- Cohesion Of AI and ML in Fintech: How It Translates for Millennials and Gen Zs
- Top 10 Use Cases of AI in Fintech: With Real Examples
- Algorithmic Trading
- Underwriting and Credit Scoring
- Automation in Customer Service
- Fraud Detection/Prevention
- Personalization in Banking Services
- Compliance Automation
- Portfolio Management
- Anti-Money Laundering
- Financial Forecasting
- Climate Finance & Investment Tracking
- Challenges of AI in the Fintech Industry
- Regulatory Compliance
- Bias and Fairness
- High Implementation Costs
- Potential Solutions to the Above Roadblocks
- How Appinventiv Can Help in AI Implementation for Your Fintech Firm
- FAQs
The release of ChatGPT in late 2022 has exponentially accelerated the global trend towards generative AI to streamline operations and reduce recurring tasks. This generative AI explosion has assisted in opening new vistas for fintech organizations to explore more.
Business Insider reveals some interesting data on how big banks use AI in the fintech industry and benefit from it.
- Goldman Sachs is leveraging AI for internal research and IPO prospectus drafting, emphasizing the acceptance of AI solutions in the fintech industry.
- JPMorgan CEO Jamie Dimon uses AI to outpace its market competitors, which is followed by major investments.
- By automating the research process, Mako AI and Louisa AI (startups) also leverage AI to attract major financial clients.
- Companies like Blackstone also use AI tools for risk analysis and deal sourcing.
- Morgan Stanley also uses OpenAI-powered tools to accelerate their decision-making and support advisors.
This AI revolution is highly appealing to millennials, but why? Because they value ease, personalization, and speed, all of which AI delivers via:
- Instant payments
- Customer service
- Tailored financial advice
Artificial intelligence (AI) in fintech empowers users with financial literacy. It is not just an innovation but also parallels millennials’ values and lifestyles. Various banks use AI in their processes.
Adopting digital banking ease and mobile payment platforms has significantly accelerated the use of AI in Fintech, which is necessary in fraud prevention, algorithmic trading, etc.
The data also revealed that digital adoption is led by 64% of Gen Zs and 68% of Millennials, thus proving the role of millennials in driving the AI game in the Fintech industry. Thus, with an expected value of USD 97.70 billion by 2033, AI in the Fintech industry is poised to exhibit a CAGR of 19.90% during 2025-2033. [Source: IMARC Group]
Build Your AI-Powered Fintech Platform Today and Start Your Journey
Cohesion Of AI and ML in Fintech: How It Translates for Millennials and Gen Zs
UnaFinancial states that by 2030, fintech AI will play a crucial role in shaping the current financial landscape.
Fintech users in the Southeast Asia region will reach 505.6 million from the current 400 million, with Millennials and Gen Zs making up 79% of the user base. It also states that by 2030, Millennials will make up 40.09% of fintech users, followed by Gen Z at 38.5%. [Source: e27].
But why are we emphasizing millennial users? For them, AI and ML in fintech won’t be just backend tools but a definition of effective user experience. AI models are continuously trained via user behavior and other data variables, providing real-time solutions to new problems. Moreover, this user base considers mobile-first interactions a default preference and distrusts outdated financial institutions. This is where the cohesion of AI in fintech trends proves to be essential. The experience translates into apps and software, automating tasks and delivering real-time insights.
“Banking has to work when and where you need it. The best advice and service in financial services happen in real-time and are based on customer behavior, using principles of Big Data, Mobility, and Gamification.”
By Brett King, a Fintech Futurist
Now that you’ve explored the need for AI in fintech, let us examine the top 10 use cases of how AI is revolutionizing the financial services sector.
Top 10 Use Cases of AI in Fintech: With Real Examples
Imagine a world where your banking partner predicts your needs in advance, reports false flags before they happen, and also helps you invest with planning. It is all possible now, thanks to AI. Artificial Intelligence in fintech is no longer a buzzword as it continuously reshapes the banking infrastructure.
JPMorgan plans to scale from 450 to 1000+ AI use cases in 2025, highlighting its strategic commitment to AI-driven transformation and the growing impact of artificial intelligence in financial services.
But Why? Because the bank’s sales grew 20% between 2023 and 2024, which is attributed to generative AI tools. Seeing the benefits, the bank has deployed its GenAI toolkit to 200,000+ employees, thus democratizing access across multiple functions. [Source: Reuters]
Approaching forward, let us now explore AI Use Cases in Fintech, along with real example-based insights:
Algorithmic Trading
Algorithmic trading is among the major use cases of AI in the fintech industry, thereby transforming how banking firms approach the market. The core of algorithmic trading involves using machine learning algorithms and advanced models like Supervised Learning, Reinforcement Learning, etc, to analyse large sets of structured and unstructured financial data to detect patterns, execute high-speed trades, and forecast price trends, all within minimum human intervention.
“A good mathematical model of a complex system gives you insights that aren’t available from intuition.”
By Edward Thorp, a Mathematician
This is true because an algorithmic model relies on statistical and rule-based learning to analyze market data, detecting unidentifiable patterns to the human eye. Apart from increasing market liquidity, it enables precise and cost-effective investment decisions.
Real Example: XTX Markets
In 2024, XTX Markets, a London-based algorithmic trading firm, surfaces as a strategic example of the successful adoption of AI in Fintech. Researchers at XTX use ML to scan large market data points to build statistical models.
As per the reports, XTX’s initial success was derived from cracking the Foreign Exchange market. Adopting algorithmic trading helped them gain a strong foothold in the market, which high-frequency AI firms have not penetrated. Seeing the boom, XTX focused on investing around $199 million in AI chips, making them overpower all other firms. [Source: Financial Times]
Underwriting and Credit Scoring
The AI-powered underwriting and credit scoring have transformed credit access, especially for millennials who deploy AI in fintech. And let’s face it, the traditional credit scoring process hasn’t been seamless. The loan approval process can be a hassle if you don’t have a good credit history or a full-time job. This is where the role of AI in fintech proves to be beneficial.
AI assesses a broader picture instead of just evaluating a single variable like past borrowing behavior. Things like utility bills, rent payments, and employer history are analyzed to give younger folks and gig workers further access to the credit they deserve. This allows bank firms and fintech companies to automate the lending process entirely, offering instant approvals and rejections to loan applicants, thus proving it as one of the prominent AI use cases in fintech.
Real Example: Zest AI
In 2024, the company, now a leader in AI-automated writing, reported significant growth, driven by the goal of making lending more inclusive. With AI for fintech, Zest AI has clocked more than 50% average annual customer growth, with over 175 clients collectively serving more than 110 million users in 2023 alone.
With the original idea around credit unions, the company has expanded into community banking, auto lending, home equity, and small business finance. This strategic alignment with technology has helped Zest AI to be in the top 1% of global companies regarding customer satisfaction. [Source: FINSMES]
Automation in Customer Service
Among the most distinguished AI use cases in fintech is its implementation in offering customer service automation. Thanks to AI-powered virtual assistants and chatbots, daily inquiries can now be handled instantly.
Bonus Read: AI Chatbots in Banking
These AI-powered systems assist by responding to common questions, including checking account balance, updating personal details, and understanding loan terms. Powered by fintech AI, these bots not only respond to questions but also learn from daily interactions and adapt their responses based on the tone of the front user.
However, it is not all. When a chatbot cannot answer a query, it escalates the ticket to a live agent, thus posing as a front-line assistance to immediate queries, eliminating human intervention. In short, this aspect of artificial intelligence in fintech is a perfect example of fast, intuitive, and personalized support, where it is exactly required.
Real Example: Bank of America – Erica
Bank of America has been a prime example of successfully deploying its chatbots, which, since their launch in 2018, have handled over 50 million customer requests. This case story reflects the rising dominance of AI in banking and finance, where automation is the new normal.
The result was that 98% of users received satisfactory answers without human intervention. Since the launch, more than 50,000 updates have been patched, improving its natural language understanding capabilities. Moreover, industry experts consider AI chatbots the central tool for financial services, thereby slowly eliminating legacy systems. Erica is a prominent example of how AI is used in the finance industry and how other firms can leverage AI for their benefit. [Source: PYMNTS]
Fraud Detection/Prevention
One of the potent use cases of AI in fintech revolves around fraud detection and prevention. Apart from financial services being costly, it also serves as a trust line between customers and financial lenders. Traditional systems work on static rules (flagging a transaction based on a threshold amount), exactly where AI overpowers them. Using ML to analyse vast datasets based on transactional data and user behavior, AI in finance can flag the following scenarios:
- When your card is used in a different country
- When high-risk purchases are made in a short time
What makes AI in fintech better is its ability to learn from past fraud patterns, thus making it highly relevant in today’s digitally risk-prone scenarios. For customers, especially the younger generation whose use cases depend heavily on mobile banking, fraud detection using AI offers a safe and secure way to execute transactions.
Real Example: Ally Financial
Ally Financial is a perfect example of a banking firm that has successfully integrated AI and RPA in finance to combat identity fraud, which is common in auto lending. The firm uses AI in financial services to detect inconsistencies and potential red flags that indicate fraudulent activity. But how?
Ally Financial’s AI cross-references user data with a database of known fraud information, which also extends to the dark web. Since its launch in 2023, the system has continuously learned and adapted, improving its ability to detect evolving patterns and making the lending experience secure and seamless. [Source: Auto Finance News]
Personalization in Banking Services
Banking personalization services are among the most impactful applications of AI in finance. Imagine logging into your banking app and getting tailored financial advice, smart tips, and product recommendations that match your lifestyle. This is what the future of AI in finance looks like. As this aspect is taking on a high progression rate, you, as a business owner, should definitely consider aligning with these current trends to maintain the experience level of the users.
Bonus Read: The Ultimate Guide to Banking App Development
By analysing a vast customer dataset (spending habits, transaction history, income patterns), AI helps create a personalized banking experience. The benefits not only include the above use cases, but also include offering:
- Personalized loan options
- Suggestions regarding savings plans
- Daily nudges as money-saving tips
From a business standpoint, using AI in fintech benefits the firms by increasing customer loyalty, engagement, and satisfaction, making it a win-win for both parties.
Real Example: Cleo
Founded in 2016, Cleo, a UK-based Fintech, looked forward to resonating with millennials and Gen Z users seeking personalized finance-related guidance. By leveraging generative AI in fintech via models like GPT-4o, the firm was able to offer real-time insights into users’ budgeting habits, strategies, and savings methodology.
This integration doubled Cleo’s ARR to $185 million, reflecting massive acceptance of AI in the fintech industry by first-hand users. The company understands engagement and caters to users by highlighting their spending patterns through their exclusive “Roast Mode”. [Source: The Times and Cleo]
Compliance Automation
Compliance automation is one significant example of how AI is used in finance and how it transforms financial institutions in managing regulatory requirements, making faster and accurate processes. Automating KYC procedures is one key area where AI is making a fundamental difference.
Traditionally, KYC procedures involve manually verifying a customer’s identity and ensuring all necessary documentation meets the criteria. By leveraging AI in financial services, such as blockchain technology for KYC, customer documents, anomaly detection, and data cross-referencing, are automatically processed via ML algorithms, reducing processing times and ensuring real-time customer activity monitoring.
Real Example: ING Bank
With assets totalling more than $1.1 trillion, ING Bank utilizes generative AI in the fintech market to improve its KYC procedures. Integration of large language models in the fintech industry helps data analysts skip time-consuming data preparation and begin immediate analysis. The benefits were as follows:
Client outreach was reduced as AI could autonomously gather and summarize the required user information
Productivity increased via AI-generated summaries on geography and involved product risks
The quality of compliance workflows has improved, with generative AI in fintech supporting risk evaluations [Source: Bank Automation News]
If you are interested in learning more about regulatory compliance software for the financial industry, you should read our blog on How to Develop Regulatory Compliance Software for the Financial Industry.
Portfolio Management
When we talk about the future of AI in fintech, Portfolio Management emerges as one of the many use cases of AI in fintech. Post-leveraging vast datasets and advanced algorithms, AI systems can assess individual risk tolerance and process market conditions while optimizing real-time asset allocation.
ML techniques like deep reinforcement learning help optimize portfolios by balancing risk-return trade-offs. These models can effortlessly adapt to volatile market conditions and investor preferences.
Real Example: Schroders Capital
With $94 billion in assets under management, Schroders Capital launched its generative AI analyst platform to speed up data analysis and enhance investment decision-making. This tool aims to automate daily investment tasks, allowing in-house analysts to focus on other high-value activities and further opening up newer possibilities for implementing conversational AI in financial services like payments.
The firm’s broader strategy involves deploying the tool to over 1000 employees daily to help improve productivity across the business. The circular aim is to elevate investment and portfolio analysis from the shackles of traditional ways via their generative AI solutions in the fintech industry. [Source: Schroders Capital]
Leverage Our AI Development Services & Suggest Tailored Offers to Your Users.
Anti-Money Laundering
Traditionally, anti-money laundering compliance heavily relied on rule-based systems and manual processes to generate high false positives and miss fraud patterns. Thanks to continuously improving AI applications in fintech, anti-money laundering is one of the best implementation examples of AI in financial services.
By analysing vast volumes of transactional data, irregular patterns that might indicate money laundering are identified. Some high-risk behaviors are analyzed by ML algorithms, which are:
- Immediate movement of large sums
- Inconsistent customer activity
- Out-of-the-blue international transfers
This automation helps detect accuracy while also reducing investigation time and compliance costs.
Real Example: HSBC
HSBC is harnessing AI to increase its capability to detect and prevent finance-related crimes. Through its DRA system, the bank had successfully improved the speed and accuracy of its fraud detection process. This effort was entirely a part of a broader trend within the AI in the fintech market, and the outcome was as follows:
- 2 to 4x increased financial fraud detection via machine learning
- Transaction analysis time got reduced to days, from weeks, boosting responsiveness
- Enhanced detection precision and better data for law enforcement
Thus, by incorporating AI solutions in the fintech industry, HSBC is improving its security infrastructure and setting the benchmark for the entire fintech industry. [Source: HSBC]
Financial Forecasting
Financial forecasting is among the most prominent use cases of fintech AI in the financial services sector. Earlier, financial forecasting entirely relied on manual analysis and historical financial statements. AI advances this process via ML models capable of analyzing market trends, social sentiment, economic indicators, and consumer behavior analysis.
These ML models continuously adapt to new information and generate patterns to reveal more accurate and dynamic predictions. ML models like Deep Learning Algorithms, Regression Models, etc., process sequential data and predict future outcomes more precisely. This prediction by artificial intelligence in the fintech industry helps businesses optimize their budgets and make informed investments.
Real Example: Goldman Sachs
In 2024, Goldman Sachs launched a three-year program to improve its organizational footprint, better manage compensation expenses, and increase automation through AI in finance. Many of its employees use generative AI tools across their various business units to forecast market predictions.
In this case, the use of generative AI in the fintech market typically revolves around tools like Developer Copilot, GS AI natural-language assistant, etc. The company plans to expand its AI integration through 2025 to improve productivity and client experience. [Source: Markets Media Group]
Climate Finance & Investment Tracking
Climate finance and investment tracking are among the emerging use cases of AI in the finance industry. With today’s shift towards sustainability initiatives, many financial firms are under pressure to align their portfolios, keeping sustainability in mind. But tracking climate risks and green investments is challenging for them. This is where artificial intelligence in fintech becomes important. ML and NLP (Natural Language Processing) analyse vast datasets related to climate finance and then evaluate whether the institution’s operations align with net-zero goals.
Real Example: BNP Paribas
BNP Paribas is actively deploying AI applications in finance and the energy sector to drive efficiency and enhance data quality. With 70% of surveyed institutions viewing inconsistent ESG data as a major barrier, BNP Paribas uses NLP and LLMs to estimate critical emissions data.
Furthermore, BNP Paribas is also preparing to harness fintech AI to harness advanced analytics for risk assessment and regulatory compliance. Thus, the firm uses AI as a game changer in driving actionable climate strategies. [Source: CIB]
Challenges of AI in the Fintech Industry
AI and fintech can go hand in hand, offering considerable benefits. However, it also presents a myriad of challenges. The first challenge of implementing AI applications in fintech is data privacy and security concerns. AI and ML algorithms require large datasets to process information for accurate predictions. This aspect raises significant issues regarding the protection of personal information. Other than this, there are other Challenges of AI in fintech as well:
Regulatory Compliance
Regulatory compliance is a major obstacle, particularly for AI fintech companies with complex infrastructure. The financial sector is among the most heavily regulated industries, and incorporating AI within the sector is not easy. Regulations are two steps behind the advancements AI is making, making it difficult for fintech firms to ensure that their AI models adhere to all applicable laws and regulations.
Also Read: Guide to Financial Regulatory Compliance Software Development
Bias and Fairness
Since the incorporation of AI and machine learning in fintech, data processing has been quite helpful for predictive systems. Among the most impactful AI applications in fintech are those related to loan approvals and fraud prevention. However, since these models learn from historical data, any potential biases present in the data can be very much perpetuated or amplified. This aspect can be critical in applications like loan approvals, fraud detection, etc., where biased decisions can lead to discriminatory outcomes.
High Implementation Costs
Implementing AI in fintech has a significant barrier: high implementation costs. This barrier affects smaller firms or traditional banks with limited digital infrastructure. In addition, developing, training, and regularly maintaining AI systems requires extensive financial and human resources, which is sometimes impossible for smaller financial institutions.
Potential Solutions to the Above Roadblocks
To overcome the AI challenges mentioned in fintech, a multifaceted approach is required, balancing user trust, ethics, and regulations. Implementing robust data governance frameworks can overcome the first challenge of data privacy and security. Data processing techniques can be implemented for encryption, secure data storage, and anonymization.
Furthermore, adherence to data protection regulations such as GDPR compliance and CCPA can also help ensure safe handling of sensitive information. Other solutions are listed below.
- To tackle regulatory compliance, fintech companies should align their work closely with legal experts and regulatory bodies to stay updated with evolving laws and standards. One solution is to invest in RegTech to automate compliance checks and documentations.
- Regular audits and third-party verification of AI models in use to monitor fairness and data bias can significantly help detect bias and mitigate biases early on. In general, Fintech companies should adopt fairness metrics and explainability tools to address this issue.
- When concerns revolve around significant investment in high implementation costs, companies should begin with pilot projects that first address high-impact areas like fraud detection and customer service chatbots. Later, implementation can also spread to leveraging open-source AI frameworks and cloud-based AI-as-a-service with specialized vendors to keep costs in check.
How Appinventiv Can Help in AI Implementation for Your Fintech Firm
Appinventiv, a renowned name in leading digital transformation and AI development, is well-positioned to help fintech firms leverage AI solutions for their unique needs. With cutting-edge experience in integrating AI into core business processes, such as:
- Streamlining operations
- Customer service enhancements
- Regulatory compliance assurance
We identify the most valuable use cases of AI for fintech, be it fraud detection, credit risk analysis, or customer service automation. Our AI development services are our standout function in today’s digital infrastructure. Our firm is well known for using advanced machine learning frameworks to build intelligent systems for transaction monitoring, credit scoring, robo-advisory platforms, and more for fintech firms.
Furthermore, to overcome the challenge of legacy system integration, Appinventiv also offers scalable API architectures to connect modern AI solutions with traditional financial systems. As a custom fintech software development company, our first-class cloud-native approach, combined with our services, ensures that fintech institutions can effortlessly scale their services without compromising performance or security.
We serve as a comprehensive end-to-end partner for financial institutions looking to leverage AI. Through our strategic combination of technical expertise and understanding of the regulatory framework, we deliver AI solutions that are not only innovative but also practical and scalable.
FAQs
Q. How is AI used in fintech?
A. Artificial intelligence (AI) in financial services automates and personalizes financial services, thereby enhancing efficiency and user experience. AI in the fintech industry is used in fraud detection/prevention, credit scoring and risk assessment analysis, customer service automation via AI-powered chatbots, personalized financial recommendations, and predictive analysis for lending and insurance.
Q. How is AI transforming wealth management in fintech?
A. For fintech firms, AI is becoming a useful tool for wealth management. It assists in making personalized recommendation strategies and financial planning more stable, accessible, and data-driven. Traditionally, wealth management was something only reserved for high-net-worth individuals. However, AI-powered recommendations have democratized this space by offering automated portfolio management based on users’ goals, risk tolerance, and market analysis.
Q. What role does AI play in the future of fintech?
A. AI is all set to play a transformative role in shaping the future of how fintech companies operate and deal with the available data. With the rise in the digital age, customers’ expectations have risen, and fintech firms must adapt to this rapidly changing digital landscape seamlessly. To tackle this, AI will become a key part of these firms, allowing them to tailor their products and services based on transactional data and real-time user behavior. This is one of the many benefits of AI in fintech.
Q. What is AI Consulting in fintech?
A. AI consultants within the fintech sector tend to process the business’s unique needs, are responsible for designing tailored solutions, and then guide the implementation of AI tools in the best possible way. AI consulting in fintech refers to the advisory services these experts provide to help financial institutions and companies integrate AI into their solutions.
Q. What are the Challenges of AI in fintech?
A. These challenges typically involve data privacy, regulatory compliance, algorithm-based biases, and subsequent implementation costs. These models heavily rely on large datasets based on historical data and sensitive financial information. Frameworks revolving around regulations are a complex task, and AI models lag behind due to the complex infrastructure of these regulations. In addition, deploying and maintaining AI infrastructure is also a difficult task.
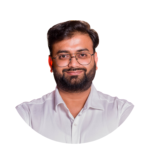
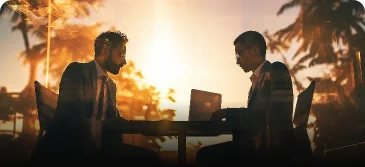
- In just 2 mins you will get a response
- Your idea is 100% protected by our Non Disclosure Agreement.
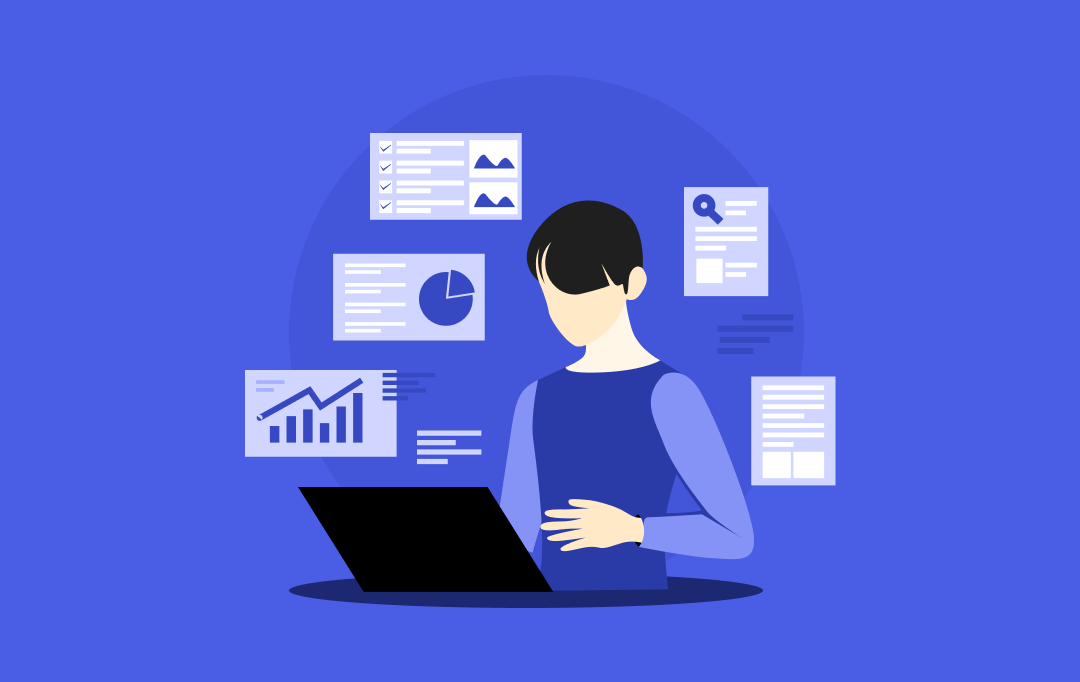
How Appinventiv Solved Scalability Challenges for FinTech Platforms with 10M+ Users
Scalability is a crucial element in the FinTech sector as it is experiencing phenomenal growth due to the global population's need for streamlined, on-demand security and instant financial services. With the rise in user bases and transaction volume, FinTech applications must manage the growing demand without compromising performance or security, while also providing a pleasant…
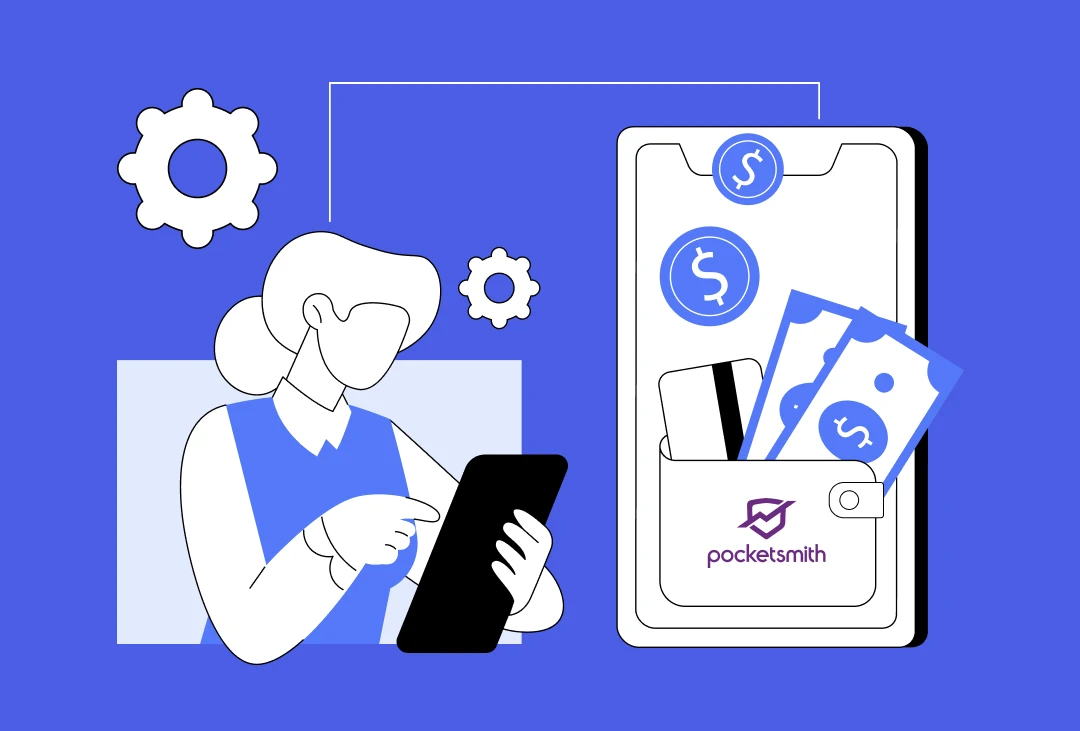
How Much Does it Cost to Build a Personal Finance App like Pocketsmith?
Imagine an app that knows when your rent is due, reminds you of upcoming bills, forecasts your savings 12 months ahead, and tells you if that third coffee this week is wrecking your budget. That’s the kind of experience apps like PocketSmith deliver, and it’s exactly why personal finance apps are becoming a must-have for…
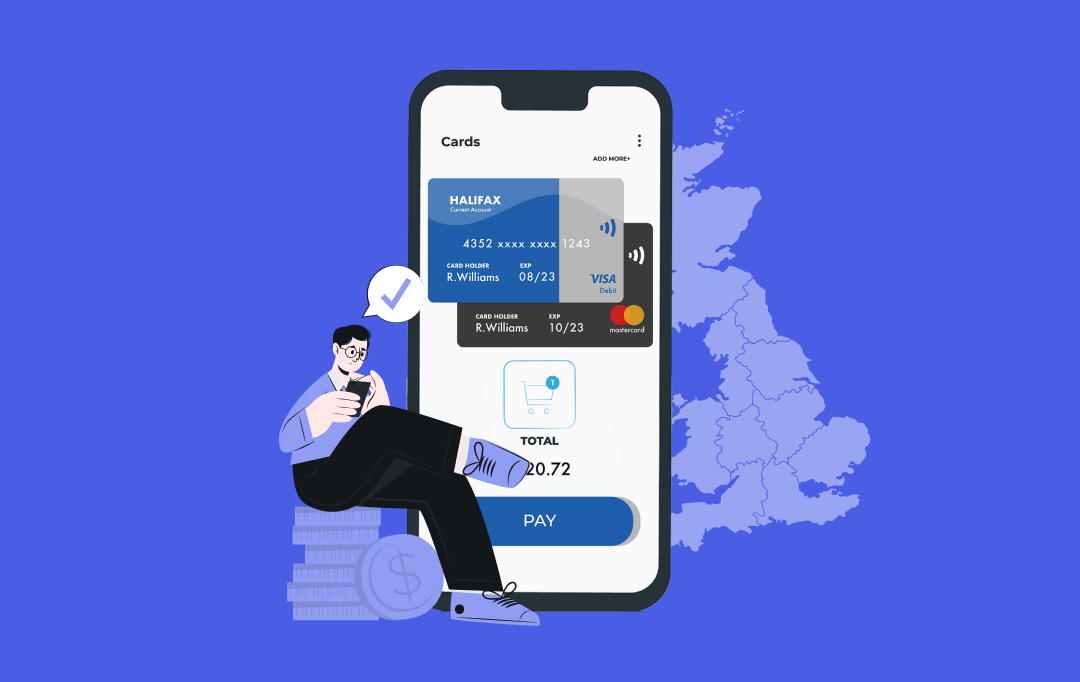
How Much Does it Cost to Build a Mobile Banking App Like Halifax in the UK?
The way people bank has changed dramatically. Gone are the days of waiting in long queues or rushing to a branch before closing hours. Today, mobile banking apps like Halifax have redefined convenience, allowing users to check balances, transfer funds, pay bills, and even apply for loans from their smartphones, anytime and anywhere. This shift…