- What is big data analytics?
- Types of big data analytics
- The key elements of big data
- The advantages of big data analytics
- Understanding customers
- Finding trends
- Monitoring competition
- Better operations
- Tweaking business models
- How to get started with big data analytics in your business?
- How does big data analytics work?
- Wrapping up!
Succeeding in today’s digital era is difficult. Business leaders have to be extremely aware of their market – of the industry, their customers’ needs, and the ever-changing market disruptions and benefits. The only way to remain on top of these events is through the incorporation of big data analytics.
With the growing adoption of emerging technologies such as Machine Learning, Artificial Intelligence, and Intelligent Automation, businesses across industries have started experimenting with multiple big data analytics applications.
In a survey conducted by NewVantage Partners in late 2021, it was found that 91.7% of IT and business executives said they’re growing their big data and AI investments, while 92.1% of them reported that they are getting massive business outcomes from these technologies. Now, to be a part of these statistics, it is essential to apply the best big data analytics techniques.
In this article, we will look into the basics of getting started with big data analytics technologies in your business. However, prior to that, we would also look into what is big data analytics and why it is important for your business.
What is big data analytics?
Big data analytics is the process of finding patterns, trends, and relations between a large amount of raw data, with the intent of making data-backed business decisions. With the constant influx of data coming in from multiple touchpoints like smart devices, web usage, and customer interactions etc., big data engineers are able to use the information and convert it into key business insights.
Also read: Big Data Analytics in the Oil and Gas Industry – Benefits, Use Cases, Examples, Challenges
Types of big data analytics
Big data can be divided into three primary categories. The three types are crucial in not just understanding how big data works but also in getting started with the incorporation of the technology in the business. Here are those three types of big data analytics.
Structured data – These data sets are organized with defined dimensions according to the set parameters. These are tangible numbers which makes it easy for an architecture to sort and collect data.
Unstructured data – Any data that comes with an unfamiliar model or structure gets categorized as the unstructured dataset. It is difficult to process and then analyze this data type as it lacks specificity in structure and form.
Semi-structured data – This data type is a mix of both structured and unstructured data types. It is one that has not been classified into any specific repository but consists of important tags or information that differentiates elements within the dataset.
The key elements of big data
The technology revolves around multiple functions and components ranging from:
Big data architecture – The traditional data warehouse gets incorporated in big data architecture for storing the structured data. Moreover, businesses use data lakes that store multiple data sets in the native formats and are usually built on big data analytics technologies like Hadoop, Spark, and NoSQL databases.
Big data analytics – The big data architectures are usually brought into use for analytics applications that can vary from reporting to advanced analytics like finding anomalies. Machine learning, specifically, has been benefiting from the presence of big data for finding patterns that dictate the business chart.
Big data integration – Several integration techniques are adopted in big data for preparing the information for analysis stages like data discovery, cleansing, and validation. The element is very crucial when it comes to defining the success of a big data project.
[Also Read: What is data integration and why it matters to your business?]
Big data governance – An efficient data governance is needed for ensuring that the collection of data is consistent and complies with the data standards and privacy regulations. In order to achieve this, data quality management practices are incorporated at every individual step of big data integration.
Now that we have settled the basics of what is big data, let us look at the importance of big data analytics for your business.
The advantages of big data analytics
The importance of big data analytics stands for both established firms and startups. For the former, it helps make sense of the massive amount of datasets, for the latter, it helps through promoting agility and the ability to take quick data-centric decisions.
Understanding customers
Big data analytics applications help with giving businesses a complete picture of their customers. What makes them act, what type of products they buy and when, how they interact with businesses, and why do they choose a certain company/product over others.
Through the right set of big data analytics techniques, businesses are able to gather data from internal data, social media, public data, and browser logs – a combination that gives an inside out view into the customers’ expectations.
Finding trends
Identifying and tracking patterns and behaviors is another set of benefits of big data analytics. It tells businesses where their growth is headed, what is the demand of their product and how it is changing over time. The technology removes “instinct” from prediction about trends.
Big data tools are engineered to collate trends from social media and traditional media sets, customer behavioral patterns. It, in turn, informs businesses of where they should focus their energy on a proactive level both in terms of targeted advertisements and customer retention.
You may also want to read, The top 5 trends shaping the future of data infrastructure in 2022 and beyond
Monitoring competition
Traditionally, understanding competition moves has been limited to activities like reading business news, pretending to be a customer to get insights into processes, etc. Today, however, you get every information you need about the competition without even leaving the desk.
Google Trends can show the popularity of a brand, social media can tell about what people are thinking about the product, and rating and review websites can show where the brand is lagging. All this is made available through simple big data analytics techniques.
Better operations
Big data technology is in demand for optimizing business processes and operations. By being incorporated in every data-heavy business operations like production line, customer ordering systems, etc. the technology is being used to define efficiencies, finding anomalies, and highlighting when the process needs improvement.
These benefits of big data analytics are being used heavily across sectors like retail, supply chain, telecom, healthcare, and other similar industries.
Tweaking business models
Big data analytics applications have been used by companies to update their current products while coming up with new products and business lines. With a massive set of market data at their disposal, businesses are able to define what their customers are looking for and which businesses are catering to their needs. This information, in turn, is being used to define new products and business models.
The advantages of big data analytics we covered are only the surface level explanations of how companies use big data. There are a number of other ways the technology is being used in the global startup and established business ecosystem. However, for a business that is new in the domain, it is important to understand that these benefits can only happen when you have rightly integrated big data analytics into your business.
[Also Read: How to use Cognitive Analytics to achieve better business outcomes]
How to get started with big data analytics in your business?
An important part of getting all the many benefits that big data technology promises is knowing how to get started with its incorporation in your business model.
At Appinventiv, we have seen this process happening whenever a client comes to us seeking help with creating a big data project.
Big data projects come into existence when a business executive gets convinced that they are missing out of big data benefits. This conviction leads to CMO and CIO teams to work together where they specify and make a scope of the insights that have to be pursued and make analytics architecture around them. Next, the business launches a small IT project where the CIO team engineers a complex system where they capture the raw data and convert them into structured information.
Now, when the analytics professionals start using the data for which the big data model was created in the first place, they start noticing problems in the system and the cycle of iterations begins.
We have witnessed this situation happening multiple times in our data analytics services journey.
For instance, when we created a data analytics platform for a leading telecom company in the US, our first step was to store and analyze their data on the cloud using Apache technologies such as Spark and Hadoop. To make data insights accessible, we deployed different BI solutions through interactive dashboards. The results?
Our efforts led to 100% processing of customer consumption data and 85% increase in data quality and accessibility.
Coming back to getting started with big data analytics in business, what we advise to our clients is firstly, starting very small. It can be as small as identifying the products their customers are viewing and leaving the page from – an information that can be used for sending follow-up email or push notification.
Secondly, we suggest capturing data in a one-off model instead of creating a process for capturing and analyzing data. If you start with capturing the specific data needed to perform the test, you will find the data volume easy to manage. At this stage, we include an analytics engineer. They can highlight what data they need and which should be ignored for a particular big data model.
Once you have mastered these two stages, you can move to the stage of a much broader adoption of big data in business. Incorporating big data through these small, targeted steps can be the least expensive, fastest way to go. It would give business a clarity if a major investment in big data would prove beneficial or how it would pay off in the long-run. It is unquestionable why big data is important, but something which is benefiting others might not benefit you the same way. By running these small-scaled prototypes, you will be able to decide if at all there is a need for big data in your business.
Thirdly, it is important to know the challenges associated with incorporation of big data. Knowing what those are beforehand, would help in taking calculated decisions around the size of the project. Here are some of those challenges –
- Managing data – as the business grows, so does the size of the data which has to be collected.
- Maintaining data quality – businesses end up spending a lot of their time scrubbing data and making them insights ready.
- Keeping data secure – privacy concerns are an inevitable part of big data, especially since the large amount of business data they contain. This is where strict compliance checks come into the picture.
While this highlights the most safe-sided approach of big data incorporation in business, knowing how big data analytics works is equally important. It would give you a framework of how to get started. Let us look into that next as we conclude the article.
How does big data analytics work?
Big data analytics is majorly a four stage process that is used by businesses to get informed data as the outcome. Here’s what those stages are:
Data collection – Here the data (structured, unstructured, and semi-structured) is collected from multiple sources and stored in data warehouses where the BI tools can analyze them. Usually, the highly diverse unstructured data are assigned their individual metadata and are stored in the data lakes.
Data processing – Once the data is gathered and stored, they are processed to get results on queries. To achieve this, two processing options are available – batch processing, where large datasets are looked at over time and stream processing where small batches of data are processed.
Cleaning of data – Data, irrespective of the size, requires scrubbing for bettering the data quality and getting better results. At this stage, the data are formatted in the same context and all the irrelevant, duplicate data are eliminated from the system.
Data analysis – This is the stage where the collected data are converted into insights. Some of the most popular data analytics methods include – data mining, predictive analytics, and deep learning.
Wrapping up!
So here is everything you need to know about getting started with big data analytics. While the approach of integration can vary, the end result should be the same – big data should solve business problems and not make them complex.
If you are looking to add in big data technology in your business and are looking for support to get started, get in touch with our data analysis team. We will sit you to identify the areas where big data services can be most beneficial and work on its incorporation.
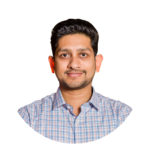
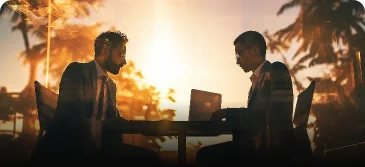
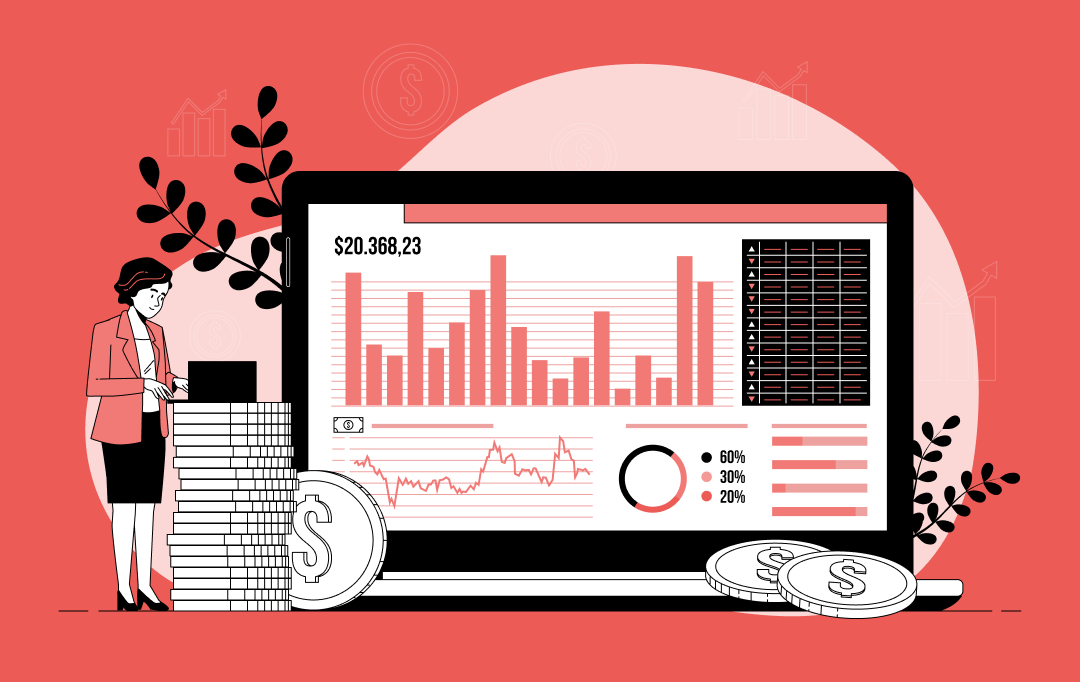
Unleashing the Role of Data Analytics in Driving Smarter Banking Decisions
The use of data analytics in banking is transforming how this data is interpreted and applied. Banks are using this data to make decisions that matter—fraud checks, loan approvals, or marketing campaigns. The focus has shifted: from storing data to using it smartly, and from pushing products to understanding people—being customer-centric. This shift is also…
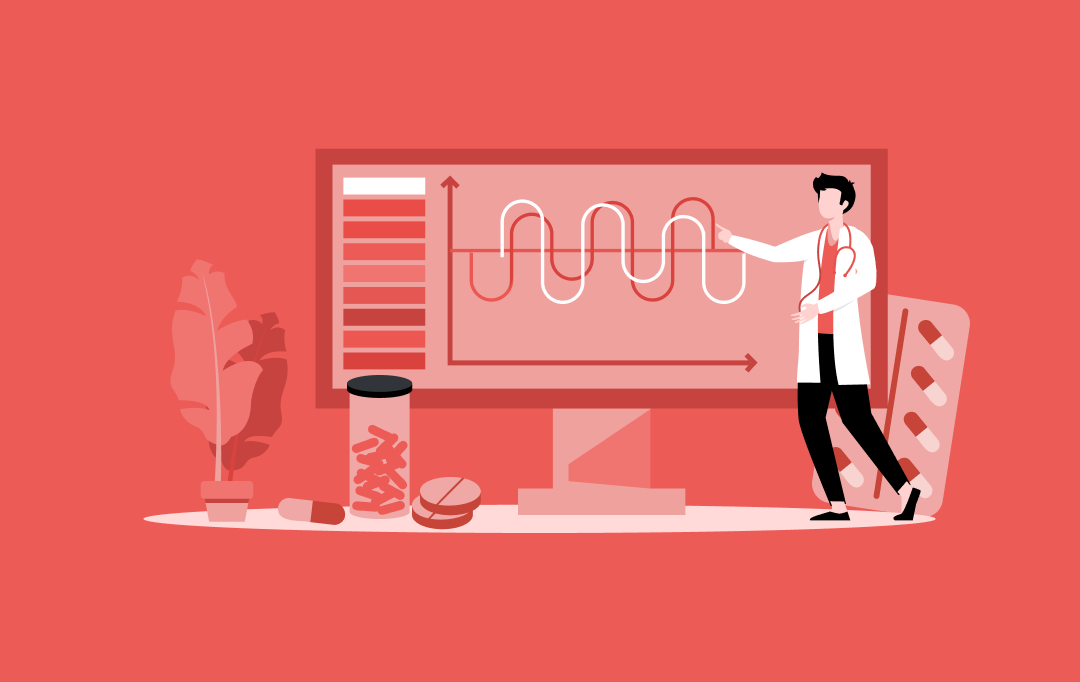
10 Use Cases and Examples of How Pharmacy Data Analytics is Transforming Operations and CX
Data has become the currency of modern business, and the pharmacy sector is no exception. From inventory optimization to regulatory compliance, pharmacies today face many challenges that demand precision and agility. Well, pharma data analytics is solving all these conundrums as the main lead, a transformative approach that now empowers businesses to get actionable insights…
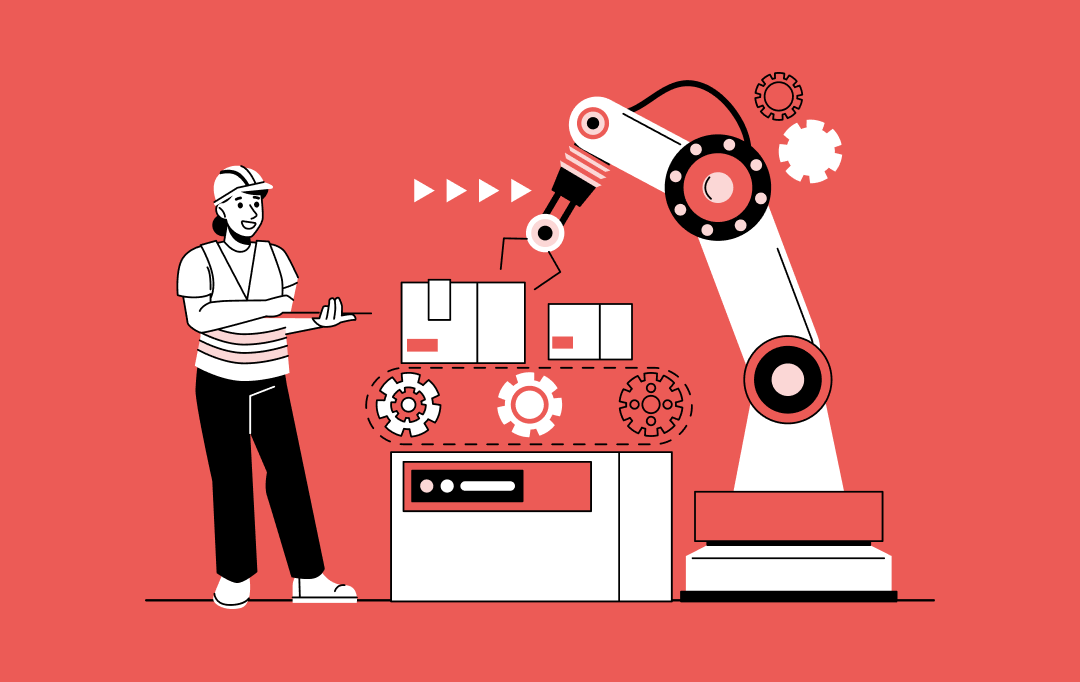
10 Use Cases and Benefits of Predictive Analytics in Manufacturing
Are you trying to stay in today’s manufacturing game with that old-school data analytics approach? Not gonna happen, buddy! Think about that old-school grind: manufacturing plant techies had to hustle around, scribbling notes and logging maintenance records by hand. All that manual intervention and hassle led to nothing more than messy data and bad calls.…