help Improve Inventory Optimization in Retail?
Workforce Management
Amazingly, the manufacturing industry can manage its workforce smartly using predictive analytics. Like your machines, your workforce is a major asset for your business. How you handle the needs of the staff members and keep them happy can make or break your factory’s productivity. If you fail to keep up, you’ll be either shorthanded or overstaffed. This could be a major buzzkill for your profits. But predictive analytics can be your super-smart HR buddy that helps you manage staff and predict labor shortages before they hit.
How Predictive Analytics Optimize Workflow Management?
You can employ predictive models to analyze the workforce data, from shift patterns to changes in absenteeism patterns, seasonal demand, etc. The insights gathered from the analysis will help you make accurate predictions for staffing needs to avoid overstaffing and understaffing. This smooths your workflow management while cutting unnecessary labor costs.
Besides staffing, predictive analytics can help you in:
- Identifying Skill Gaps: Predictive analytics utilizes data from multiple sources, including employee performance metrics, industry trends, and feedback mechanisms. This helps you identify your team’s skills and predict future requirements to pinpoint improvement areas.
- Predicting Revenue: Predictive analytics collect insights from employee engagement data, such as employees with lower absenteeism, satisfied employees, productive employees, etc. Such insights can help you predict your revenue growth.
- Improving employee performance: Predictive Analytics provides valuable insights from data that help identify various factors that boost employees’ performance. Based on these data-driven insights, you can strategize impactful employee training and development programs and develop targeted interventions and recognitions to improve workforce productivity and efficiency.
Real-Life Example: PepsiCo
PepsiCo employs predictive analytics to control overtime requirements by projecting seasonal workforce requirements using historical sales data and other external factors, such as market trends. This allows them to avoid making too much overtime and perfectly schedule the shifts and staffing requirements, cutting off overtime costs and enhancing employee satisfaction.
As a result, PepsiCo reduced overtime costs by some 20% during peak seasons compared to previous years; employee retention improved during peak seasons.
Product development strategy optimization
In the world of predictive analytics, why need to spend millions on the unplanned development of a product that’s doomed to fail? With predictive analytics, you can easily master complex product development processes. You can get a clear picture and directions to what will and won’t work for you. It smartly captures data from various sources, such as customer trends, preferences, and feedback mechanisms.
Predictive analytics smartly analyze the captured data and collect valuable insights and components to forecast product outcomes and enhance the effectiveness of product development.
How does predictive analytics work in optimizing product development strategy?
Predictive analytics significantly helps smoothen product development strategies by turning data into actionable insights. This allows you to easily predict trends, reduce the risks associated with offering products, and obtain the most value from products by making the right decisions.
The fact is that optimizing each phase of the product development cycle, from ideation to after-launch stages, ensures that products will meet the market’s needs since they are designed to hold immense value for consumers. Here’s a brief overview of how it works:
Real-Life Example: Harley-Davidson
Harley Davidson crushed it by teaming up with an Algorithm AI model called Albert to get the inside scoop on its customers’ preferences and crank up its market game. The results were off the charts! They saw a serious hike in targeted leads, revving their New York sales. Not only this, but in just one week, they moved 15 bikes off the lot.
Material Procurement
Reactive calls, inconsistent supplies, extra stock pilling, stockouts, and sky-high ops costs are signs of chaotic procurement that disrupt production. But predictive analytics can be a game changer, providing a crystal ball to predict what, when, and how much you need. This data-driven approach fuels the smart decision-making process, enabling the identification of issues and solutions before they become major ones.
How Does Predictive Analytics Streamline Material Procurement?
- Data Collection: Data on material consumption, supplier performance, market trends, and inventory stock levels is extracted from ERP systems and IoT sensors.
- Data Cleaning and Preparation: Remove inconsistencies in data and fill missing values to ensure high quality.
- Analytics: Use historical data to explain consumption patterns, seasonal variations, and suppliers’ reliability.
- Predictive Modeling: Our advanced machine learning algorithms predict future material needs based on production schedules and market demands, giving you the confidence to plan.
- Optimization: With the optimization algorithm, develop an effective procurement plan that ensures less wastage with an economical strategy.
- Reporting and Decision Making: Create reports and dashboards that visualize procurement metrics and trends for stakeholders.
Real-Life Example: Caterpillar
Predictive analytics helped this construction equipment company to improve the procurement process. Analyzing equipment usage data and market trends can predict demand for specific parts and materials. This allows the company to negotiate better contracts with suppliers, cutting costs and ensuring timely delivery of critical components.
Manufacturing Execution Systems
MES is a critical link between enterprise resource planning and the shop floor. It is a real-time data source about production processes, equipment, and workforce for manufacturers to optimize their operations. Predictive analytics incorporated into MES improves decision-making, efficiency, and productivity by using historical and real-time data to predict future outcomes and detect potential problems before they occur.
How Predictive Analytics Helps in Manufacturing Execution Systems
Core Functionality: Predictive analytics will improve manufacturing by enabling real-time equipment monitoring, predicting failure, and proactive maintenance to avoid downtime. It enhances quality control by identifying patterns of defects for timely corrective actions. Efficient analysis of material usage leads to resource optimization, reducing costs and waste.
It also optimizes supply chain management by predicting demand trends, thus allowing producers to adjust their schedules and avoid overproduction. Lastly, predictive analytics will equip the decision-maker with what is needed to guide production planning and workforce management for optimum inventory.
Real-Life Example: Siemens
Siemens integrated predictive analytics into its MES. Using IoT sensors and analyzing historical data, the MES could predict real-time machine failure and maintenance. This innovation brought sustainability to the organization and reduced unplanned downtime while improving OEE.
Supply Chain Management
Managing the global supply chain is as complex as juggling a Rubik’s cube in a hurricane. One wrong move can snowball into a whirlwind of delayed shipments and angry customers, and a PR nightmare could hit your brand harder and put your entire reputation on the line. Thanks to predictive analytics in the supply chain, it ease these complexities and facilitates you with more resilient supply chain management.
How Predictive Analytics Smoothen Supply Chain Management?
Core Workflow: The supply chain management workflow begins at the following:
The data collection stage sources historical and live data from sales, inventory, and supplier performance.
Data processing follows, cleaning and preparing that data to spot what moves the needle in your supply chain.
Model development follows, utilizing machine learning and stats to build demand forecasting models and optimize inventory.
Finally, it’s implementation, applying all those insights to make decisions in the real-time moment of choice; it’s using all that information to keep all the wheels well-greased.
Real-Life Example: Amazon
The company employs predictive analytics to the extreme with the help of the dedicated Supply Chain Optimization Technology, or SCOT. This AI-driven technology can collect valuable insights like inventory data, order volume, traffic, and weather conditions.
With such useful insights, the team forecasts the time it will take to deliver and increases the simplicity of the supply chain. Consequently, Amazon boosted its demand forecasting by 15 times over two years, making deliveries faster and enhancing stock management.
Energy Optimization
One of the most significant cost drivers and environmental concerns is energy consumption. Manufacturers use more predictive analytics to ensure optimum energy usage, cost savings, and sustainability. Predictive analytics uses statistical algorithms and machine learning techniques to understand patterns in historical data for energy usage and predict what that will be in the future, enabling manufacturers to develop more efficient practices.
How Predictive Analytics Helps in Energy Optimization
- Demand Forecasting: Predictive models are applications that examine prior data and are, hence, precise in predicting future demand. They allow providers and users to distribute services and utilization properly.
- Waste Identification Patterns: Companies can identify areas of energy wastage by analyzing the consumption records of smart meters and IoT devices. This insight allows for implementing corrective measures, effectively reducing energy wastage.
- Maintenance Prediction: With predictive analytics, the foreseen breakdown of equipment becomes possible, which allows predictive maintenance and minimizes energy losses while optimizing performance.
- Renewable Energy Integration: It predicts weather patterns and estimates renewable energy generation, thus balancing sources such as solar and wind with energy consumption.
Real-Life Example: NextGen Invent
NextGen Invent also provides AI and predictive analytics solutions to help manufacturers address energy consumption challenges. These include automated energy audits and optimizing facility energy management systems by predicting and managing peak usage times.
These advancements streamline energy management and ensure that manufacturing operations are aligned with broader goals of reducing carbon footprints and adhering to environmental standards.
Marketing and sales optimization
Building great products is only half the battle—you’ve gotta sell ‘em like a pro. AI-enabled predictive analytics empower you to market and sell your products efficiently—targeting the right product to the right customer with the right message at the right time. Beyond boosting your sales game, predictive analytics have tons of benefits:
- Scope Out the Market & Competition: Monitor your competition and the market for anything coming your way.
- Dynamically Set Pricing: Set prices dynamically upon demand and competitive moves.
- Drop Discounts: Do deals and promos at just the right time when you need them the most.
- Craft Killer Campaigns: Build and launch campaigns in real-time, hitting just right with your target audience.
How AI-enabled Predictive Analytics Optimize Marketing & Sales?
Core Functionality: The predictive model effectively leverages customer data to analyze past purchase behavior and market trends to spot high-value prospects. You’ll get a clear picture of what drives customers, and based on this, you can easily develop laser-focused marketing strategies that augment your sales and reduce customer churn.
Real-Life Example: Honeywell
Honeywell employs predictive analytics in its B2B marketing to target customers through purchase history and engagement analysis. With these insights, they alter marketing campaigns and strategies to meet an exact customer need, increasing conversion rates by up to 15%.
Benefits of Predictive Analytics in Manufacturing
Say goodbye to the old-school reactive approach of analyzing data and making half-baked decisions. Now, it’s time to level up your analytics game. How? Switch to a data-driven analytics approach and make Predictive Analytics your go-to move. Deploying Predictive analytics in the manufacturing industry hits you with spot-on forecasts, keeps your ops running smoothly, and increases your business performance. Let’s explore some other perks predictive analytics bring to your table:
1. Real-Time Monitoring & Alerts
Predictive Analytics have completely transformed the conventional monitoring approach into a real-time monitoring method that acts as proactive surveillance. Along with the 360-degree view of your data, AI sensors create an alert about potential faults in machine performance or production inefficiency before it becomes a snowball. These spot-on insights on time can fix the anomalies and reduce unnecessary maintenance losses.
2. Reduce Downtime
An unexpected machine or equipment fault can wrench your production and affect revenue and profit goals. Thanks to predictive analytics that save you from the last-minute scramble to fix faults. AI sensors collect insights from past data and crunch numbers against current wear-and-tear patterns. However, this provides spot-on forecasting of potential faults and enables you to schedule the maintenance ahead, dodging unexpected downtime.
3. Cost Saving
Predictive analytics provide you access to laser-focused and actionable insights that help you spot potential faults before they become major problems. This allows prioritizing early maintenance before they go out of control. Subsequently, this dodges unexpected breakdowns of expensive equipment and saves a big buck by slashing the unnecessary maintenance costs of repairs and replacements.
What are the Challenges of Predictive Analytics in Manufacturing?
Implementing predictive analytics in manufacturing is unique; however, effective solutions can manage the challenges and ensure optimization, cost efficiency, and enhanced competitive ability. Here’s a look at the major three challenges and how to address them:
Data Quality and Availability
Challenge: Predictive analytics depends on a quality data stream from various sources, such as sensors, ERP, and CRM systems. Inconsistencies, missing information, or inaccuracies could lead to flawed predictions, discouraging companies from trusting the analytics output.
Solution: Establish a strong data governance framework standardizing data collection, processing, and validation. Cleanse data frequently and update it as often as possible. Data enrichment methods may also be employed to fill in the gaps. Access to IoT sensors and automation of data pipelines may also improve accuracy and availability.
High Implementation Costs
Challenge: Predictive analytics investment is rather high because of technology, infrastructure, and expertise, which discourages small manufacturers or individuals with financial instability.
Solution: Start pilot projects in critical areas, like maintenance or quality control. Small-scale pilots can justify further investments and prove the ROI. Further, manufacturers can adopt cloud-based analytics since they have flexibility in scalability and can reduce the investment in on-premises infrastructure.
Data Security and Privacy Concerns
Challenge: Predictive analytics deals with voluminous sensitive production and operational data. This may increase the risk of cyber threats, data breaches, and even potential regulatory compliance issues.
Solutions: Use firewalls, encryption, access controls, and compliance with relevant data protection regulations, such as GDPR, among others. Regularly audit security protocols. Consider integrating AI-enabled security monitoring systems to detect an attack in real-time and respond immediately.
What is the Future of Predictive Analytics in the Manufacturing Industry?
If you’re wondering about the future of predictive analytics in the manufacturing industry or looking for the trends of predictive analytics in manufacturing, here are a few predictions that will help you understand the significance of adopting predictive analytics for manufacturing.
- Grand View Research forecasts that global predictive maintenance in manufacturing will reach USD 60.13 billion by 2030, registering a CAGR of 29.5% from 2023 to 2030.
- IDC forecasts that By 2027, nearly 75% of all manufacturing data will be processed at the edge, thanks to the rise of edge computing and IoT.
- Gartner predicts the growing adoption of digital twins in manufacturing. By 2030, over 90% of large-scale manufacturers will have a fully integrated digital twin.
How Appinventiv’s Data Analytics Consulting Service Boosts Predictive Analytics for Manufacturers
Manufacturers aren’t messing around with conventional data analytics approaches anymore. In this cutthroat competition, they’re playing it smart to stay ahead of the curve and crush the competition. That’s why they count on Appinventiv as the best manufacturing software development company.
Thinking predictive analytics is rocket science? That’s where Appinventiv’s Data Analytics Consulting Service steps in to make predictive analytics for manufacturers as easy as pie.
Let’s explore how Appinventiv empowers you to leverage predictive analytics to make some serious waves:
1. Customized Data Processing Approach
Something that sets us apart at Appinventiv in the world of predictive analytics is – Our Custom-fit Approach. Our squad of data scientists digs deep into your existing data and shifts through every bit to extract actionable insights. We build customized predictive models based on insights that custom-fit your specific ops.
2. Constant Improvement With Real-Time Monitoring & Alerts
We set up real-time monitoring and alerts for your equipment and production lines. This delivers immediate updates for timely interventions, as issues can be addressed before they escalate into major problems. You also reduce unexpected downtime costs, keeping your ops running smoothly.
3. Minimize Downtime With Predictive Maintenance
Downtime is the biggest culprit in manufacturing. Every minute that the machine sits idle costs bucks. Worry not! The benefits of predictive maintenance in manufacturing are that it swings it into action. With Appinventiv’s custom predictive models, manufacturers can predict when their equipment will fail and schedule maintenance in advance.
4. Supply Chain Optimization
Our bespoke Data Analytics Consulting Services provide insights on possible supply chain bottlenecks. These insights help manufacturers anticipate disruptions, embrace the necessary changes, and always be ahead of the curve.
How Appinventiv Helped Build a Customer-Centric Data Analytics Platform for a US-Based Company
The client was a leading US-based telecom company with issues regarding data silos and inconsistencies that were about to impair their ability to deliver the right personalized experience across 90 million customers. Thus, it required a full analytics platform for optimum data quality, churn rate, and customer lifetime value.
We consolidated the cloud-based data analytics platform using a broad spectrum of Apache technologies, including Spark and Hadoop. We facilitated the client with the interactive dashboard featuring an AI-powered analytics engine, making data more accessible and enhancing customer understanding.
Results
- 100% processing of customer consumption data
- 100% availability of customer data to every department
- 85% increase in data quality and accessibility
- 26% decrease in license, hardware & maintenance cost
Let’s deploy predictive analytics in manufacturing operations and develop a future-proof approach to manufacturing operations. Contact us to see what kind of customized support Appinventiv can offer you to unlock the full potential of predictive analytics for manufacturing.
FAQs
Q. What is Predictive Analytics in Manufacturing?
A. Predictive analytics in manufacturing is the application of sophisticated data analysis techniques and machine learning models to predict future events and trends based on historical data. It enables manufacturers to predict machinery breakdowns, optimize production, forecast demand, and guarantee that everything runs smoothly, thus avoiding surprise downtime and raising overall production efficiency. Therefore, the manufacturing industry using predictive analytics has seen unprecedented business revenue and profit growth.
Q. What are some major benefits of using Predictive Analytics in manufacturing?
A. Manufacturing predictive analytics can ripe various benefits, including:
- Real-Time Monitoring & Alerts: Proactive alerts based on real-time data for timely intervention
- Reduce Downtime: Predicts machinery faults and arranges their maintenance beforehand.
- Cost Savings: Avoid costly repair works by addressing faults beforehand.
- Supply chain optimization: Improves inventory management and demand forecasting.
- Product Quality Improvement: Identifies anomalies in the production process and enforces consistency.
Q. What is Predictive Maintenance Compared to Traditional Maintenance?
A. Traditional maintenance tends to be reactive, mainly because it relies on waiting for equipment failures before service or maintenance work is triggered. However, predictive maintenance in manufacturing uses real-time data and advanced machine learning algorithms to predict when failures will occur. It then enables advanced maintenance planning, reducing unplanned downtime and direct repair costs. Predictive analytics for manufacturing is like a tracker that keeps track of the health of your equipment.
Q. What are the best predictive analytics use cases in manufacturing?
A. 10 best predictive analytics use cases in manufacturing are:
- Demand Forecasting
- Predictive Maintenance in Manufacturing
- Inventory Management
- Workforce Management
- Product Development Strategy Optimization
- Material Procurement
- Manufacturing Execution Systems
- Supply chain management
- Energy Optimization
- Marketing and Sales Optimization
Q. What are some of the top predictive analytics platforms for manufacturing?
A. One can enjoy the benefits of predictive analytics in manufacturing with some popular predictive analytics platforms like Tableau, Oracle, IBM SPSS, Rapid Miner, Power BI, and more.
Q. How is Predictive Analytics the best solution to the manufacturing supply chain?
A. Predictive analytics optimizes the supply chain by collecting and analyzing different sources of information, such as sales, inventory levels, and supplier performance. This allows the determination of precise demand predictions, identification of bottlenecks, and real-time corrections in inventory and logistics plans to achieve better efficiency and cost-cutting in the supply chain.
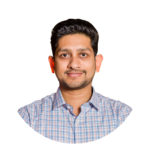
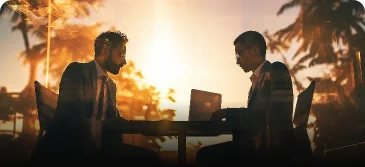
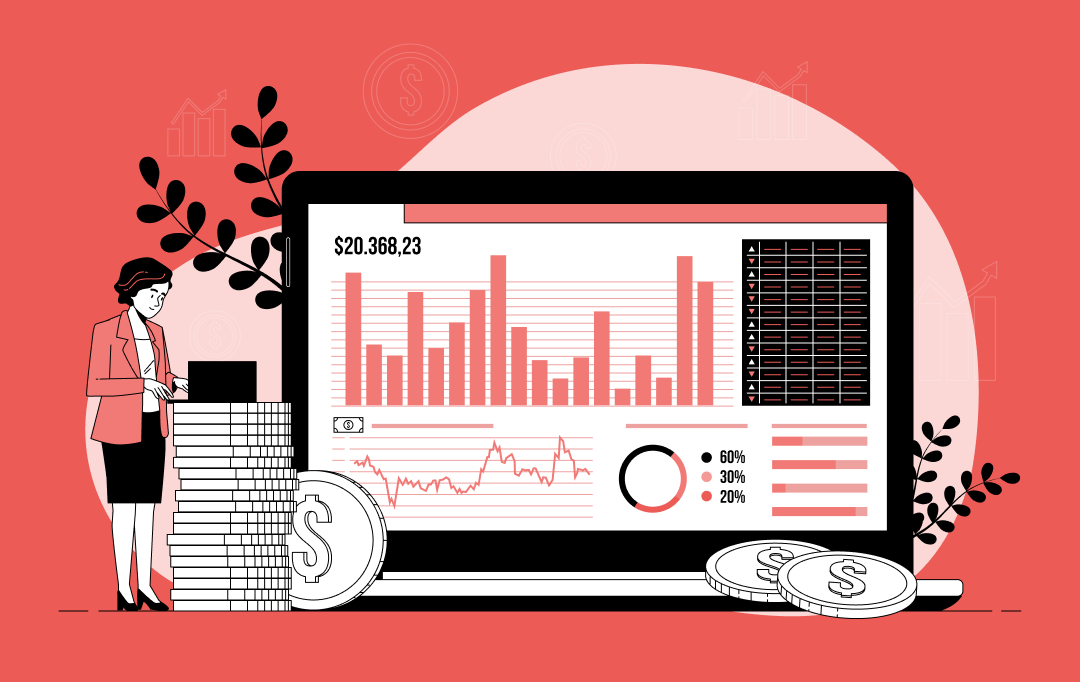
Unleashing the Role of Data Analytics in Driving Smarter Banking Decisions
The use of data analytics in banking is transforming how this data is interpreted and applied. Banks are using this data to make decisions that matter—fraud checks, loan approvals, or marketing campaigns. The focus has shifted: from storing data to using it smartly, and from pushing products to understanding people—being customer-centric. This shift is also…
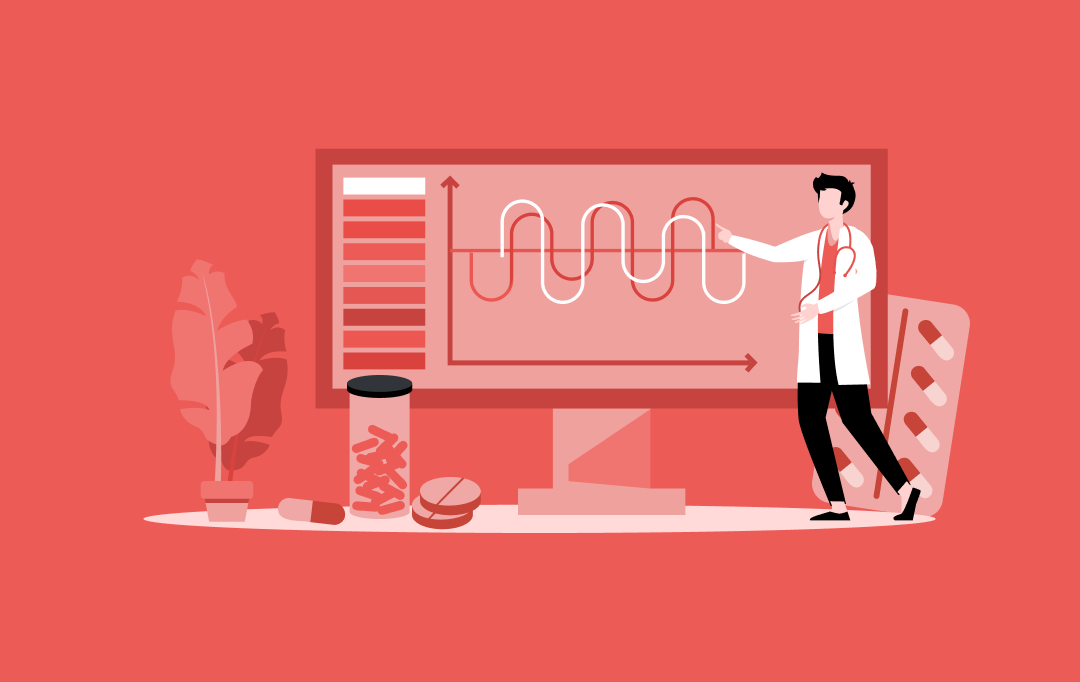
10 Use Cases and Examples of How Pharmacy Data Analytics is Transforming Operations and CX
Data has become the currency of modern business, and the pharmacy sector is no exception. From inventory optimization to regulatory compliance, pharmacies today face many challenges that demand precision and agility. Well, pharma data analytics is solving all these conundrums as the main lead, a transformative approach that now empowers businesses to get actionable insights…
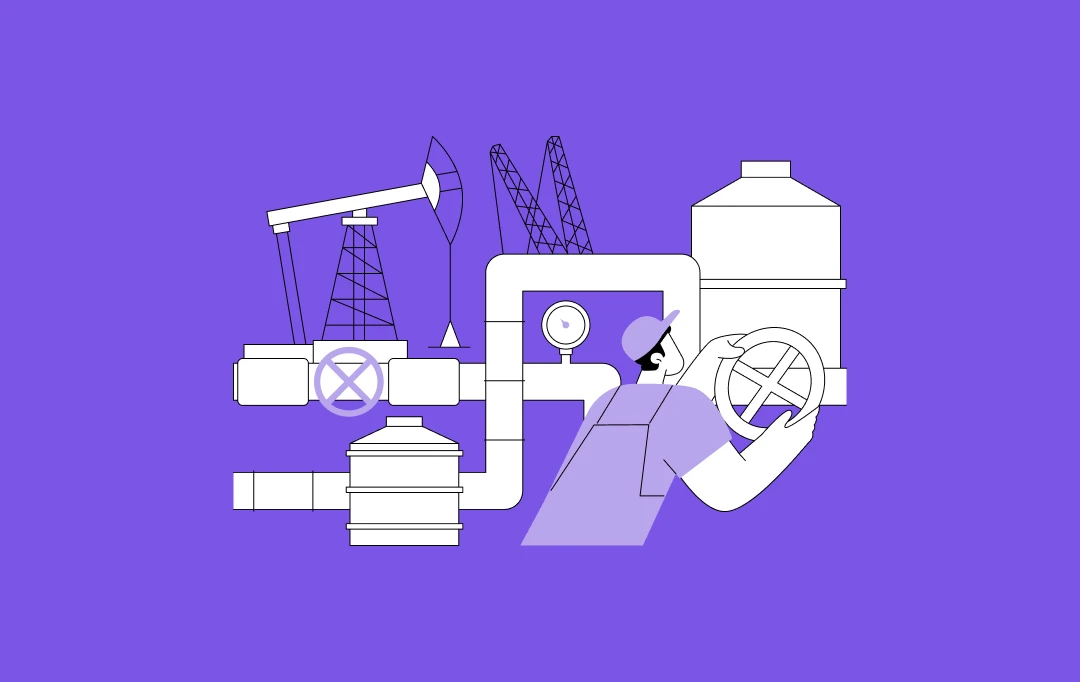
10 Use Cases and Benefits of Adopting Predictive Analytics in the Oil and Gas Industry
The oil and gas industry has always been at the forefront of technological advancements, constantly seeking innovative solutions to improve efficiency and maximize output. It is where predictive analytics emerges as a game-changing solution, transforming how companies manage operations, optimize production, and mitigate risks. In the oil and gas sector, where even the smallest oversight…