- Traditional AI Agents vs. Agentic AI Systems in Healthcare
- Technical Overview of Agentic AI in Healthcare
- Perception and Context Awareness
- Decision-Making Under Uncertainty
- Action and Execution Layer
- Continuous Learning
- Key Features of Agentic AI in Healthcare
- Core Functional Domains of Agentic AI in Healthcare
- 1. Clinical Decision Support Systems (CDSS): Data-Driven Decisions
- 2. Diagnosis: Real-time Insights for Accurate Detection
- 3. Treatment and Patient Care: Personalized, Adaptive Care Models
- 4. Patient Engagement and Monitoring: Empowering Patients with Continuous Feedback
- 5. Healthcare Administration and Operations: Streamlining Hospital Management
- 6. Drug Discovery and Research: Accelerating the Development of New Medications
- 7. Robot-Assisted Surgery: Precision at the Cutting Edge
- 8. Chronic Care Agents
- 9. Surgical Planning
- Essentials to Build an AI Agent for Healthcare Industry - Cost, Readiness, and Challenges
- Understanding the Cost to Build AI Agent for Healthcare Industry
- Preparing for Agentic AI in Healthcare - What Founders and Business Leaders Need to Know
- Real-World Challenges of Agentic AI for Healthcare
- Building the Future of Healthcare, One Agent at a Time
- FAQs
- Q. How is Agentic AI being used in healthcare?
- Q. What are some real-world applications of Agentic AI in healthcare?
- Q. How does Agentic AI improve patient outcomes in healthcare?
Key Takeaways
- Agentic AI goes beyond traditional AI by autonomously initiating, planning, and executing tasks, acting more like a digital teammate.
- It transforms core healthcare functions, such as real-time diagnostics, personalized treatment, robotic surgeries, drug discovery, etc.
- Adoption requires readiness. From clean data to regulatory compliance, healthcare providers must prepare holistically to deploy agentic AI at scale.
- Implementation costs vary from $50K to $300K for basic task-specific agents to $1M+ for enterprise-grade systems.
Imagine walking into a hospital where systems don’t just process information – they make decisions, anticipate needs, and take action on behalf of both patients and providers. Not in a decade. Not in theory. But now.
This isn’t the latest plot twist in a sci-fi series – it’s the emerging reality powered by Agentic AI in healthcare. While traditional AI has played a critical role in diagnostics, imaging, and data crunching, Agentic AI takes things several steps further. It doesn’t just respond, it initiates. It doesn’t just support, it collaborates, plans, and adapts, like a digital colleague embedded within the healthcare workflow.
The healthcare industry, long burdened by administrative overload, fragmented systems, and rising patient expectations, is beginning to see what happens when intelligent agents are given to act. And the transformation is anything but subtle.
In this article, we’ll explore how Agentic AI healthcare is reshaping clinical operations, patient engagement, and care delivery – introducing an era where intelligent automation doesn’t just optimize healthcare, it redefines it.
Traditional AI Agents vs. Agentic AI Systems in Healthcare
To really grasp the impact Agentic AI is having on healthcare, it would help to take a step back and compare it with what we’ve known so far.
Traditional AI agents have been around for a while now, and they’ve made a meaningful dent in healthcare, there’s no denying that. Tools that analyze medical images for early signs of disease, chatbots that help patients book appointments, systems that suggest treatment options based on large datasets – all of these are powered by traditional AI.
But their roles are usually limited to reactive tasks. They wait for input. They respond based on what they’ve been trained on. They’re impressive – but they’re not exactly thinking ahead or connecting the dots on their own.
These systems are excellent at pattern recognition and classification tasks. Feed them data, and they’ll give you results. But they won’t decide what to do with those results, they won’t coordinate with other systems. And they definitely won’t re-evaluate their approach if conditions change.
Healthcare for AI agentic, on the other hand, operates on a very different level. These are goal-driven systems that combine reasoning, planning, and adaptive decision-making to carry out complex, often multi-step tasks, sometimes entirely autonomously.
Let’s say a hospital wants to reduce avoidable re-admissions for patients with congestive heart failure. A traditional AI might analyze patient histories and generate a risk score. Helpful? Sure. But it stops there.
Agentic AI systems in healthcare would start with that risk score, and then move forward. It could:
- Coordinate automated follow-up calls or messages
- Schedule home health nurse visits based on availability and patient location
- Update the EHR in real-time
- Alert clinicians to deviations in vital signs
- Even rework care plans dynamically based on patient adherence or recovery progress
That’s not just analytics – it’s agency. AI agents for healthcare are a system that understands a high-level objective and executes a series of intelligent actions to achieve it, involving both humans and machines as needed.
Another major difference is in how these systems evolve. Traditional AI/ML models often need periodic retraining with new data, and they typically operate within the boundaries of their training set. Agentic AI systems, however, are designed to learn from interaction and adapt their behavior – kind of like a junior care coordinator who quickly learns the ropes and gets better with every shift.
In essence:
- Traditional AI answers questions; Agentic AI asks, “What should I do next?”
- Traditional AI supports human action; Agentic AI can initiate and orchestrate that action
- Traditional AI is tool-like; Agentic AI is teammate-like
And this distinction isn’t just theoretical, it has massive implications for patient care, clinical efficiency, and system-level transformation. When you build an AI agent for the healthcare industry it doesn’t just help humans make better decisions; it starts to take on decisions and workflows that were previously bottlenecked by bandwidth or complexity.
Technical Overview of Agentic AI in Healthcare
Unlike traditional AI that focuses on narrow tasks, agentic AI in healthcare is designed to function as an autonomous decision-maker within complex healthcare environments. It perceives context, weighs multiple variables, adapts in real time, and takes action, much like a skilled human agent would. Let’s unravel how autonomous agents work behind the curtains:
Perception and Context Awareness
The system begins by ingesting data from multiple sources: EHRs, medical devices, diagnostics, and even wearables. It doesn’t just collect this information; it interprets it in real time. This deep contextual awareness allows the AI to spot subtle patterns, respond to shifting patient conditions, and stay continuously updated as new data flows in.
This lays the foundation for adaptability – the system isn’t bound to static workflows. If a patient’s vitals suddenly change or lab results deviate from expectations, the agentic AI for healthcare adjusts its recommendations or actions accordingly.
Decision-Making Under Uncertainty
At its core, agentic AI healthcare blends deterministic logic with probabilistic models. Instead of rigid rule-following, it calculates possible outcomes, weighs risks, and makes decisions even when data is incomplete or ambiguous. That means it can, for example, assess whether a deteriorating patient needs immediate escalation, even before a nurse arrives.
Here’s where autonomy and intelligent reasoning come into play, healthcare for AI agentic doesn’t just assist, it acts. And it does so with built-in accountability by assigning confidence levels to its decisions.
Action and Execution Layer
Once a decision is made, the agentic AI systems in healthcare can act – automatically triggering alerts, modifying care plans, or initiating workflows across connected systems. This closes the loop between sensing and doing, allowing real-time, meaningful intervention without waiting for human input. This is not just passive analysis, it’s active, hands-on participation in care delivery.
Continuous Learning
What sets AI agents for healthcare apart is its ability to learn from outcomes. Every action and its result feeds back into the system to refine future responses. If a specific dosage adjustment improves patient recovery in certain conditions, the system remembers and applies that insight going forward.
Key Features of Agentic AI in Healthcare
- Autonomy
It doesn’t need to be hand-held. The system can detect, decide, and act, handling routine tasks or urgent interventions on its own, freeing up human clinicians for higher-level decisions. - Adaptability
Patient conditions are fluid. Agentic AI evolves with them, adjusting to new information and modifying plans in real time. - Scalability
Designed to grow with your infrastructure, the features of agentic AI in healthcare handles rising data volumes and system complexity without added manual effort. - Probabilistic Decision-Making
Instead of rigid yes/no logic, it calculates probabilities – offering confidence scores and nuanced choices, especially useful when the stakes are high but data is messy.
Core Functional Domains of Agentic AI in Healthcare
The benefits of agentic AI in healthcare are making a transformative impact across multiple aspects of healthcare, empowering providers, researchers, and patients with systems that are not only intelligent but also adaptive, autonomous, and predictive. These domains represent areas where the technology is revolutionizing the landscape, offering personalized, proactive, and precise care.
Let’s explore these core areas that make the efforts to build an AI agent for the healthcare industry fruitful.
1. Clinical Decision Support Systems (CDSS): Data-Driven Decisions
Beyond suggestions, CDSS agents of tomorrow won’t just “recommend”, they’ll simulate. Rather than scoring medications by guidelines, they’ll visualize treatment paths as branching timelines tailored to the patient’s physiology, genomics, and environment.
Use Case: PathSim Agent for Dynamic Outcome Forecasting
Say a patient’s been diagnosed with a rare autoimmune disorder. Instead of choosing based on precedent, agentic AI would run thousands of real-time simulations using synthetic biology models of the patient’s immune system, microbiome, and drug-response curves.
It shows: Day 0 to Month 6, here’s what happens if we go with Drug A, B, or CRISPR editing – side effects, hospitalizations, even socioeconomic impacts. The physician doesn’t just treat; they time-travel through the possibilities.
2. Diagnosis: Real-time Insights for Accurate Detection
One of the most significant agentic AI use cases in healthcare is the improvement of diagnostic accuracy. Traditional diagnostic processes, while thorough, can be prone to human error or delays, especially when dealing with complex conditions. Agentic AI, with its ability to autonomously analyze large datasets, ranging from medical imaging to genetic data, significantly enhances the accuracy and speed of diagnoses.
Use Case: Early Disease Detection with Imaging
For instance, AI systems such as Zebra Medical Vision have developed algorithms that can analyze medical imaging to detect early signs of diseases like cancer, cardiovascular conditions, and neurological disorders. These AI systems are trained on millions of imaging scans, learning to spot patterns and anomalies that are sometimes too subtle for the human eye to catch. With this early detection capability, clinicians can intervene much sooner, leading to better patient outcomes and lower treatment costs.
In a groundbreaking collaboration, Google Health is leveraging AI to enhance mammography interpretation. In a study, Google’s deep learning model outperformed human radiologists in breast cancer detection, demonstrating a significant reduction in false positives and false negatives. This not only helps in providing a quicker, more accurate diagnosis but also improves patient confidence and reduces unnecessary procedures.
Also Read: How AI Expedites the Medical Diagnosis Process
3. Treatment and Patient Care: Personalized, Adaptive Care Models
Agentic AI applications in healthcare specially in treatment and patient care are immense, shifting healthcare from a one-size-fits-all model to a personalized, dynamic system where interventions can be adapted in real time based on the patient’s evolving condition. This results in more effective treatments and reduced healthcare costs.
Use Case: AI in Remote Monitoring and Chronic Disease Management
For chronic diseases such as diabetes, hypertension, and asthma, continuous monitoring is critical. Examples of agentic AI in healthcare like Livongo Health enable real-time tracking of vital health metrics, such as glucose levels and blood pressure. These systems analyze the data to provide actionable insights and trigger interventions, such as adjusting medication or suggesting lifestyle changes. For example, if a diabetic patient’s blood sugar readings become dangerously high, the system automatically recommends corrective measures or alerts the healthcare provider.
Livongo’s AI-driven platform empowers patients to manage their conditions proactively, reducing hospital readmissions and improving quality of life. By automating routine monitoring and providing real-time feedback, it allows patients to make data-driven decisions in managing their health.
4. Patient Engagement and Monitoring: Empowering Patients with Continuous Feedback
Instead of reacting to fluctuations in heart rate or oxygen levels, future agents will actively manage physiological states like inflammation, cortisol levels, or neurotransmitter imbalances in real time, tuning the patient toward optimal states like a pilot stabilizing a plane.
Use Case: Autonomic Balance Orchestrator
For ICU patients, an agent would adjust not just ventilator pressure or IV dosage but use neuromodulatory feedback loops (e.g. via optogenetics or targeted vagus nerve signals) to maintain calm, reduce cytokine storms, and synchronize circadian cycles. It’s not “alert fatigue” – it’s “alert orchestration.” Such agentic AI systems in healthcare have the tendency to become the silent conductor of biological harmony.
5. Healthcare Administration and Operations: Streamlining Hospital Management
AI’s role in healthcare administration cannot be overstated. From optimizing hospital workflows to enhancing patient scheduling, agentic AI systems in healthcare are streamlining operations and improving the efficiency of healthcare providers. This reduces administrative burdens, allowing staff to focus more on direct patient care.
Use Case: AI for Predictive Resource Management
Hospitals face the challenge of managing scarce resources like ICU beds, ventilators, and staff. Benefits of agentic AI in healthcare can be gauged through AI systems like Qventus which are helping healthcare providers optimize hospital operations by predicting patient volume, staff needs, and resource allocation. By analyzing historical data, Qventus’ AI can forecast surges in patient admissions, enabling hospitals to proactively allocate resources and manage staff workload, ensuring that critical resources are always available when needed.
During the COVID-19 pandemic, such healthcare for AI agentic systems were instrumental in helping hospitals adjust to rapidly changing patient volumes, ensuring that hospitals could provide timely care even during peak times.
Also Read: Hospital Management System Development – Benefits, Process, Features, Costs
6. Drug Discovery and Research: Accelerating the Development of New Medications
Traditionally a long and expensive journey, the drug discovery process is now being transformed by artificial intelligence, particularly, agentic AI. By analyzing massive datasets, AI can identify potential drug candidates, predict their effectiveness, and even optimize clinical trial designs.
Use Case: AI in Drug Development for Rare Diseases
Transcripta Bio, a pharmaceutical startup, utilizes AI to match existing medications with new use cases, focusing on rare diseases. Their Drug-Gene Atlas database enables the identification of new applications for existing drugs, and they have successfully repositioned five FDA-approved drugs for new indications within two years.
Moreover, AI agents for healthcare can analyze data from thousands of clinical trials to identify patterns, providing new insights into drug efficacy and side effects. This reduces trial-and-error, ultimately speeding up the development of life-saving drugs.
7. Robot-Assisted Surgery: Precision at the Cutting Edge
Robot-assisted surgery, empowered by agentic AI healthcare, is revolutionizing surgery by providing higher precision, reduced recovery times, and enhanced patient outcomes. With AI, robotic surgical systems can analyze patient data in real time and adjust surgical techniques based on the patient’s specific anatomy.
Use Case: Minimally Invasive Surgery with AI
The da Vinci Surgical System by Intuitive Surgical is one of the most advanced examples of AI-powered robotic surgery. Surgeons use the robotic system to perform complex procedures with greater precision and smaller incisions, which leads to faster recovery times and less postoperative pain. The system’s AI continually monitors and adjusts the instruments during surgery, ensuring that the surgery is as precise as possible.
Recent studies have shown that robotic-assisted surgeries can reduce complications and improve long-term patient outcomes. The da Vinci system’s real-time feedback allows surgeons to refine their techniques during operations, leading to higher success rates in surgeries such as prostatectomies and heart valve repairs.
8. Chronic Care Agents
Forget static dashboards or alerts. Agentic AI in chronic care will become a persistent presence, growing as your physiology changes, your life context shifts, and treatments evolve.
Use Case: EpiAgent for Life-Stage-Aware Care
Imagine a teen diagnosed with juvenile arthritis. Instead of managing medications reactively, the agent evolves over decades. It adapts dosing strategies when the user hits puberty, shifts care planning during pregnancy, alters goals during menopause, and communicates lifestyle trade-offs based on socioeconomic changes. Not just a “care plan” – a lifelong co-evolving AI caregiver.
9. Surgical Planning
Agentic AI won’t stop at planning incisions. It will design the post-op future first – and then work backward.
Use Case: Recovery-First Surgery Composer
Before a knee replacement, the agent models recovery trajectories based on the patient’s gait, muscle density, pain thresholds, and home setup. It tells the surgeon: “Make a 2cm medial cut, rotate 3° inward, she’ll walk unassisted in 21 days.” The agent designs the cut not for now, but for how you want to feel later.
Agentic AI is pushing the boundaries of what’s possible in the sector. From diagnosing diseases faster and more accurately to creating personalized treatment plans that evolve with the patient, AI is transforming the industry. These benefits of agentic AI in healthcare are not only improving the precision of care but also making healthcare more efficient, affordable, and accessible.
Let’s explore how we can tailor these capabilities.
Essentials to Build an AI Agent for Healthcare Industry – Cost, Readiness, and Challenges
Adopting Agentic AI for healthcare isn’t just about embracing the next big trend – it’s about reshaping how decisions are made, patients are treated, and care systems evolve over time. But for those considering the leap, three big questions often arise:
What will it cost? Are we ready for it? And what roadblocks should we anticipate? Below, we break down these crucial areas – not from a theoretical lens, but from the practical realities of building and deploying intelligent healthcare agents in 2025 and beyond.
Understanding the Cost to Build AI Agent for Healthcare Industry
The cost of implementing agentic AI healthcare depends on how advanced and autonomous you want your systems to be. It’s not a one-size-fits-all model – and that’s exactly what makes pricing both flexible and nuanced.
For example, if you’re starting small with task-specific agents – say, automating follow-ups or basic patient routing – you might be looking at $50K–$300K. These systems often rely on existing APIs, pretrained models, and minimal customization.
But when you move into more intelligent territory, such as clinical decision support, personalized recommendations, or adaptive care assistants – the cost can scale to $300K–$800K, especially as integration, data complexity, and compliance checks increase.
Now, if you’re building enterprise-grade, closed-loop systems – agents that can analyze diagnostics, respond to patient feedback in real-time, or even assist in clinical research – then you’re looking at $1M+. These solutions require robust architecture, multimodal reasoning, custom agent workflows, and often, their own governance and risk layers.
These costs to build AI agents for the healthcare industry are increasingly seen as investments – not just in tech, but in long-term operational savings, outcome improvements, and competitive edge.
Preparing for Agentic AI in Healthcare – What Founders and Business Leaders Need to Know
AI agents for healthcare don’t just plug into existing systems – they evolve alongside them. Which means your organization has to be ready at multiple levels, something that your partnered healthcare application development services provider will be able to help with.
First, take a look at your data maturity. Are your clinical and operational datasets structured, clean, and interoperable? If your data is siloed or inconsistent, your agents won’t perform well, or worse, they might draw inaccurate conclusions.
Second, when building AI agents for healthcare, focus on workflow clarity. Agents need clearly defined outcomes – whether it’s reducing patient wait times, improving diagnostic accuracy, or optimizing surgical schedules. Without a goalpost, the agent’s autonomy becomes noise.
Team readiness is just as important. AI adoption isn’t only technical; it’s cultural. Clinical and ops teams need to understand what the system will do, how it will behave, and where human oversight fits in.
Then there’s infrastructure. Can your organization support high-compute workloads, secure data sharing, and real-time processing? Cloud-native systems and scalable data layers can make a big difference here.
And finally, compliance. You’ll want to think ahead about consent frameworks, patient explainability, and compliance with HIPAA, GDPR, or any region-specific AI regulations.
Real-World Challenges of Agentic AI for Healthcare
Even with capital and motivation in place, you’ll face challenges that are unique to agentic AI for healthcare. Here are the major ones that founders, CTOs, and innovation leaders need to prepare for:
A. Model Availability and Data Privacy
While LLMs and vision models are more accessible than ever, healthcare-specific agentic models remain hard to find – especially ones that can be fine-tuned securely. Accessing high-quality patient data while ensuring privacy and encryption is an ongoing balancing act.
B. Navigating Regulatory Complexity
Healthcare is one of the most regulated sectors globally. Introducing autonomous agents means you must meet not only AI-specific standards but also healthcare-specific compliances around patient safety, data traceability, and decision explainability.
Also Read: How to Make a HIPAA-Compliant App?
C. Workflow Integration
If your agents don’t seamlessly plug into EHR systems, care delivery platforms, or scheduling tools, adoption will stall. Agentic AI healthcare that operate in silos become glorified chatbots, not strategic tools.
D. Infrastructure Constraints
Not every hospital or health startup has access to secure compute, scalable cloud storage, or GPU-backed systems. If your environment isn’t ready, even the smartest agent won’t work well.
E. Adversarial Threats
Agentic systems are vulnerable to manipulation, especially when connected to external data sources or user inputs. Defensive architectures, threat detection, and reinforcement learning with safety checks are key.
F. Ethical and Legal Responsibility
When agentic AI systems make or recommend life-impacting decisions, who’s responsible? Establishing human-in-the-loop control, audit trails, and accountability structures is a non-negotiable.
G. Human Oversight and Governance
Agentic systems require a governance layer that keeps autonomy in check. Define clear roles for human intervention, override protocols, and feedback loops that evolve with the system.
H. A New Standard for Responsible AI
Ultimately, building AI responsibly means designing with clarity, control, and compliance from day one. Transparency, fairness, and traceability shouldn’t be afterthoughts – they should be built into the blueprint.
Building the Future of Healthcare, One Agent at a Time
Agentic AI isn’t just another leap in automation – it’s the reimagining of healthcare as we know it. From self-directed diagnostic engines to AI caregivers that adapt in real time, the horizon of healthcare is shifting fast. But for organizations to tap into this future, they need more than just AI, they need strategic implementation from a skilled custom agentic AI development company who understand tech, healthcare systems, and the evolving regulatory environment.
That’s where Appinventiv comes in.
We don’t just build AI-powered systems. We co-create intelligent agents that think, act, and evolve with your healthcare workflows. Our experience spans full-stack AI product development, cloud infrastructure setup, data engineering, regulatory compliance, and continuous post-deployment optimization.
Whether you’re looking to:
- Design autonomous diagnostic agents for remote care.
- Build HIPAA-compliant adaptive decision support tools, or
- Roll out patient-facing agentic platforms with multilingual, multi-modal capabilities –
we have the expertise to bring your vision to life.
And we’re not just reacting to where healthcare is going, we’re helping shape it. Our R&D teams are actively exploring the future of agentic AI in healthcare:
- Multi-agent collaboration models for hospital ecosystems
- Agentic AI embedded in wearables for real-time intervention
- Federated learning frameworks that preserve patient data privacy
- Context-aware AI that can balance patient-specific needs with systemic efficiency
As a healthcare application development services firm, we see the future of healthcare as not only intelligent, but also personal, transparent, and ethical. Agentic AI is the means – your vision is the goal. Let’s make both real.
FAQs
Q. How is Agentic AI being used in healthcare?
A. Agentic AI is being used to build intelligent systems that can autonomously perceive, reason, and act within clinical environments. Unlike traditional AI models, these agents operate with a high degree of autonomy, enabling them to assist with diagnoses, suggest treatment plans, monitor patient progress, and even coordinate across hospital departments.
They’re also increasingly being embedded in virtual health assistants, diagnostic engines, and workflow optimization tools, bringing real-time intelligence to every layer of care delivery.
Q. What are some real-world applications of Agentic AI in healthcare?
A. Real-world applications of Agentic AI for healthcare include:
- AI diagnostic agents that assess patient records and imaging data to flag early signs of cancer or cardiovascular issues.
- Clinical decision support tools that continuously learn from patient feedback and treatment responses.
- Agentic systems in operating rooms that guide robotic surgeries or anticipate surgeon needs.
- Autonomous patient monitoring tools that adjust alerts and interventions based on evolving vitals and behavioral cues.
- Intelligent virtual care agents that engage with patients post-discharge, ensuring medication adherence and recovery tracking.
Hospitals and healthtech providers across the US and Europe are already piloting such solutions to reduce readmissions and increase diagnostic accuracy.
Q. How does Agentic AI improve patient outcomes in healthcare?
A. Agentic AI improves patient outcomes by enabling faster, more accurate, and personalized interventions. These systems don’t just follow static rules, they learn and adapt in real time, identifying patterns across massive datasets that human clinicians might miss.
For example, an agentic AI could detect early signs of sepsis hours before symptoms become critical, or fine-tune a cancer treatment regimen based on a patient’s individual response, lifestyle, and history. The result is smarter decisions, quicker care, and higher chances of recovery – all with reduced strain on care teams.
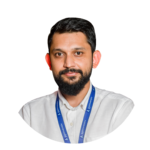
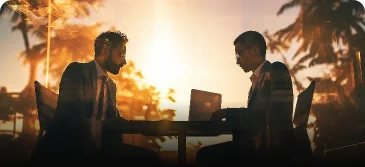
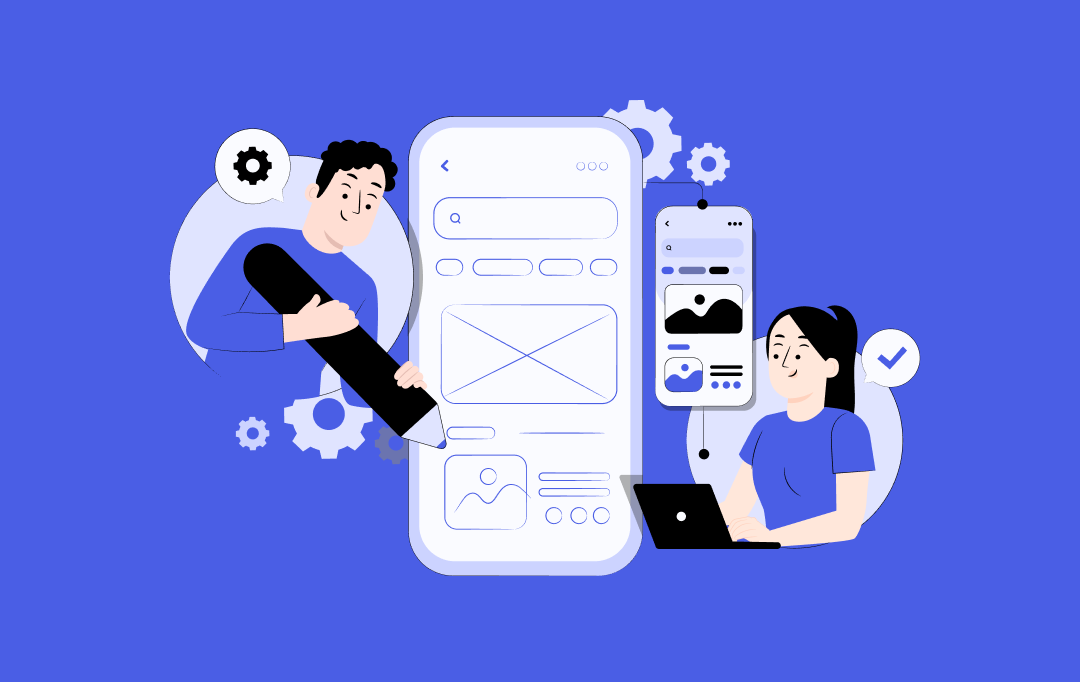
How Much Does It Cost to Build an App like Janitor AI?
Key takeaways: Cost Breakdown: The cost of app development varies, with an MVP App costing $40,000 to $90,000, a Mid-Tier App costing $100,000 to $200,000, and an Enterprise Grade App costing $300,000 to $ 500,000 or more, depending on features, complexity, and the expertise of the development team. Key Factors: Platform choice, AI integration, and…
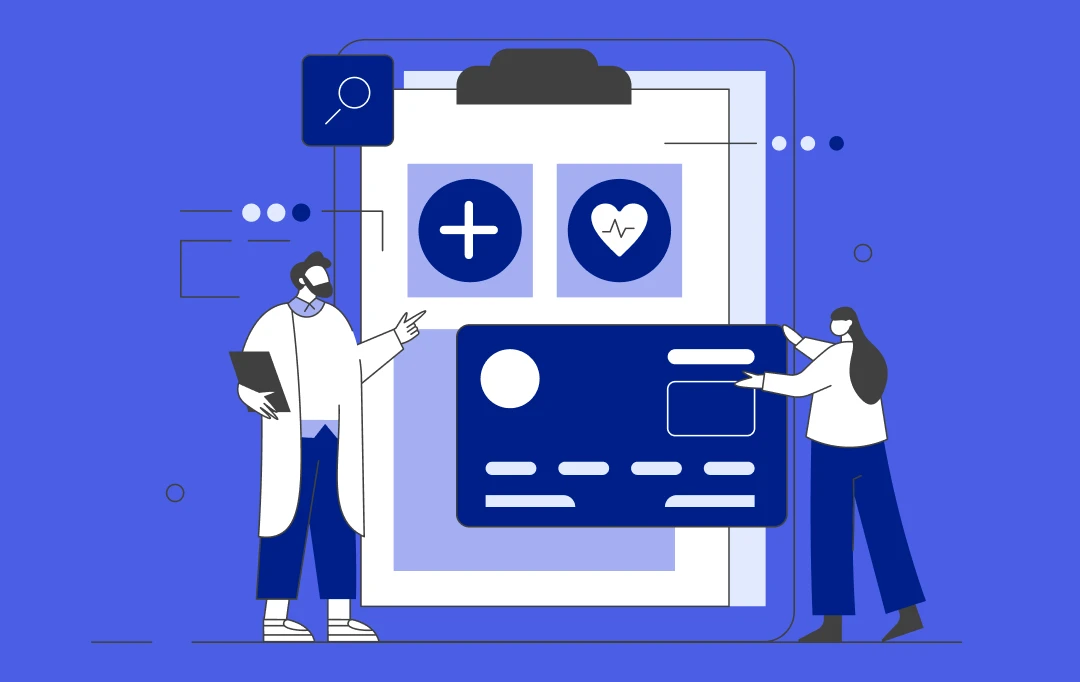
10 Ways AI is Transforming the Healthcare Sector in Australia
Key takeaways: Generative AI could add $13 billion annually to Australia’s healthcare sector by 2030. AI is already transforming operations across diagnostics, admin tasks, and patient care. Healthcare in remote areas is improving with AI-powered virtual assistants and monitoring tools. AI can help address workforce shortages by automating routine tasks and supporting clinical decisions. Major…
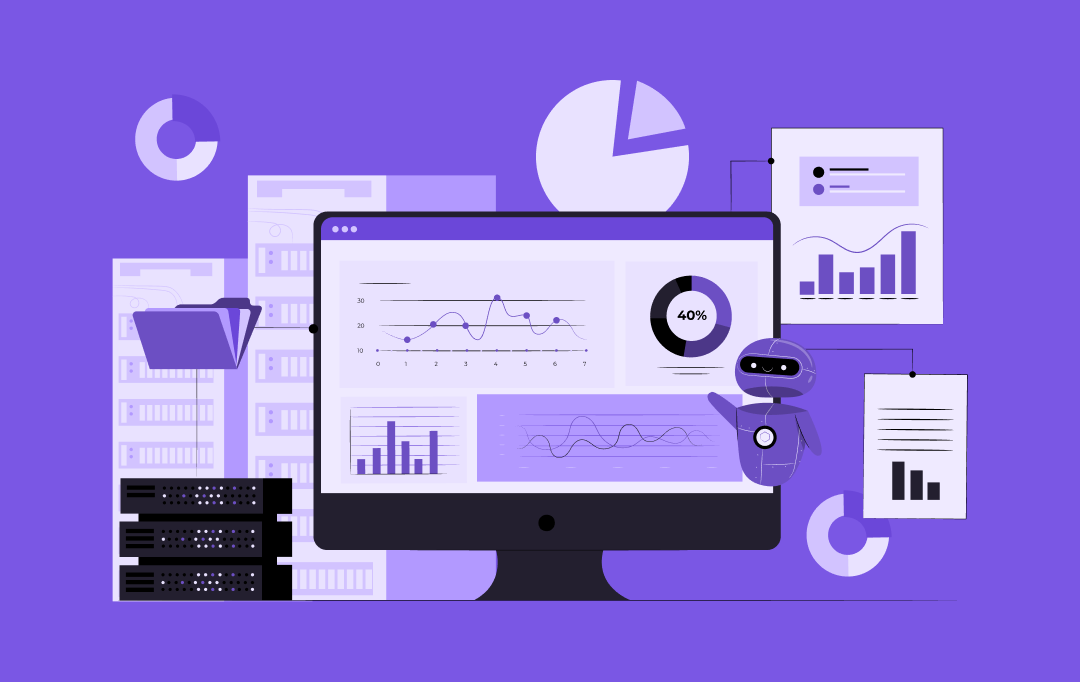
Exploring the Power of AI in Creating Dynamic & Interactive Data Visualizations
Key takeaways: AI transforms data visualization by providing automated insights, interactive dashboards, and NLP, thereby tackling revenue loss and improving data quality. Key features include predictive analytics, real-time data integration, and user-friendly visualizations, enabling faster and more informed decisions. Top 2025 tools, such as Tableau, Power BI, and Julius AI, offer NLP and real-time analytics…