- Role of Artificial Intelligence in Drug Discovery and Development
- Benefits Of AI in Drug Discovery
- Accelerated Drug Development
- More Effective Drugs
- Improved Clinical Trial Design
- Prediction of Drugs' Bioactivity
- Quality Assurance
- Drug Repurposing
- Drug Combination Analysis
- Patient Stratification
- Applications of AI in Drug Discovery
- Target Selection and Validation
- Compound Screening and Lead Optimization
- Preclinical Studies
- Clinical Trials
- How AI for Drug Discovery Monitor Post-market Safety
- Signal Detection
- Real-Time Monitoring
- Risk Prediction
- Drug-Drug Interactions
- Real-World AI Drug Discovery Examples
- Cancer Treatment Compound Discovery
- MEK Protein Inhibitor Identification
- Alzheimer's Disease Therapeutic Targeting
- Novel Antibiotic Discovery
- COVID-19 Therapeutic Research
- Challenges of Using AI in Drug Discovery
- Data Privacy and Regulatory Compliance:
- Data Quality and Quantity:
- Cost and Technical Expertise
- Interpretability and Transparency
- Lack of Standardization
- Future of AI in Drug Discovery
- Conclusion
- FAQ’s
The discovery of new drugs has always been a challenging and expensive process. It involves extensive timeframes and significant costs, which have long highlighted the need for reform in this critical field. Despite previous efforts to accelerate drug discovery through computational methods, progress has remained limited.
Artificial intelligence (AI) has emerged as an exciting solution that shows great potential in revolutionizing AI for drug discovery and development. AI facilitates the identification of optimal drug candidates, provides unprecedented insights into various diseases, and effectively manages extensive patient datasets. These capabilities are driving transformative change across the pharmaceutical landscape.
According to McKinsey, the incorporation of AI capabilities in big data strategies has the potential to generate an annual value of up to $100 billion within the US healthcare system. This includes leveraging predictive modeling and conducting comprehensive analysis of sensor data.
Moreover, a joint research endeavor conducted by Carnegie Mellon University and a well-known German institution has highlighted that AI in drug discovery significantly reduces drug discovery expenses. This collaboration suggests that companies could potentially cut their costs by up to 70%. So, in this article, we will discuss the power of artificial intelligence in drug discovery and development and how these advanced AI techniques revolutionize healthcare.
Role of Artificial Intelligence in Drug Discovery and Development
Artificial intelligence drug discovery research focuses on finding medications that effectively treat specific diseases by positively affecting the body. Researchers traditionally conduct thorough screenings of molecular libraries to identify a molecule that can bind to a target molecule, particularly a protein associated with a specific disease. Following this, these identified molecules undergo multiple rounds of testing to refine them into potential drug candidates.
Recent trends indicate a rising acceptance of rational, structure-based drug design methods. These approaches, while bypassing initial screening stages, still require chemists to undertake the design, synthesis, and evaluation of numerous compounds in order to identify potential new drugs.
The challenge lies in the uncertainty surrounding which chemical structures possess the desired biological effects and necessary properties for effective drug function. As a result, refining a promising compound into a viable drug candidate becomes a resource-intensive and time-consuming process. Current data indicates that bringing a new drug to market now costs around $2.6 billion.
Moreover, even if a new drug shows promise during laboratory testing, it may encounter obstacles throughout the clinical trial phase. Phase I trials for these drug candidates demonstrate that 9.6% of them are successful in reaching the market.
The data processing capabilities of AI systems have gained significant attention due to the challenges faced. Experts predict that AI for drug discovery can accelerate the process and reduce costs, serving as a catalyst. Market research firm Bekryl has projected potential savings, exceeding $70 billion in the drug discovery process by 2028 through the integration of AI.
Benefits Of AI in Drug Discovery
The use of AI in drug discovery presents several key advantages for the pharmaceutical industry:
Accelerated Drug Development
With the introduction of artificial intelligence in drug discovery and development, the process of identifying potential drug targets can be greatly expedited. be employing algorithms powered by machine learning in healthcare, extensive datasets can be swiftly analyzed, resulting in the prompt discovery of potential drug candidates. This acceleration streamlines the lead discovery process, ultimately saving valuable time and resources for researchers and pharmaceutical companies.
More Effective Drugs
Artificial intelligence in drug discovery and development plays an essential role in predicting the pharmacological properties of lead molecules based on their chemical structure, making drug development more effective. Through the use of machine learning algorithms, researchers can create predictive models that estimate key properties like solubility, bioavailability, and toxicity. These models then guide the design of new molecules with improved pharmacological characteristics, boosting the efficiency and safety of potential drug candidates.
Improved Clinical Trial Design
AI plays a significant role in enhancing clinical trial design. By analyzing electronic medical records and patient data, AI streamlines patient recruitment by identifying suitable candidates more efficiently. Moreover, AI helps in optimizing trial designs by identifying patient subgroups that are more likely to respond positively to specific treatments. The utilization of AI-driven wearable devices allows for real-time monitoring, ensuring precise data collection and the necessary adjustments to trial protocols for enhanced patient safety. Additionally, AI algorithms facilitate robust data analysis, offering valuable insights for future research and clinical practices.
Prediction of Drugs’ Bioactivity
Artificial intelligence in drug discovery and development has revolutionized the prediction of drugs’ bioactivity. Researchers now use AI to predict the bioactivity of different compounds using techniques like quantitative structure-activity relationship (QSAR) modeling and molecular docking.
These methods analyze the chemical structure of compounds and their interactions with target proteins, leading to more accurate predictions of their biological activity. Utilizing deep learning techniques, AI uncovers intricate patterns and relationships within vast datasets, enabling precise predictions of the bioactivity of untested compounds.
Quality Assurance
AI for drug discovery plays a crucial role in enhancing the accuracy and efficiency of various quality assurance processes in the domain of drug quality control. By employing computer vision algorithms for automated inspection, AI assists in identifying defects, contamination, and packaging inconsistencies. This ensures that drugs meet strict quality standards.
Furthermore, AI algorithms analyze sensor data from manufacturing equipment, enabling predictive maintenance measures to prevent equipment failure and minimize production downtime. AI also aids in fraud detection by analyzing sales and distribution data to identify suspicious patterns, thereby ensuring the integrity and safety of drug distribution channels.
Drug Repurposing
AI offers a promising approach to discovering new therapeutic applications for existing drugs. This not only significantly reduces the time and costs associated with traditional drug development but also allows for the identification of potential new uses for established medications.
By analyzing extensive datasets of drug and disease information, AI algorithms can unveil patterns and relationships, leading to the exploration of novel therapeutic opportunities. Furthermore, AI and machine learning in pharma enables the investigation of intricate interactions between drugs, targets, and diseases, unlocking further potential for existing medications.
This not only significantly reduces the time and costs associated with traditional AI drug development but also allows for the identification of potential new uses for established medications.
It can accelerate AI drug development, improve clinical trial designs, predict drug bioactivity, and ensure quality assurance.
Drug Combination Analysis
AI’s robust analytical capabilities play a crucial role in the examination of complex diseases that may necessitate the utilization of multiple drugs. By predicting the collaborative effects and determining the optimal dosage for various drug combinations, AI contributes to the development of more efficient treatment strategies.
Furthermore, AI assists in tailoring drug combinations for individual patients by taking into account their genetic and molecular characteristics, ultimately enhancing treatment effectiveness and patient outcomes.
Patient Stratification
AI-powered drug discovery proves itself a valuable tool when it comes to classifying patients. It helps identify specific groups of patients with similar disease profiles and characteristics. Through the utilization of predictive modeling and biomarker identification, AI empowers healthcare providers to personalize treatment approaches, leading to a higher success rate in drug development and ultimately improving patient outcomes.
Applications of AI in Drug Discovery
Here are the some applications of AI in drug discovery that makes processes more simpler than traditional methods.
Target Selection and Validation
AI-powered drug discovery simplifies the process of finding and confirming potential molecular targets by analyzing various datasets, including Drug Information Banks and public libraries. Through the utilization of deep autoencoder, relief algorithms, and binary classification, AI-based drug discovery efficiently prioritizes these targets. Additionally, AI platforms employ graph-convolutional networks and computer vision models trained on cryo-EM microscope data to understand protein structures.
Compound Screening and Lead Optimization
In the field of compound screening, the utilization of AI-driven Virtual Screening enables the efficient identification of potential lead molecules from extensive compound databases. AI Retrosynthesis Pathway Prediction, an automated approach to chemical syntheses, greatly enhances the process of chemical synthesis planning. Moreover, AI-based drug discovery models play a crucial role in cell target classification and facilitate intelligent image-activated cell sorting, resulting in more efficient cell separation.
Preclinical Studies
AI plays a crucial role in the molecular mechanisms of action and predicting dose-response relationships in pharmacokinetic/pharmacodynamic modeling. It effectively streamlines toxicology evaluations through the Deeptox Algorithm, which accurately forecasts compound toxicity. Furthermore, deep learning algorithms utilize transcriptomic data to make precise predictions about pharmacological properties.
Clinical Trials
AI tools hold an essential role in clinical trials, as they enhance several key aspects. AI and drug discovery together contribute to the recognition of patient diseases, identify specific gene targets, and predict molecular effects. Moreover, AI-driven applications improve medication adherence and enable risk-based monitoring, resulting in increased efficiency and success rates for clinical trials.
How AI for Drug Discovery Monitor Post-market Safety
In the field of post-market drug safety monitoring, artificial intelligence (AI) has emerged as a crucial tool. It enables continuous evaluation of drug safety following regulatory approval and widespread patient usage. AI-driven drug discovery plays a significant role in monitoring drug safety after it enters the AI in drug discovery market. This involves carrying out various essential functions, which include:
Signal Detection
AI algorithms play a crucial role in analyzing extensive patient data archives. These algorithms enable the discovery of potential signals that indicate adverse events associated with specific drugs. This capability of AI drug discovery is instrumental in identifying uncommon or unforeseen side effects that may not have been evident during the initial clinical testing phases.
Real-Time Monitoring
AI-driven drug discovery uses data from electronic health records and social media to monitor drug safety in real-time. It swiftly identifies and flags potential safety concerns, allowing for timely interventions to ensure patient safety and well-being.
Risk Prediction
AI algorithms are utilized to predict the probability of adverse events related to a specific medication. These algorithms consider unique patient characteristics and other pertinent factors. By identifying individuals who are at a higher risk of experiencing negative effects, AI enables targeted interventions and personalized medical care, resulting in improved patient safety and treatment outcomes.
Drug-Drug Interactions
Through analyzing intricate patterns and interrelationships among various drugs, AI can forecast potential interactions that could result in unfavorable effects. This capability empowers healthcare providers to make well-informed decisions regarding drug combinations, thereby reducing the risk of detrimental interactions and enhancing patient safety.
Real-World AI Drug Discovery Examples
The AI for drug discovery has seen numerous notable case studies that emphasize the successful incorporation of AI methodologies. Some remarkable AI drug discovery examples include:
Cancer Treatment Compound Discovery
Gupta, R. et al. showcased the potential of AI in discovering new cancer treatment compounds. They employed a Deep Learning (DL) algorithm, which was trained on a vast dataset of known cancer-related compounds, resulting in promising outcomes. By utilizing AI’s capabilities, this approach effectively identifies previously unknown compounds that hold great promise for future therapeutic interventions in cancer research.
MEK Protein Inhibitor Identification
The successful utilization of Machine Learning (ML) in identifying inhibitors for the MEK protein, a crucial target in cancer therapy, has recently been documented. Discovering effective inhibitors for MEK has been a challenging task. However, through the application of ML algorithms, researchers are able to successfully identify novel inhibitors, highlighting the effectiveness of AI drug discovery approaches in addressing complex biomedical hurdles.
Alzheimer’s Disease Therapeutic Targeting
The application of machine learning (ML) algorithms has simplified the discovery of new inhibitors for beta-secretase (BACE1), a key protein implicated in the progression of the disease. This successful incorporation of AI methods has unlocked fresh opportunities for addressing complex neurodegenerative disorders, highlighting the role of AI in advancing therapeutic solutions for intricate health challenges.
Novel Antibiotic Discovery
AI-driven drug discovery has expanded its capabilities to identify new antibiotics. Advanced machine learning techniques have successfully identified potent antibiotic candidates from a vast pool of over 100 million molecules. As a result, a powerful antibiotic has been discovered, proving effective against various drug-resistant bacterial strains, including tuberculosis. This impressive accomplishment emphasizes the pivotal role of AI in combatting critical global health threats.
COVID-19 Therapeutic Research
The ongoing research on combatting COVID-19 has made significant progress by utilizing ML algorithms. Through the analysis of extensive datasets, AI in drug development has played a crucial role in identifying specific compounds for treating the virus. This particular use case demonstrates the agility and adaptability of AI in responding to emerging global health crises, confirming its irreplaceable position in contemporary drug discovery efforts.
With our top-notch healthcare software development services, we have impacted the lives of patients everywhere.
Challenges of Using AI in Drug Discovery
AI holds great potential to revolutionize drug discovery. However, its widespread integration is hindered by critical challenges that disrupt seamless implementation.
Data Privacy and Regulatory Compliance:
The sensitive nature of patient data gives rise to concerns surrounding data privacy and regulatory compliance. It is crucial to adhere to strict data protection regulations, including the US Health Insurance Portability and Accountability Act (HIPAA) and the European Union’s General Data Protection Regulation (GDPR), to address ethical and legal considerations in AI-driven drug discovery.
Data Quality and Quantity:
AI’s effectiveness greatly depends on the availability of high-quality data. In drug discovery, however, the data landscape often poses a challenge. It is marked by the scarcity of data, its diverse nature, and the varying quality it possesses. Such characteristics pose difficulties for AI systems in accurately analyzing and modeling this data.
Cost and Technical Expertise
Implementing AI in drug discovery requires significant investments in terms of finances and technical expertise. The process involves establishing and maintaining the necessary infrastructure while actively recruiting skilled data scientists and AI experts. However, these requirements pose a substantial commitment, creating a challenge for widespread adoption.
Interpretability and Transparency
The complexity of AI models often presents challenges in terms of interpretability and transparency. To establish trust and confidence, it becomes crucial to understand the underlying mechanisms and decision-making processes of these models. This understanding further promotes the broader adoption of AI in drug discovery.
Lack of Standardization
The field of drug discovery faces a significant challenge due to the absence of standardized data formats, collection methodologies, and analysis techniques. This lack of standardization makes it difficult to compare studies and datasets effectively. Consequently, AI encounters obstacles in generating consistent and reliable predictions and models.
Future of AI in Drug Discovery
The pharmaceutical industry is increasingly embracing the integration of AI solutions to alleviate the significant financial burden and potential setbacks associated with traditional Virtual Screening (VS) methods. This shift in approach is demonstrated by the remarkable growth of the AI market, which skyrocketed from $200 million in 2015 to $700 million in 2018. Projections indicate a further surge to $5 billion by 2024, highlighting AI’s transformative potential in reshaping the pharmaceutical and medical sectors. This anticipated 40% growth from 2017 to 2024 highlights the profound impact of AI on these domains.
Conclusion
The merger of AI and drug development has the potential to revolutionize both the pharmaceutical industry and the healthcare sector. It can accelerate drug development, improve clinical trial designs, predict drug bioactivity, and ensure quality assurance. Being a cutting-edge AI development company, we deliver faster, cost-effective, and efficient solutions catalyzing advances in healthcare and the development of life-saving treatments.
If you want to develop your healthcare software development with advanced AI techniques, contact Appinventiv. Our team of experts is dedicated to delivering customized healthcare AI consulting solutions that revolutionize the healthcare industry. Start your journey with us!
FAQ’s
Q. How artificial intelligence is changing drug discovery?
A. AI is changing drug discovery by expediting the search for potential medications. Also, it’s making personalized treatments more feasible and even making it easier for us to find new uses for existing drugs.
Q. How is AI used in drug discovery?
A. AI and drug discovery are employed together in several ways Firstly, AI scans through libraries of chemicals and predicts how different compounds would react with proteins. It also examines individual patient data to develop personalized treatments. Moreover, AI works to streamline clinical trials, making the whole research process more efficient.
Q. How does AI impact the cost and timeline of drug discovery?
A. Artificial intelligence can make the process of discovering new drugs reasonable by improving the way we select compounds and set up clinical trials. This could save an impressive amount in research and development costs. By speeding up the identification of potential medicine candidates and making the clinical trial process more efficient, AI in drug discovery can also help get new treatments into the hands of patients faster.
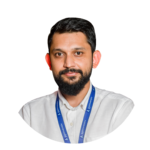
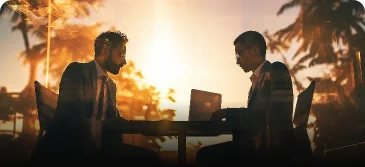
- In just 2 mins you will get a response
- Your idea is 100% protected by our Non Disclosure Agreement.
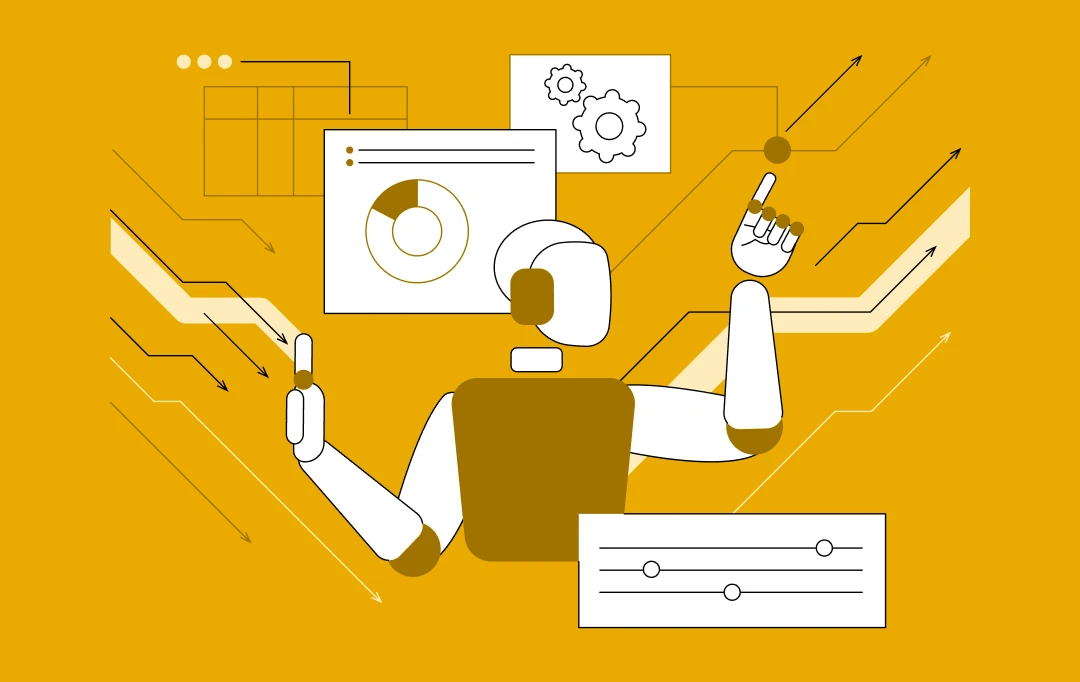
How to Integrate AI with EHR/EMR Systems for Streamlined Healthcare Operations
Key takeaways: Not adopting AI in EHR/EMR today risks falling behind. AI streamlines EHR workflows, cutting clinical and admin delays. Real-world hospitals are already seeing faster, smarter care with AI. Seamless AI integration needs system checks, data prep, and training. AI in EHR tackles real challenges like bias, cost, and compliance. Custom AI integration costs…
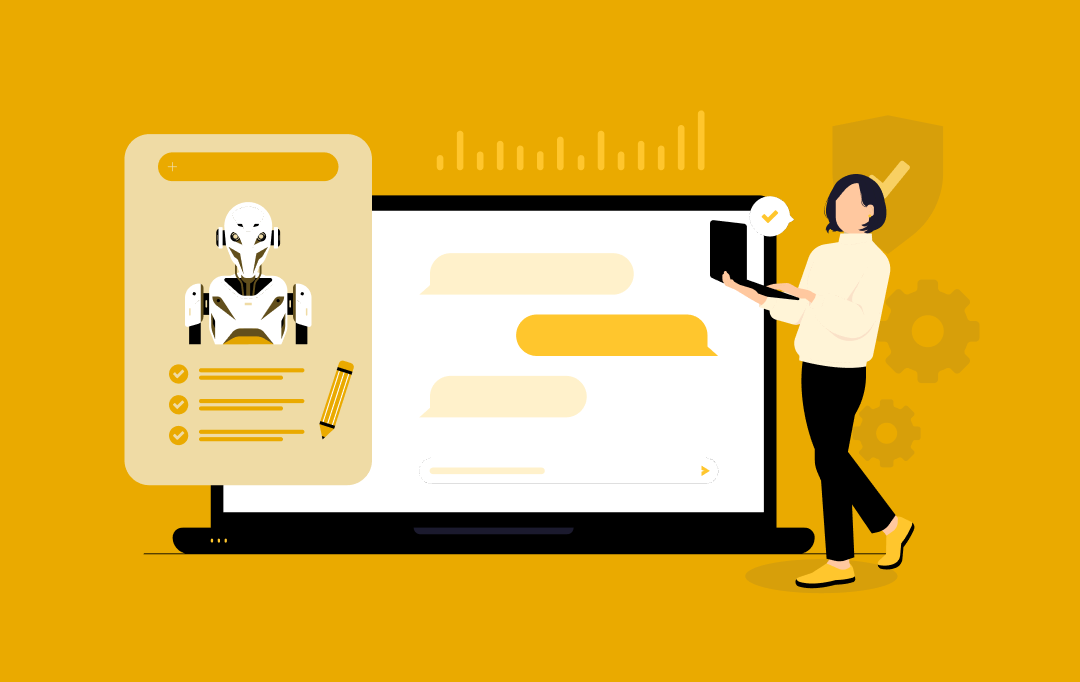
AI Agent-Driven UIs: Replacing App Menus and Buttons
Key takeaways: Challenge: Static menus and buttons also become outdated because people are frustrated by limited, multi-stage navigation. Solution: The AI Agent interacts with the user through a friendly UI that does not require cludgy menus but conversational and context-sensitive navigation. The Way AI Agents Work: AI agents establish a basis of natural language understanding,…
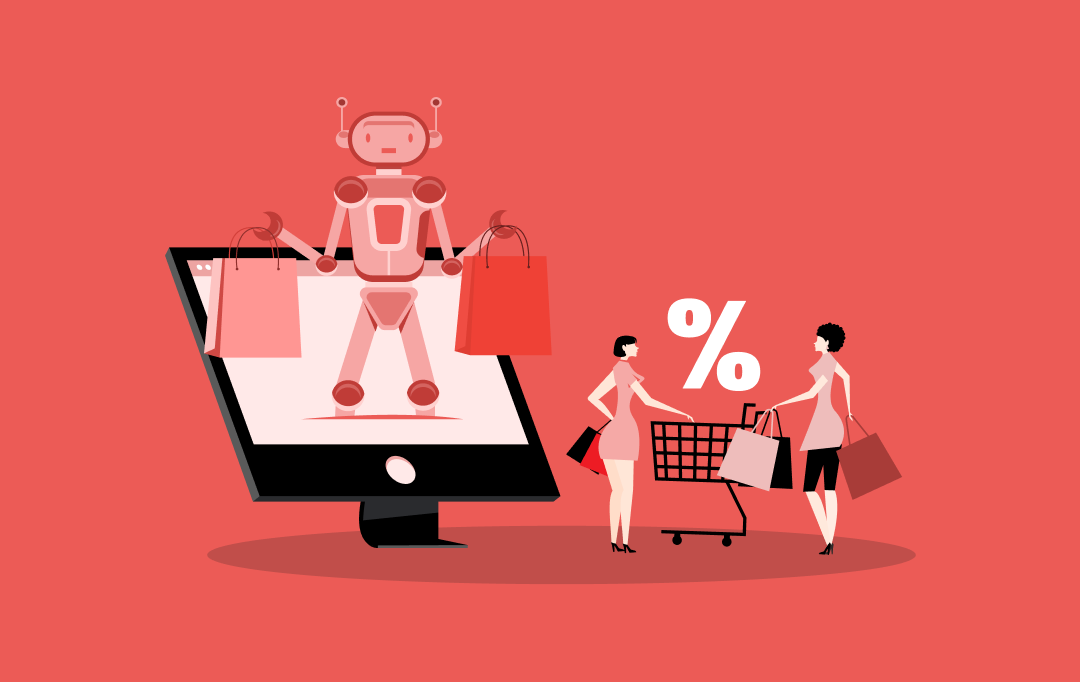
10 Use Cases and Benefits of How AI Agents Are Revolutionizing the Retail Industry
Key takeaways: AI agents in the retail industry are revolutionizing businesses by enhancing customer experience and streamlining operations. From personalized recommendations to predictive analytics, AI offers tangible benefits like increased conversions and reduced costs. AI adoption can lead to scalable growth and a competitive edge in the retail industry. Retailers must consider data strategies, talent…