- Product Analytics Market: Global Share and Growth Trajectory
- Why Product Analytics Deserves a Front-Row Seat in Product Strategy
- Intuition Can’t Compete with Insight
- The Real Problems Are Often Quiet Ones
- Better Prioritization Starts with Behavioral Proof
- One Size Never Fits All - And Analytics Proves It
- It Builds a Shared Language Across Teams
- Every Release Becomes a Feedback Loop
- The KPIs That Make Product Analytics Investment Worth It
- Cohort Analysis: Understand how user behavior evolves over time
- Churn Analysis: Detect warning signs before users walk away
- Retention Curve Analysis: Visualize how sticky your product is
- Funnel Analysis: Spot where users drop off - and why
- Conversion Path Analysis: Discover the actual paths users take - not the ones you imagined
- Milestone Analysis: Track progress toward meaningful success moments
- User Journey Mapping: Connect quantitative actions with contextual insights
- Customer Experience Analysis: Overlay user sentiment onto behavior
- Why Product Analytics Isn’t Just Another Data Tool?
- Product Analytics vs. Business Intelligence
- Product Analytics vs. Mobile Analytics
- Product Analytics vs. Data Management Platforms
- Product Analytics vs. Marketing Analytics
- Types of Product Analytics Platforms You Should Know About
- Product Development Analytics
- Product Experience Analytics
- Product Performance Analytics
- User Engagement Analytics
- Retention and Churn Analytics
- Conversion and Acquisition Analytics
- Customer Feedback Analytics
- Growing Your Product Analytics Stack as You Scale
- Startups: Lightweight, scrappy, insight-first
- Mid-sized Companies: Structured tracking, cross-team visibility
- Enterprise: Governance, custom queries, and predictive analytics
- How to Implement Product Analytics Effectively
- Define Business Goals Before You Define Metrics
- Identify Key Events and Metrics That Map to Those Goals
- Set Up a Scalable Event Taxonomy
- Build a Cross-Functional Analytics Ritual
- Choose the Right Tools for Your Stage and Stack
- Visualize, Democratize, and Automate Insights
- Establish Strong Data Governance and Access Control
- Stay Agile: Review, Iterate, and Improve
- Challenges in Implementing Product Analytics (and How We Overcame Them)
- Misaligned Stakeholder Expectations
- Event Tracking Chaos
- Tool Overload, Low Adoption
- Data Silos and Integration Gaps
- Lack of Actionable Insight
- Build Smarter with the Right Analytics Development Partner Like Appinventiv
- FAQs
You’ve launched a product. The interface is clean, the core features are stable, and early reviews are encouraging. But under the surface, there’s a growing list of questions you can’t quite answer.
- Which features are driving retention?
- Why do users drop off halfway through onboarding?
- What’s the real reason behind those five-star reviews – or the churn?
That’s where product analytics comes in. It’s not just about tracking clicks or counting sessions, it’s about understanding why users behave the way they do and turning that insight into smarter decisions.
In a world where user expectations are sky-high and competition is always one release away, flying blind is no longer an option. Product management analytics gives you the visibility to iterate with confidence, identify friction points before they become churn risks, and double down on what’s working.
But here’s the challenge: collecting data is easy. Acting on it? That’s the hard part. Too many teams fall into the trap of overtracking and underanalyzing and end up with more dashboards than direction.
In this article, we’ll unpack why product data analytics should be baked into your product strategy from day one – and how to implement it effectively without getting lost in the weeds. Whether you’re scaling fast or starting, it’s time to stop guessing and start knowing.
Product Analytics Market: Global Share and Growth Trajectory
The global product analytics market is experiencing explosive growth, driven by businesses’ surging demand for actionable insights from massive data sets.
According to Extrapolate, the global Product Analytics Market size was valued at USD 15.11 billion in 2023 and is projected to grow from USD 18.27 billion in 2024 to USD 65.35 billion by 2031, exhibiting a CAGR of 20% during the forecast period.
Why Product Analytics Deserves a Front-Row Seat in Product Strategy
Building a great product is no longer just about launching features and fixing bugs. Today’s users expect seamless experiences, and businesses must move fast, without breaking what’s already working.
Product data analysis helps bridge this gap.
It offers real-time visibility into how users interact with your product, revealing what’s helping them succeed, slowing them down, and quietly driving them away.
It’s not just about measuring outcomes. It’s about uncovering opportunities, validating assumptions, and continuously shaping a product that aligns with how users want to use it, not just how it was designed. Here’s why it matters now more than ever:
Intuition Can’t Compete with Insight
Even the most experienced teams make assumptions about what users want. But the truth is, what feels right inside the war room often doesn’t reflect how people behave in-product. Digital product analytics fills this gap – it shows how users move, where they pause, what they ignore, and which actions lead to conversion or churn.
Take a new feature release, for example. Your team’s confident it’ll boost engagement. But within a week, most users drop off halfway through the workflow. With product analytics tools in place, you don’t have to guess why – it’s clear that users aren’t even discovering a key step pointing to a UX or discoverability issue. This kind of visibility turns instinct-driven decisions into evidence-backed actions.
The Real Problems Are Often Quiet Ones
It’s easy to fix what users complain about. But the more dangerous issues are the ones no one talks about—broken flows, frustrating delays, or confusing layouts that quietly push users away. In the product development process, analytics surfaces these silent friction points long before they appear as churn in your retention dashboard.
Let’s say users sign up but never complete onboarding. Support doesn’t hear a word, and NPS scores stay average. But a closer look at product analytics software shows a steep drop at a single tooltip step. That small hiccup, invisible in feedback forms, is why hundreds of users never make it to their first ‘aha’ moment.
Better Prioritization Starts with Behavioral Proof
Product roadmaps often get shaped by the loudest voices – whether that’s internal stakeholders or a handful of vocal customers. But when decisions are tied to user behavior data, you focus on what drives actual value, not just what sounds good in theory.
Say you’re choosing between two features. One has a strong internal buzz, while the other feels minor. The AI in product development ensures a robust product analytics framework that shows users consistently get stuck where the “minor” feature would help. Now, that decision becomes obvious—it’s not about flash but friction reduction. And that shift leads to faster, more meaningful impact.
One Size Never Fits All – And Analytics Proves It
Different users use your product in various ways. What works for a new user on mobile in Asia might completely miss the mark for a power user on desktop in the U.S., product analytics strategy allows you to segment these behaviors, so you’re not optimizing for a mythical average.
Maybe you’ve built an onboarding experience that works great in English-speaking markets. But your international segments are skipping steps or dropping off early. Without behavioral data, that nuance would be missed, and you’d be building for only part of your audience. You can tailor each segment’s flows, features, and communications with it.
It Builds a Shared Language Across Teams
One of the most underrated benefits of product analytics is cross-functional clarity. Product managers, marketers, designers, and support teams can all rally around the same behavioral insights, so there’s less finger-pointing and more coordinated action.
Imagine marketing is driving a campaign for a feature the product believes is sticky. However, analytics show that adoption is low and drop-off is high. Suddenly, the conversation changes. Instead of debating opinions, the team can align around what’s happening and decide whether to improve the flow, rethink the messaging, or pull the plug entirely.
Every Release Becomes a Feedback Loop
Shipping is no longer the finish line – it’s just the start of the learning cycle. With clear roles of product analytics, every launch becomes an opportunity to validate, iterate, and refine based on real-world usage.
Let’s say you release a new dashboard redesign. It looks better and loads faster, and internal teams are excited. However, early analytics show that users spend less time on it and aren’t completing key actions. That signals that while the visual upgrade worked, the layout needs rethinking. Product analytics use cases turn that hunch into hard data and ensure you’re always learning, not just launching.
The KPIs That Make Product Analytics Investment Worth It
Collecting metrics is one thing. Interpreting them with context? That’s where real product intelligence begins. Analyses act like diagnostic tools – they help you break down behaviors, spot bottlenecks, and understand users’ journey from curiosity to commitment. Below are the most impactful key metrics used in product analytics, each revealing a different dimension of user interaction and product health.
Cohort Analysis: Understand how user behavior evolves over time
Not all users behave the same, and treating them like they do can lead to misleading conclusions. Cohort analysis groups users based on shared starting points (like sign-up week, acquisition channel, or feature adoption) to track how their behavior unfolds.
For instance, you might discover that users acquired through referral campaigns retain better than those from paid ads. Or those who complete onboarding in the first 24 hours become power users. These insights driven from product analytics for business can help you double down on what’s working – and fix what isn’t.
Churn Analysis: Detect warning signs before users walk away
Churn isn’t just a retention problem – it’s a symptom of deeper experience gaps. Product management analytics helps you look at behavioral patterns leading up to user drop-off, assisting teams to identify what actions (or inactions) precede cancellation.
If users consistently churn after ignoring a certain feature or skipping a configuration step, you’ve found a friction point. With that knowledge, you can redesign the experience to address it before it costs you the customer.
Retention Curve Analysis: Visualize how sticky your product is
Retention curves show how many users return on day 1, day 7, day 30, and beyond, giving a clear picture of user longevity. But they also help highlight activation moments.
Say your curve flattens out after week two – that plateau can signal success (your core users are staying) or stagnation (growth has stalled). Layering in user behavior at each point in the curve helps explain the ‘why’ behind the trend.
Funnel Analysis: Spot where users drop off – and why
Every product has key flows – signup, onboarding, checkout, and upgrade. Funnel analysis breaks these into stages and shows where users abandon the journey.
For example, an ecommerce app might see strong product views but weak checkouts. That’s a sign to investigate pricing, trust signals, or payment UX. Product data analytics funnels show you where the leaks are and prompt targeted experiments to fix them.
Conversion Path Analysis: Discover the actual paths users take – not the ones you imagined
Unlike linear funnels, conversion paths are messy and often surprising. These analytics for product managers help trace the actual steps users take before completing a goal: making a purchase, upgrading a plan, or finishing a project.
It might reveal that users are taking five clicks to do something you assumed needed two. Or that a popular help article is consistently visited before upgrades. These real-world paths help you simplify, optimize, and guide users toward conversion more smoothly.
Milestone Analysis: Track progress toward meaningful success moments
Users don’t just want features – they want outcomes. Milestone part of product data analysis helps teams track whether users reach moments that unlock long-term value, such as “published first post” or “invited a teammate.”
These are often the moments that separate casual users from committed ones. Identifying them lets you redesign onboarding or notifications to steer more users in that direction and, as a result, increase retention.
User Journey Mapping: Connect quantitative actions with contextual insights
The user journey part of digital product analytics maps the entire lifecycle, from first touch to power user status. This approach combines behavioral data (clicks, session times, conversions) with contextual markers like device, geography, or source.
It can uncover patterns like mobile users bouncing after hitting a non-optimized screen, or international users struggling with currency settings. By viewing the journey holistically, teams can solve local and systemic issues.
Customer Experience Analysis: Overlay user sentiment onto behavior
Sometimes the data says “this feature is used a lot,” but users still don’t like it. Customer experience software development integrates NPS, CSAT, survey results, and support feedback into product data to fill that gap.
If a popular feature has high usage but low satisfaction, it’s a sign of forced engagement, not real value. By aligning quantitative metrics with AI sentiment analysis, CX-based product analytics tools ensure you’re improving the experience, not just the numbers.
Why Product Analytics Isn’t Just Another Data Tool?
It’s easy to assume that product analytics is “nice to have” when your stack includes BI dashboards, mobile SDKs, or marketing analytics. However, each of those tools was built for a different job. Product development analytics isn’t about broad trends or attribution models – it’s about what happens inside your product, in real time, with real users. And no other system offers that lens with the same level of clarity or actionability. Here’s how it stacks up against other popular tools:
Product Analytics vs. Business Intelligence
BI tools like Power BI, Tableau, or Looker are built for broad, high-level reporting. They’re great for finance, sales, and executive dashboards. But they weren’t designed to help product teams answer rapid-fire questions like “Which onboarding step sees the most drop-offs?” or “Do activated users behave differently by device type?”
Product analytics software is lightweight, real-time, and granular. It empowers PMs, UX designers, and growth leads to ask product-specific questions and get answers without waiting for a data analyst or engineer. You don’t need SQL to uncover what matters – context, filters, and speed.
Bonus Read: Business Intelligence vs Business Analytics
Product Analytics vs. Mobile Analytics
Mobile analytics platforms like Firebase or Adjust help track performance metrics like crash rates, screen loads, and device types. They’re great for maintaining app stability and understanding install trends, but they miss the mark on user intent.
The product analytics framework zooms out from individual technical events and captures how users engage with features, their in-app paths, and where they find (or don’t find) value. It isn’t just about mobile – it’s about behavior across every product surface, including web, tablet, and embedded systems.
Product Analytics vs. Data Management Platforms
DMPs are essential for creating audience segments and fueling targeted marketing campaigns. They’ll tell you who your users are, where they came from, and what ad brought them in. But they won’t show whether those users engaged with your product, or how long they stayed.
Incorporating analytics as a product allows you to go beyond the acquisition moment and into the full user lifecycle. You can measure onboarding completion, track usage depth, or identify early churn indicators – insights no DMP can deliver independently.
Product Analytics vs. Marketing Analytics
Marketing analytics helps you optimize campaigns, channels, and conversion rates. It’s excellent at answering what brought the user in. But it ends where the user journey inside your product begins.
That’s where product analytics for businesses takes over—tracking what happens post-click or post-signup. For example, your campaign may drive a 10% click-through rate, but the ROI disappears if those users never make it past onboarding or don’t return after the first week. Product analytics helps connect marketing efforts to product stickiness so that you can align spending with long-term value.
Every tool in your stack plays a role. However, product analytics only gives you a real-time, end-to-end view of user behavior within the product. It helps you ship more confidently, optimize with purpose, and build features that solve user needs. In today’s market, relying solely on BI, mobile analytics, or marketing dashboards is like building a house with only a hammer. Analytics as a product can be the full toolbox.
Types of Product Analytics Platforms You Should Know About
Product management analytics isn’t a one-size-fits-all solution. Different tools serve different purposes depending on your product’s stage or the question you’re trying to answer. Some dive into feature performance, others into user journeys, or technical health. Understanding these categories can help you invest in the right product analytics tools instead of a bloated one.
Product Development Analytics
These platforms are designed to inform design and development decisions. They help teams validate features, track adoption, and identify friction points early on.
- What they measure: Feature usage, interaction heatmaps, click paths, and drop-off points during key flows.
- Use case: You launch a new dashboard feature but notice only 30% of users click beyond the first tab. A product development analytics tool reveals users are confused by the layout, saving your team months of blind iteration.
- Popular tools: Pendo, Amplitude, Mixpanel
Product Experience Analytics
Experience-focused product analytics software analyzes your product’s usability and fluidity. It’s about more than just function—how intuitive and smooth your product feels to use.
- What they measure: Session recordings, heatmaps, rage clicks, scroll depth, and navigational behavior.
- Use case: If users repeatedly click on a non-clickable element or abandon the page mid-scroll, these tools highlight your UX blind spots.
- Popular tools: Hotjar, FullStory, Smartlook
Product Performance Analytics
These product analytics for business platforms monitor how well your product performs technically—speed, stability, and responsiveness—all the things users notice instantly but rarely articulate.
- What they measure: Load times, crash rates, API latency, error logs, system uptime.
- Use case: You see a spike in churn and find out a specific API failure is crashing sessions for users in one region. Performance analytics catches it before support tickets pile up.
- Popular tools: Datadog, Sentry, Firebase Crashlytics
User Engagement Analytics
These product management analytics tools help you understand how deeply users interact with your product, what keeps them returning, and what signals long-term value.
- What they measure: The session frequency, feature adoption, time-in-app, and MAU/DAU ratios.
- Use case: You notice power users tend to use a certain feature daily. That insight can help prioritize what to improve or offer in a premium plan.
- Popular tools: Heap, Amplitude, Mixpanel
Retention and Churn Analytics
Retention tools uncover patterns behind long-term user behavior. They help pinpoint when and why users leave, allowing your team to course-correct before it’s too late.
- What they measure: Retention cohorts, drop-off timelines, lifecycle trends, re-engagement rates.
- Use case: A cohort analysis reveals that users who complete onboarding within the first day are three times more likely to stay after a month. That insight drives a redesign of your welcome flow.
- Popular tools: Mixpanel, CleverTap, Woopra
Conversion and Acquisition Analytics
These product data analytics software focus on what happens between awareness and activation. They help track acquisition sources and how well those users convert into meaningful product actions.
- What they measure: Conversion funnels, source attribution, campaign ROI, trial-to-paid movement.
- Use case: Users from one ad campaign have high activation but low retention. This insight helps you align your product narrative with your acquisition strategy.
- Popular tools: For analytics for product managers: Google Analytics, Branch, Appsflyer
Customer Feedback Analytics
Sometimes numbers alone aren’t enough. Feedback platforms turn open-ended responses into structured insights, helping you hear the why behind the what.
- What they measure: NPS scores, in-app surveys, satisfaction ratings, and user comments.
- Use case: Users keep mentioning “too complex” in feedback. When layered with usage data, you discover friction in setting configuration, a fix that improves satisfaction and engagement.
- Popular tools: Delighted, Qualtrics, Chameleon
Growing Your Product Analytics Stack as You Scale
Your product data analysis stack shouldn’t be static. What works for a 5-person startup won’t cut it at the enterprise level. But that doesn’t mean you need a dozen tools out of the gate. A scalable stack grows in complexity and depth alongside your product, team, and customer base.
Here’s what a smart, stage-wise evolution can look like:
Startups: Lightweight, scrappy, insight-first
At this stage, speed matters more than complexity. You’re shipping fast and experimenting weekly, and your biggest questions are about feature adoption, onboarding friction, and basic retention.
What you need:
- One end-to-end product analytics tool (e.g., Mixpanel, Amplitude, Heap)
- Funnel and cohort tracking
- Basic event tracking (signups, activation, first key action)
- Lightweight heatmaps or session replays (e.g., Hotjar or Smartlook)
Focus:
Startups don’t need big dashboards and features-heavy digital product analytics – they need answers. How many users are dropping off before the ‘Aha’ moment? What features are getting used? Prioritize tools that are easy to set up and don’t require a dedicated analyst or engineer.
Mid-sized Companies: Structured tracking, cross-team visibility
Now you’ve got traction. Multiple teams are involved—product, growth, marketing, and more users mean more complex user journeys. You need deeper segmentation, lifecycle tracking, and the ability to connect product data to marketing or sales platforms.
What you need:
- Event schema governance (naming conventions, documentation)
- Deeper funnel breakdowns and path analysis
- Retention tracking across cohorts
- Attribution tools (e.g., Appsflyer, Branch)
- Customer feedback tools (e.g., Chameleon, Delighted)
- Integration with CRMs, marketing automation, and customer support systems
Focus:
Build consistency across teams. In this stage, misaligned definitions (e.g., what counts as an active user?) can cause chaos. Introduce event taxonomies, shared dashboards, and cross-functional rituals around metrics.
Enterprise: Governance, custom queries, and predictive analytics
You’ve scaled. Now the challenge is speed, governance, and keeping everyone aligned. Product analytics software must support cross-functional strategy, regulatory compliance, and predictive modeling.
What you need:
- Data warehouse integration (Snowflake, BigQuery, Redshift)
- Custom dashboards for teams and leadership
- SQL-layer flexibility + no-code interfaces
- Role-based data access and audit trails
- Predictive models for churn, LTV, and conversion
- Feature-level experimentation tools (e.g., LaunchDarkly, Optimizely)
Focus:
At this level, product analytics becomes part of a larger decision engine. The tools you use should help drive roadmap prioritization, revenue strategy, and long-term user retention. It’s also the time to bring in data engineers and analytics leads to maintain a clean, scalable infrastructure.
Irrespective of what your stage is, the goal is the same:
Use product data to make better, faster decisions. But how you get there – and what your stack looks like – should evolve with you. Build enough at each stage to stay ahead of the curve, not buried under it.
How to Implement Product Analytics Effectively
Product analytics for business is only as good as how it’s implemented. Dumping events into a dashboard without a clear plan leads to noise, not insight. Successful implementation requires a mix of strategy, tooling, collaboration, and a culture of continuous iteration. Here’s a step-by-step approach to doing it right:
Define Business Goals Before You Define Metrics
Before you track anything, get crystal clear on the outcomes you’re trying to drive. Are you improving retention, optimizing trial-to-paid conversion, or increasing feature adoption?
Why it matters: Without defined goals, your analytics will become a vanity metric machine. Instead, align your product analytics plan directly to your business objectives—whether reducing churn by 20%, improving onboarding success, or expanding ARPU.
Identify Key Events and Metrics That Map to Those Goals
Once goals are in place, work backward to determine the events and metrics that signal progress. This might include funnel drop-offs, activation milestones, or usage frequency.
Examples:
- For onboarding: track “completed setup,” “invited team member,” or “created first project.”
- For retention: track weekly active use of core features or repeat purchase behavior. Use frameworks like HEART (Happiness, Engagement, Adoption, Retention, Task success) to cover quantitative and qualitative indicators.
Set Up a Scalable Event Taxonomy
Define a consistent schema for naming events, properties, and user identifiers. This becomes especially critical as your product grows and more teams rely on shared data.
Actionable Tip: Use a central event dictionary. Keep naming conventions descriptive and human-readable (e.g., Button Clicked: Upgrade CTA vs. btn_clk_1), and document why each event matters.
Build a Cross-Functional Analytics Ritual
A single team should not own your product management analytics setup. Involve product managers, designers, marketers, customer success managers, and engineers early on.
Why it matters:
Cross-functional collaboration ensures that you ask the right questions from day one and consistently act on them. Teams can co-own metrics, contribute hypotheses, and stay aligned through shared dashboards and regular reviews.
Choose the Right Tools for Your Stage and Stack
Pick product data analytics tools that match your goals, team size, and technical maturity. Don’t default to the most popular platform – default to what gives your team clarity and velocity.
Examples:
- Early stage: Amplitude, Mixpanel, Heap
- Mid-growth: Add tools like Segment, FullStory, or Mode for richer insights
- Enterprise: Integrate with Snowflake, Looker, or dbt for custom modeling and access control
Visualize, Democratize, and Automate Insights
Don’t just collect data – make it visible. Build live dashboards in your product data analysis tool for each goal and make them accessible to all stakeholders. Set up alerts for critical changes (e.g., a drop in daily active users or spike in failed logins).
Pro tip: Automate reporting and flag anomalies so teams can focus on action, not extraction.
Establish Strong Data Governance and Access Control
As your setup matures, so do the risks. Implement governance to avoid duplication, confusion, or data misuse.
Implementation must-haves:
- Role-based access to sensitive data
- Audit logs for data changes
- Documentation for every tracked event
- Clear process for introducing new metrics or tools
Stay Agile: Review, Iterate, and Improve
Don’t treat your digital product analytics setup as a one-and-done project. Revisit dashboards monthly, prune unused metrics, and evolve your tracking based on new product strategies.
Best practice: Build a feedback loop where learnings from data are folded into the roadmap and retested. Analytics should always lead to action.
When implemented right, product analytics software becomes more than a reporting tool—it becomes your team’s compass. It reveals what’s working and signals early what’s not before it snowballs into lost users or revenue.
Let’s design your scalable analytics Strategy
Challenges in Implementing Product Analytics (and How We Overcame Them)
Product analytics sounds straightforward in theory – track, analyze, act. But in practice, teams often hit friction points that can delay, derail, or dilute the impact of their analytics efforts. We’ve seen it firsthand.
At Appinventiv, we’ve helped multiple clients build and scale custom product analytics platforms from the ground up. In the early days of this journey, we faced our share of challenges, especially around aligning stakeholder goals, defining meaningful metrics, and setting up scalable event tracking systems.
Here are some common product analytics challenges we’ve encountered (and how we solved them):
Misaligned Stakeholder Expectations
Different teams often want different things from analytics—growth wants conversions, product wants feature usage, and executives want business impact. Without early alignment, analytics becomes fragmented fast.
How Appinventiv solved it:
We now begin every analytics build with a discovery sprint. This includes joint workshops with stakeholders across product, marketing, and leadership to define north star metrics and supporting KPIs before implementing a single event.
Event Tracking Chaos
One of the biggest challenges was implementing a consistent and future-proof event schema. In one case, a client had hundreds of custom events – most of which no one was using, or even sure what they meant.
How Appinventiv solved it:
We created a shared event taxonomy framework that includes event names, descriptions, properties, and ownership. All our implementations now follow this standard, reducing redundancy and making dashboards meaningful from day one.
Tool Overload, Low Adoption
Sometimes, clients came to us with half a dozen tools stitched together, but no one was using them effectively. Others had tools that were too advanced for their team’s maturity.
How Appinventiv solved it:
We match tools to both the current stage and future goals. For startups, we integrate flexible tools like Heap or Mixpanel with minimal engineering lift. For larger orgs, we recommend warehouse-native setups with dbt or Looker for deep custom queries.
Data Silos and Integration Gaps
Product insights get trapped without a unified data layer – one team sees usage, another sees revenue, and no one sees the full picture.
How Appinventiv solved it:
We push for early adoption of tools like Segment or RudderStack to create a single data collection and distribution pipeline. It helps unify product, marketing, and customer data under one roof.
Lack of Actionable Insight
Tracking data is easy. Making it actionable is hard. One client had great dashboards but couldn’t tie them back to product decisions.
How Appinventiv solved it:
We embed feedback loops into product rituals, such as monthly reviews, experiment retrospectives, and roadmap planning sessions. Dashboards aren’t standalone—they’re built to support real conversations and choices.
Every product analytics journey is different, but the roadblocks tend to rhyme. Having gone through the trenches across industries, we know where things typically break – and more importantly, how to build with resilience from the start.
Build Smarter with the Right Analytics Development Partner Like Appinventiv
In a product-led world, guessing is a luxury no business can afford. Product analytics helps teams ship with purpose, adapt quickly, and grow confidently. But getting it right requires more than tools—it demands strategy, alignment, and deep technical execution.
That’s where Appinventiv comes in.
Whether starting from scratch or looking to scale your existing analytics stack, our product development services have helped global businesses design and implement product analytics platforms tailored to their product vision and growth goals. From setting up a clean event taxonomy to integrating custom dashboards that fuel decision-making, we don’t just build analytics systems; we create clarity.
Ready to turn your product data into a competitive edge? Let’s build something that speaks with numbers and scales with purpose.
FAQs
Q. What is Product Analytics?
A. Product analytics for businesses involves collecting and analyzing user interaction data to understand how people engage with your digital product. It reveals what features users love, where they drop off, and what drives them to convert or churn, helping teams make smarter product decisions based on actual behavior, not assumptions.
Q. Why is Product Analytics Important?
A. Building a great product is not just about vision—it’s about validation. Product analytics provides concrete insights into user behavior, allowing teams to iterate faster, reduce guesswork, and focus on what truly matters. It bridges the gap between what users think they want and what they actually do inside your product.
Q. Who Uses Product Analytics?
A. Product managers, growth teams, marketers, designers, and leadership rely on product analytics for different reasons. Analytics for product managers is used to prioritize features, growth teams to optimize funnels, marketers to personalize experiences, and execs to track business impact. It’s a cross-functional superpower when used right.
Q. How to Grow Your Product Analytics Stack with Your Organization
A. As your product evolves, so should your product management analytics setup. Startups often begin with plug-and-play tools like Mixpanel or Amplitude to capture basic engagement and retention metrics.
As your user base and data complexity grow, layering in tools for warehouse syncs, advanced modeling, and custom reporting becomes essential. Enterprises typically invest in unified analytics stacks that support multi-product tracking, granular cohorting, and automated insights across departments.
Q. When Should a Company Invest in a Product Analytics Tool?
A. Ideally, but not before defining what you want to learn as early as possible. The best time to invest in product data analytics is once you have an MVP or beta version live and need user feedback to refine it. The earlier you start tracking, the more data you’ll have to guide your roadmap. Waiting too long can mean flying blind or making costly assumptions.
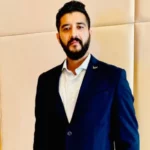
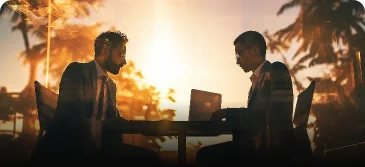
- In just 2 mins you will get a response
- Your idea is 100% protected by our Non Disclosure Agreement.
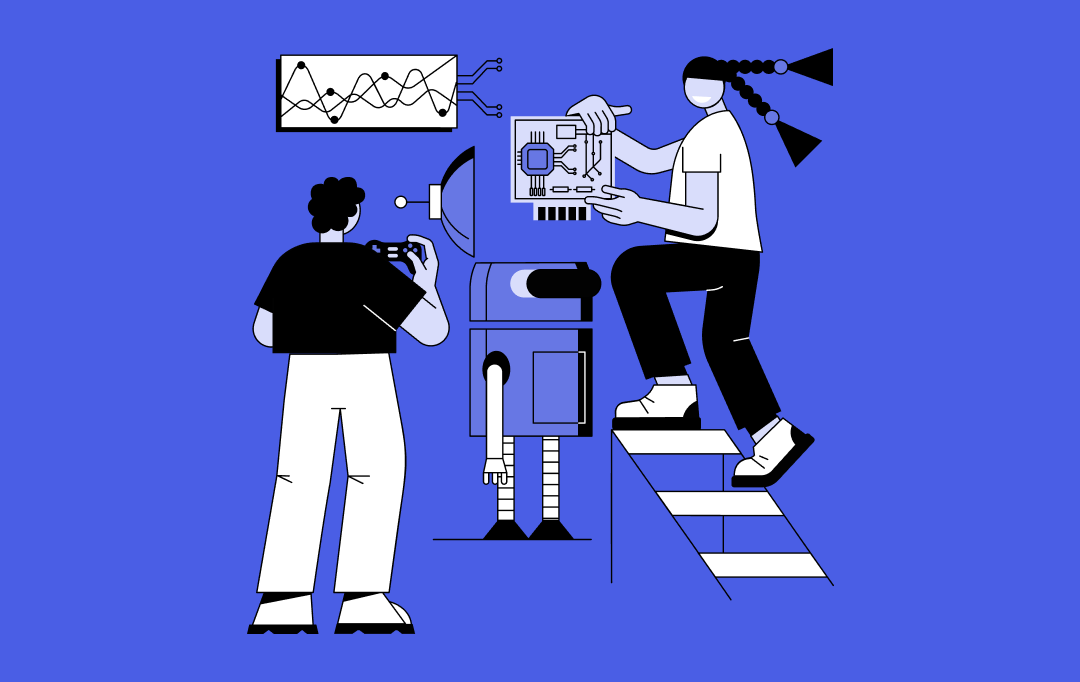
Exploring the Key Benefits and Use Cases of Digital Twins in Product Development for Enterprises
The major survivor factor is the companies' ability to develop, launch, and enhance the product. McKinsey predicted that - over the next five years, about $30 trillion in corporate revenues will depend upon products that have not yet reached the market. Consumers also need compelling reasons to retain the purchase of your product - advanced…
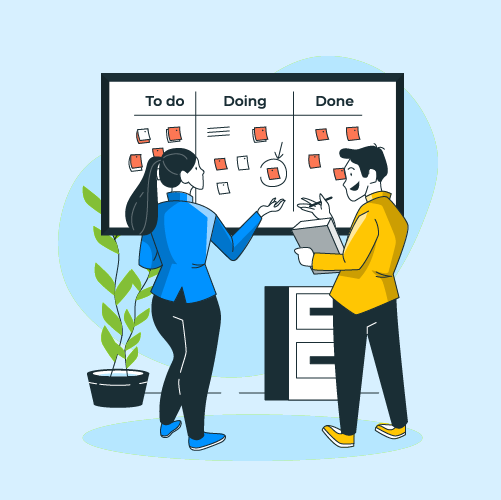
Have you ever had the feeling that your team makes the same mistakes again and over? You believe things are not progressing correctly, and you need to make some changes to improve the project development process. A product backlog here can help a team decide what they're working on and what they want to focus…
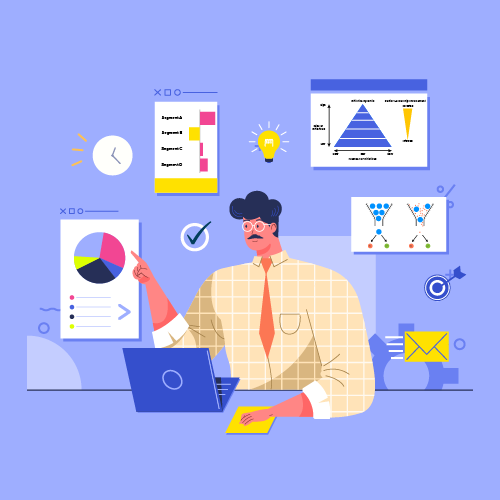
Best 5 Diagrams Used To Explain Product Management Concepts
There are a lot of things product managers are accountable and responsible for. A product manager is not only liable to strategize by creating a roadmap but also needs to articulate the release cycle of a new product to the team along with everything that comes in between. They are also required to have the…