- AI in the UAE Energy Sector
- Why is AI needed in the Hour for the Emirate’s energy sector?
- Improving Energy Use and Power Grid Management
- Boosting Renewable Energy
- Boosting Predictive Maintenance and Strengthening Infrastructure
- Lowering Emissions and Promoting Sustainability
- Improving Energy Safety and Blocking Cyber Threats
- Boosting Investment and Supporting Economic Diversification
- Best Use Cases of AI in The Energy Sector
- Operational Efficiency and Predictive Maintenance
- Grid Reliability Enhancement and Machine Learning
- Energy Transitions & Decarbonization
- Strengthening Cybersecurity Protocols
- How to Implement AI in Energy Software/Apps?
- Define Objectives and Use Cases
- Collect and Prepare Data
- Choose the Right AI Models
- Develop and Train the AI Model
- Integrate AI into the Software/App
- Test and Validate the Software
- Deploy and Monitor
- Cost Estimations for Implementing AI in the Energy Sector
- Factors Affecting the Cost of Implementing AI in the Energy Sector
- Hidden Costs For Developing & Implementing AI in the Energy Sector
- Strategies to Minimize AI Costs in the Energy Sector
- What are the Challenges and Solutions For Implementing AI in the Energy Sector?
- Challenge 1: Availability and Quality of Data
- Challenge 2: High Implementation Costs
- Challenge 3: Insufficient Skilled Workforce
- Challenge 4: Regulatory and Ethical Concerns
- Challenge 5: Scalability and Adaptability
- How Does Appinventiv Shine as the Go-To AI Tech Partner for Your Energy Development Needs?
- Appinventiv’s Expertise in Oil and Gas Software Development
- FAQs
It’s time for the really big energy upgrade in the UAE, with Artificial Intelligence acting as the MVP in churning these winds of change. This is an intriguing deep dive into why artificial intelligence is far from being just another buzzword, but a necessity for the Emirates’ oil and gas and renewable energy businesses. Artificial intelligence in the energy sector in the UAE is rewriting the operation manual for rig optimization and emission reduction.
AI in Energy Efficiency Market by Application – Global Forecast to 2030
According to Statista, the Artificial Intelligence market in the UAE is expected to grow at an annual rate (CAGR 2025-2031) of 26.22%, resulting in a market volume of US$4.74bn by 2031. Thus, this opportunity led to the inception of attractive opportunities in the AI energy market that revolutionized the energy industry while maintaining sustainability.
AI in the UAE Energy Sector
The energy sector revolution in the UAE comes with the deployment of Artificial Intelligence. The United Arab Emirates (UAE) is leading the way in technological advancements in various industries, most notably AI in energy use. The UAE uses the latest effectiveness, modernity, and energy supply technology. Artificial intelligence in the energy sector in the UAE is changing how we process everything within the energy space, from renewable energy companies to oil and gas providers.
- The Abu Dhabi National Oil Company (ADNOC) reported a $500M value creation record by applying more than 30 cutting-edge AI systems into its value chain. These technologies have helped us achieve autonomous production and reservoir management and prevent the forced venting of as much as 1 million tons of carbon dioxide emissions between 2022 and 2023.
- In the same manner, DEWA is using AI to transform utilities. The company has utilized Gen AI to improve the customer journey and achieved a remarkable 99.62% smart customer service adoption rate in 2023, as reported by Gulf Today.
No matter your industry, be it energy, oil, gas, or anything else, your internal efforts alone won’t determine your achievement with AI. It’s also a question of who your partners are. Depending on who you choose to work with, the right AI tech partner can elevate your profile or leave you struggling to stay afloat. A good partner doesn’t only focus on creating solutions; they craft detailed AI strategies that enhance operational effectiveness while ensuring you remain a market leader.
Let’s understand why AI in the UAE energy sector is a game-changer. We will cover the use cases of artificial intelligence in the UAE’s energy sector, the challenges faced by the energy industry, the associated benefits, and the road ahead. Let’s get down to the meat-and-potatoes of why and how.
UAE businesses, don’t sleep—team up with Appinventiv to dominate the game.
Why is AI needed in the Hour for the Emirate’s energy sector?
With the rapid acceleration of global energy transitions, the Emirates has ambitiously set goals to diversify its energy mix and achieve net-zero emissions by 2050, as per the UAE Net Zero by 2050 Strategic Initiative. With a growing demand, especially from AI-driven technologies, the country seeks to boost the share of AI in renewable energy in the UAE, optimize conventional energy systems, and enhance grid reliability.
The government views AI as a means to diversify the economy and decrease dependency on fossil fuels, thus positioning the Emirates as a front-runner in sustainable innovation. So why is the AI in the UAE Energy Sector important for service providers?
Improving Energy Use and Power Grid Management
AI systems are changing energy use by making grids more efficient and cutting operational costs. Smart grids using AI handle huge amounts of data in real-time. They help predict maintenance needs, forecast energy demand, and balance power loads. A study in Scientific Research Publishing showed that smart grids using AI have made energy grids about 20% more efficient. This highlights how helpful they have become.
Boosting Renewable Energy
The UAE invests significantly in renewable energy. Projects such as the Mohammed bin Rashid Al Maktoum Solar Park plan to produce 5,000 MW by 2030. But adding sources like solar and wind to the grid is tricky because they are not available. AI plays a role by helping to maximize energy output and strengthen the grid.
Boosting Predictive Maintenance and Strengthening Infrastructure
AI and machine learning in the UAE’s energy sector are crucial in regularly maintaining its energy infrastructure, including oil rigs and renewable energy systems, to keep everything running. Predictive maintenance powered by AI relies on machine learning to study sensor data, find unusual patterns, and foresee equipment breakdowns before they happen. This method cuts maintenance costs, avoids delays, and boosts safety.
Lowering Emissions and Promoting Sustainability
The UAE’s energy strategy relies on sustainability as a key focus, and AI has an important role in reducing the carbon footprint tied to energy activities. AI helps to trim greenhouse gas emissions by improving how energy is produced and used. Research from 122 peer-reviewed studies shared on MIT DSpace shows that AI tools have made significant progress in boosting energy efficiency and reducing emissions throughout the energy field.
Improving Energy Safety and Blocking Cyber Threats
Cybersecurity becomes a pressing issue as energy systems rely more on digital technologies. AI can improve energy security by spotting and stopping cyber threats as they occur. A study in ScienceDirect shows that AI methods work better than older models to stop cyberattacks, helping smart grids and IoT-enabled energy setups stay strong.
Boosting Investment and Supporting Economic Diversification
Artificial intelligence in the energy sector in the UAE is not only used to improve efficiency but also to diversify its economy. AI helps the nation rely less on oil and gas, aligning with its UAE Vision 2021 and 2031 plans. According to PwC, the Middle East is set to gain $320 billion from AI by 2030, with the UAE leading efforts in areas like energy.
Best Use Cases of AI in The Energy Sector
Throughout the UAE and MENA region, AI is enhancing processes within the energy sector, accelerating smartness, efficiency, and innovation, and sustaining them all simultaneously. The UAE government and several other energy leaders are using AI to fully optimize operations and minimize carbon footprints while aiding sustainable transitions in energy servicing and diversifying the economy. Below is an in-depth analysis of the application of AI with real case studies illustrating its use.
Operational Efficiency and Predictive Maintenance
What do the best energy service providers do differently from other providers? Energy Predictive Maintenance. Their AI innovation provides them with strategic off-the-shelf analysis that lets them understand possible equipment failure scenarios well before they happen and put mitigation strategies in place. Their AI system examines temperature, vibrations, humidity, moisture, pressure readings, speed, voltage, and even ultrasound data from embedded sensors and equipment alongside other datasets, thousands of data points, to foresee maintenance requirements while decreasing operational delays and expenses.
Real Example: ADNOC’s Predictive Maintenance Program
Abu Dhabi National Oil Company (ADNOC) leverages AI technology suites to perform predictive maintenance on upstream and downstream oil and gas refineries using IoT sensors. The company employs several intelligent AI models that retrieve streaming data from IoT sensors located on equipment such as turbines, pumps, and compressors in chronic monitoring, and diagnose every possible failure in real time before it happens. Additionally, the Machine Learning constituent of AI systems’ algorithms also learns equipment performance, detects the performance pattern, and instantly alarms on unusual activity that signals probable equipment failure while reducing downtime and maintenance spending.
Upshots:
Based on IoT ADP analysis data, they estimate that the AI-powered ADNOC Neuron 5 system can slash unplanned shutdowns by 50% while ramping up planned maintenance activity windows by 20%.
Grid Reliability Enhancement and Machine Learning
Conventionally, the UAE energy sector deployed a manual approach and deterministic models for grid management, ultimately bringing significant grid stability and reliability challenges. Moreover, the companies failed to identify and address the surging variabilities and unpredictabilities. Then, enter the disruptor—machine Learning, which smartly manages and optimizes energy production and distribution, enhancing grid reliability.
Real Example: DEWA’s Smart Grid Optimization
Dubai Electricity and Water Authority (DEWA) optimizes grid management smartly by deploying machine learning and integrating conventional energy sources to power Dubai. The AI system extracts data, based on which machine learning algorithms learn and analyze data like consumption patterns, weather, and grid performance, and adjust these elements while prioritizing renewable energy sources through AI and Renewable Energy Integration.
Bonus Read: Renewable Energy Software: Why It Matters for Businesses and How to Build It Right
Upshots:
According to WAM-Emirates News Agency, AI deployment breaks DEWA’s 1.06 minutes per customer record in 2023. It is significantly lower than the approximately 15 minutes recorded by leading utility companies in the European Union.
Energy Transitions & Decarbonization
Burning fossil fuels alters the temperature and wreaks havoc on the environment. If industries want to continue having a positive impact, they must shift to renewable resources and decarbonize. This approach fosters economic growth and energy independence by altering the negative perception of the environment and reducing reliance on fossil fuels. Decarbonisation reduces air pollution.
Real Example: Emirates Water and Electricity Company (EWEC)
Along with other innovations brought by artificial intelligence, the Emirates Water and Electricity Company leveraged Artificial Intelligence to transform outdated systems. Now, EWEC can adjust its strategy to meet set decarbonization achievement milestones and switch to environmentally sustainable materials. Here’s what artificial intelligence technologies offer.
- AI Integration: After the lockdown, EWEC focused on operational flow restoration and net revenue streams, thus holistically implementing AI-powered energy management systems with advanced analytics, workflow automation, and cyber protection.
Bonus Read: Building Energy Management Systems for Carbon Neutrality
- Grid Development: AI now helps EWEC manage grid stability and flexibility with automated, real-time, and advanced problem-solving applications. Complex task simplifications and sophisticated execution guarantee balanced energy source supply.
- Prescriptive Analytics: Creating energy sources is the best option within the scope of processes for deconstructing combustible machines. AI-implemented advanced data analytics enhances forecasting.
Upshots:
This helps EWEC to plan for an average of 1.4GW of new solar PV capacity per year between 2027 and 2037. It also recommends that projects that use renewable and clean energy be set to provide more than 50 percent of Abu Dhabi’s electricity by 2030. (Source: EWEC)
Strengthening Cybersecurity Protocols
Implementing AI technologies in the energy sector has transformed system safety and security for this industry. AI in cybersecurity ensures proactive supervision of system parameters such as network traffic, operational data for anomaly detection, and much more. In addition, predictive analytics fueling AI systems can swiftly scan enormous datasets to identify data, predicting threats or unusual patterns of suspicious activities much more efficiently than before.
Real Example: The Emirates Nuclear Energy Corporation (ENEC)
It is remarkable how far ENEC has come in reinforcing safeguards against cyber intrusion while utilizing AI in the UAE Energy Sector. With the help of the International Atomic Energy Agency (IAEA), ENEC hosted a forum discussing how AI in the UAE Energy Sector can protect nuclear processing against cyber threats while utilizing AI.
Upshots:
According to Utility Business MENA, Mohamed Al Hammadi, Managing Director and CEO of ENEC, highlighted the growing importance of cybersecurity in protecting critical infrastructure like the Barakah Nuclear Energy Plant, which supplies 25% of the UAE’s electricity.
These cases show AI being hardcore embraced across the UAE’s energy scene. From cranking out power to delivering it, using it, and planning, the vibe is about slamming machine learning and automation for maximum results. Every real-world example pumps out data that tightens up AI models, speeding up the whole takeover.
Crush energy distribution challenges and predict equipment failures with our cutting-edge AI solutions.
How to Implement AI in Energy Software/Apps?
The energy sector is undergoing digital transformation, and AI is at the cusp of innovation. AI-powered software and apps are reshaping energy production, distribution, and consumption, from optimizing renewable energy systems to ensuring grid reliability. Here is a thorough guide to AI implementation in energy software/apps, covering use cases, technicalities, tools, and implementation.
Define Objectives and Use Cases
Start by identifying the problem you want to solve. For example:
- Are you building an app to optimize home energy consumption?
- Do you need software to predict wind turbine maintenance?
- Is the goal to enhance grid reliability?
Clearly define measurable objectives, such as reducing energy waste by 10% or improving prediction accuracy for solar output.
Collect and Prepare Data
AI thrives on data. Energy software relies on diverse datasets, including:
- Historical Data: Past energy consumption, production, or equipment performance.
- Real-Time Data: Sensor data from smart meters, IoT devices, or grid systems.
- External Data: Weather forecasts, market prices, or regulatory data.
Data Preparation Steps:
- Clean Data: Remove duplicates, handle missing values, and correct inconsistencies.
- Normalize/Scale: Ensure data is in a consistent format (e.g., scaling sensor readings to a 0-1 range).
- Feature Engineering: Create relevant features, such as average daily consumption or peak load times.
- Label Data: For supervised learning, label data (e.g., “equipment failure” or “normal operation”).
Tools: Python (Pandas, NumPy), SQL, Apache Spark for large-scale data processing.
Choose the Right AI Models
Select AI/ML models based on your use case:
- Regression Models: For predicting continuous value variables like energy output or consumption (e.g., Linear Regression, Random Forest).
- Time-Series Models: For forecasting (e.g., ARIMA, LSTM for energy demand prediction).
- Classification Models: For detecting anomalies or equipment failures (e.g., SVM, Decision Trees).
- Deep Learning: For complex tasks like image-based defect detection in solar panels (e.g., CNNs).
- Reinforcement Learning: For optimizing energy trading or grid control strategies.
Tools: TensorFlow, PyTorch, Scikit-learn, Keras.
Develop and Train the AI Model
- Split Data: Divide data into training (70-80%), validation (10-15%), and test (10-15%) sets.
- Train Model: Use the training data to optimize model parameters.
- Validate: Fine-tune hyperparameters using the validation set to avoid overfitting.
- Test: Evaluate the model on the test set to ensure generalization.
Best Practices:
- Use cross-validation for robust performance.
- Monitor metrics like Mean Absolute Error (MAE) for regression or F1-score for classification.
- Optimize for computational efficiency, especially for real-time applications.
Integrate AI into the Software/App
Embed the trained AI model into your energy software or app. This involves:
- API Development: Create APIs to serve model predictions (e.g., Flask, FastAPI).
- Frontend Integration: Build user-friendly interfaces using React, Angular, or Flutter frameworks.
- Backend Integration: Connect the AI model to databases (e.g., PostgreSQL, MongoDB) and real-time data streams (e.g., Kafka, MQTT).
- Cloud Deployment: Use cloud platforms like AWS, Azure, or Google Cloud for scalability.
Test and Validate the Software
- Functional Testing: Ensure the app meets user requirements (e.g., accurate predictions, fast response times).
- Performance Testing: Verify the software handles peak loads and real-time data streams.
- User Testing: Gather feedback from end-users to refine the interface and functionality.
Deploy and Monitor
- Deployment: Launch the software/app on the target platform (web, mobile, or embedded systems).
- Monitoring: Track performance metrics like prediction accuracy, latency, and user engagement.
- Continuous Learning: Retrain models periodically with new data to maintain accuracy.
- Maintenance: Address bugs, update dependencies, and scale infrastructure as needed.
Tools: Docker, Kubernetes, Prometheus (for monitoring), Jenkins (for CI/CD).
Cost Estimations for Implementing AI in the Energy Sector
The cost of implementing Artificial Intelligence in the energy sector in the UAE typically ranges from $45,000 to $600,000+. The costs vary widely depending on several factors, including the type of software, its complexity, the development team’s expertise, and the time required to complete the project. Here’s a breakdown of the estimated cost of AI in the UAE Energy Sector based on the complexity.
Complexity Aspect | Features | Cost Estimation |
---|---|---|
AI Basic Project | Include: predictive maintenance or energy consumption forecasting. | $45,000 to $250,000 (1,65,282 AED to 9,18,238 AED) |
Medium Level Project | Include: asset optimization, demand forecasting, smart metering, or fault detection across a grid or energy distribution network. | $500,000 – $5 million (18,36,477 AED to 1,83,64,775 AED) |
Large Scale Project | Includes: upstream/downstream operations, renewables, smart grid, and energy trading | $10 million – $50+ million (3,67,29,550 AED to 18,36,47,750 AED) |
Factors Affecting the Cost of Implementing AI in the Energy Sector
Several essential factors influence the costs of implementing AI in the UAE Energy Sector. These factors significantly impact the performance and user satisfaction of your app/software, ultimately shaping the overall cost of AI development in the UAE. Let’s explore these factors in detail and dive into their gravity and impact on numbers!
Factors | Description | Examples | Cost Impact |
---|---|---|---|
Data Availability | Clean and Structured data reduces development overhead | Cloud-based data lakes and IoT sensors. | $100,000-$300,000 in savings |
Legacy Infrastructure | Older systems have less compatibility with AI solutions. | Outdated grid systems and siloed databases | $200,000 – $500,000 in added costs |
Regulatory Complexity | Compliance uplifts infrastructure costs. | GDPR and AI ethics governance. | $100,000 – $250,000 in added costs |
AI Maturity Level | AI tools and practices reduce custom development costs. | Pre-trained ML models, DevOps-ready AI tools | $150,000 – $400,000 in savings |
Custom AI Solutions | Tailored AI solutions are expensive but ensure maximum ROI. | Bespoke AI for energy distribution and optimization | $300,000 – $700,000 in added cost |
Hidden Costs For Developing & Implementing AI in the Energy Sector
Now, you know the obvious costs of designing, developing, and implementing AI in the energy sector, but several hidden costs can arise unexpectedly, affecting the overall budget. Understanding these can help with better financial planning and management.
Hidden Cost Area | Estimated Impact |
---|---|
Data Cleaning & Labeling | $50,000 – $500,000+ |
Model Retraining & Drift Handling | $20,000 – $150,000 per year |
Change Management & Training | $50,000 – $300,000+ |
Custom Integrations | $100,000 – $1 million+ |
Cybersecurity Upgrades | $100,000 – $500,000+ |
Strategies to Minimize AI Costs in the Energy Sector
You don’t have to break the bank to develop and implement AI in the energy sector. Smart optimization strategies can significantly reduce costs without sacrificing quality. Energy service providers in the UAE can create AI-powered energy solutions on a lean budget by focusing on efficiency, prioritization, and modern tools. Here’s how to effectively minimize costs with actionable insights for each approach.
Areas | Cost Optimizing Strategies | Estimated Cost Savings |
---|---|---|
Data Management | Instead of creating a new pipeline, deploy existing data lakes or IoT sensors. | $50,000 – $200,000 |
Model Development | Leverage open-source, pre-trained models and AutoML. | $100,000 – $300,000 |
Infrastructure | Use cloud AI platforms | $200,000 – $ 1M+ |
What are the Challenges and Solutions For Implementing AI in the Energy Sector?
AI has been changing the landscape of industries worldwide, and energy is no exception. Since energy and power innovation in the UAE are fundamentally set for economic diversification and sustainability goals, AI holds tremendous potential to optimize operations, enhance efficiency, and give impetus to the clean energy agenda.
But implementing AI in energy is not a plug-and-play process. There are some major challenges for which reasonable solutions must be found.
Challenge 1: Availability and Quality of Data
AI usually works on data, but the energy industry is often plagued with poor-quality, incomplete, or siloed data. Older systems in oil and gas processing facilities, power plants, and utilities can produce disjointed datasets that are challenging to consolidate.
Solutions:
- Data Standardization and Integration: Spend in data management platforms that standardize the formats and allow easy integration between systems.
- IoT and Sensor Deployment: Deploy Internet of Things (IoT) devices and smart sensors to gather real-time, high-quality data.
- Data Governance Frameworks: Set strict policies to maintain data accuracy, security, and accessibility.
Challenge 2: High Implementation Costs
Implementing AI solutions involves substantial initial investment in infrastructure, software, and qualified staff. For UAE energy firms, especially the smaller ones or those shifting to renewables, the price of AI hardware, cloud computing, and recruiting talent can be too high.
Solution:
Cloud-Based AI Solutions: Take advantage of cloud platforms such as Google Cloud, Azure or AWS, which provide scalable AI solutions at reduced prices compared to on-premises infrastructure.
Challenge 3: Insufficient Skilled Workforce
AI deployment requires data science, machine learning, and energy domain expertise. Although the UAE government has spent heavily on education and innovation, there is still a shortage of professionals connecting AI and energy expertise. Consequently, this hinders projects and lowers their impact.
Solution:
Hire an AI development company over freelancers: There are several compelling reasons to hire artificial intelligence developers over freelancers and an in-house team, including:
- AI developers possess advanced technical skills that allow them to leverage machine learning and data analytics to drive innovation and efficiency within your organization.
- They can develop sophisticated algorithms and systems tailored to your specific needs, such as predictive analytics, automation, and personalized customer experiences.
- They can help you gain a competitive edge and stay ahead in today’s tech-savvy landscape by integrating cutting-edge technology into your digital products and services.
By hiring an AI developer, you can make data-driven decisions that align with your business goals, ensuring that your company remains at the forefront of technological advancements.
Challenge 4: Regulatory and Ethical Concerns
The energy industry is already heavily regulated, and AI complicates data privacy, cybersecurity, and responsibility matters. In the UAE, where energy installations are part of national defense, keeping AI systems in line with regulations such as the UAE Data Protection Law is essential.
Solution:
Strong Cybersecurity Practices: Adopt strong encryption and secure deployment of AI models to guard confidential energy information. The UAE Cybersecurity measures for businesses give guidelines for securing essential infrastructure.
Challenge 5: Scalability and Adaptability
AI algorithms built for certain energy use cases—e.g., wind turbine performance optimization or grid load prediction—can be challenged to scale in varied environments or to accommodate shifts in conditions.
Solutions:
- Modular AI Architectures: Design modular AI components that are easily adaptable or scalable. Modular architecture supports cross-industry applications across various energy subsectors, from fossil fuels to renewables.
- Continuous Learning Models: Use AI models that learn and improve over time with technologies such as reinforcement learning.
Want the real cost of AI-powered energy solutions? Grab a custom quote from our AI experts that is tailored to you.
How Does Appinventiv Shine as the Go-To AI Tech Partner for Your Energy Development Needs?
At the heart of the digital revolution is Artificial Intelligence (AI), which is changing how companies manage exploration, drilling, asset management, and market volatility. To navigate this complex landscape, businesses need a trusted tech partner—one that combines deep industry knowledge with AI solutions. Enter Appinventiv, a global oil and gas software development company and your go-to AI tech partner.
Appinventiv’s Expertise in Oil and Gas Software Development
Being a trusted software development company in Dubai, we offer end-to-end software development services to digitalize and optimize internal processes. Our AI-driven solutions across the energy sector address the unique needs of each segment, ensuring holistic transformation for energy businesses. Here’s how our AI-powered innovations make you stand out in the oil and gas tech landscape:
- Predictive Maintenance: AI algorithms predict equipment failures before they happen, reducing downtime and maintenance costs.
- Real-Time Market Analysis: AI tools process massive datasets to give you insights so that you can adapt to market changes.
- Automation: From robotic process automation (RPA) to AI-driven workflows, Appinventiv automates repetitive tasks so you can focus on strategy.
We offer sustainable and scalable solutions, whether custom IoT platforms, predictive analytics tools, or complex solutions. We can be your ambassadors. Partner with us now!
FAQs
Q. How will the future of AI in the UAE’s energy sector contribute to sustainability?
A. The future of AI in the UAE’s energy sector focuses on sustainability by improving renewable energy integration, such as solar and wind, and minimizing carbon emissions. AI-driven solutions enable smarter energy consumption, demand forecasting, and efficient storage systems, aligning with the UAE’s Net Zero by 2050 goals.
Q. How is AI transforming traditional energy management practices?
A. AI in the UAE’s Energy Management System shifts from manual, reactive approaches to predictive, real-time systems. AI-powered energy management systems enable utilities and businesses to monitor consumption, predict demand, automate load balancing, and boost efficiency. AI driven energy management systems ensure analytics and automation deliver faster, data-backed decisions, cutting costs, reducing outages, and promoting sustainability.
Q. How is AI transforming the energy industry in the UAE?
A. In the UAE, AI enhances renewable energy production, smart grid operations, and demand forecasting. From predictive maintenance in solar plants to real-time grid balancing, AI drives efficiency and supports the shift to sustainable energy systems.
Q.What are some successful AI-driven energy projects in the UAE?
A. Notable AI-driven projects in the UAE include Masdar’s AI-optimized solar energy systems, DEWA’s AI-enhanced smart grid programs, and DEWA’s AI chatbot “Rammas” for customer service.
Q. What is the role of AI in smart grids in the UAE?
A. AI enables smart grids in the UAE with real-time monitoring, predictive maintenance, and automated demand response. It balances energy loads, quickly detects faults, and effectively integrates renewable sources like solar and wind.
Q. How does AI align with the UAE Energy Strategy 2050?
A. AI supports the UAE Energy Strategy 2050 by improving energy efficiency, accelerating clean energy adoption, and enabling data-driven policies. It reduces consumption, optimizes grids, and lowers emissions, aligning with the goal of 50% clean energy and a reduced carbon footprint.
Q. Are there government initiatives in the UAE promoting AI in energy efficiency?
A. The UAE promotes AI in energy through initiatives like the Dubai Clean Energy Strategy, AI Strategy 2031, DEWA’s Future Lab, and AI Innovation Center. These foster research, public-private collaboration, and an ecosystem for AI adoption in energy systems.
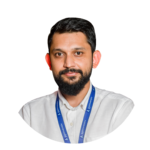
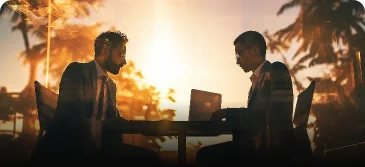
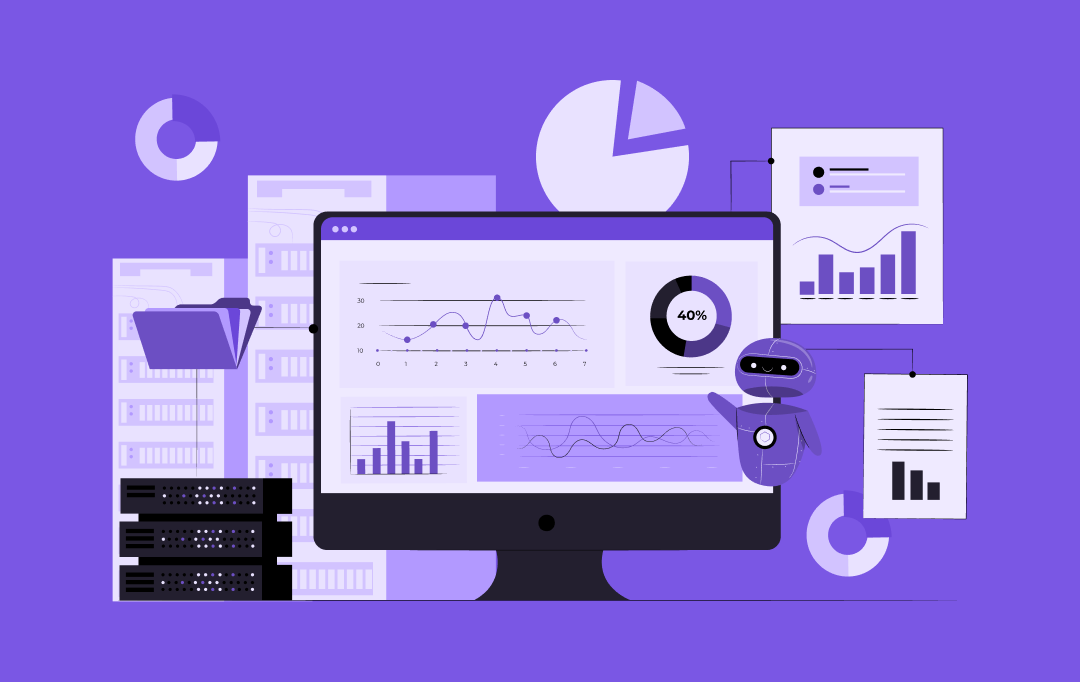
Exploring the Power of AI in Creating Dynamic & Interactive Data Visualizations
Key takeaways: AI transforms data visualization by providing automated insights, interactive dashboards, and NLP, thereby tackling revenue loss and improving data quality. Key features include predictive analytics, real-time data integration, and user-friendly visualizations, enabling faster and more informed decisions. Top 2025 tools, such as Tableau, Power BI, and Julius AI, offer NLP and real-time analytics…
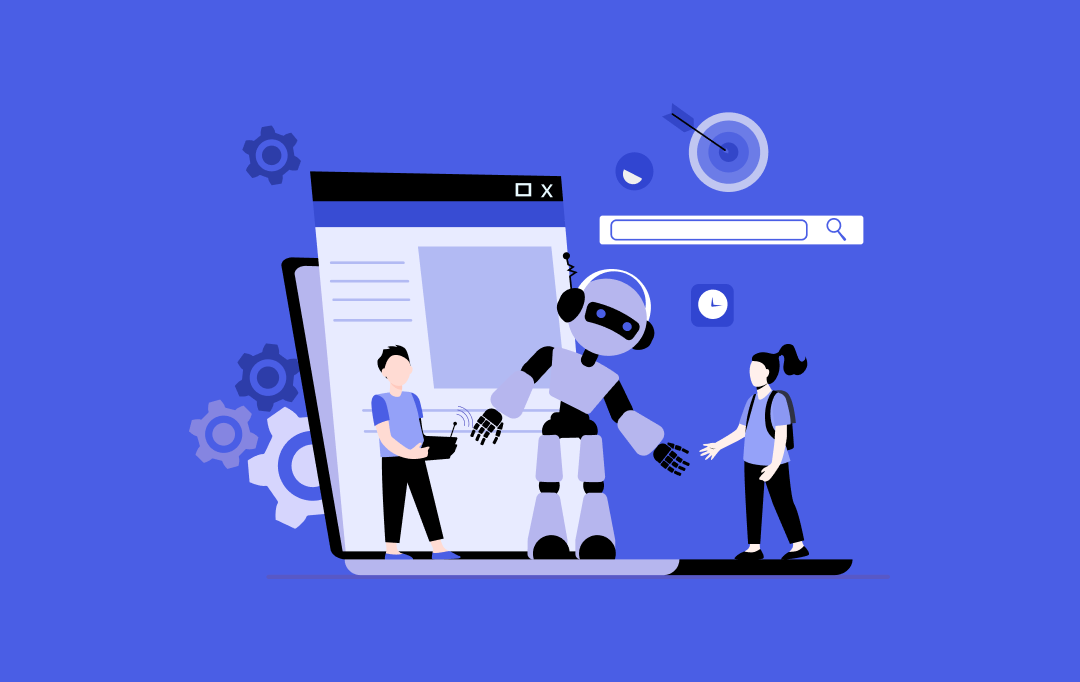
Practical Applications of AI as a Service for Your Business
Key takeaways: AIaaS enables fast, infrastructure-free AI adoption. Used across industries for automation and insights. Real-world examples show proven business impact. Pilot-first approach ensures smooth implementation. Key challenges include data, cost, and scaling. Trends include ethical AI, edge, and SME growth Imagine being able to cut down your operational costs while simultaneously improving decision-making and…
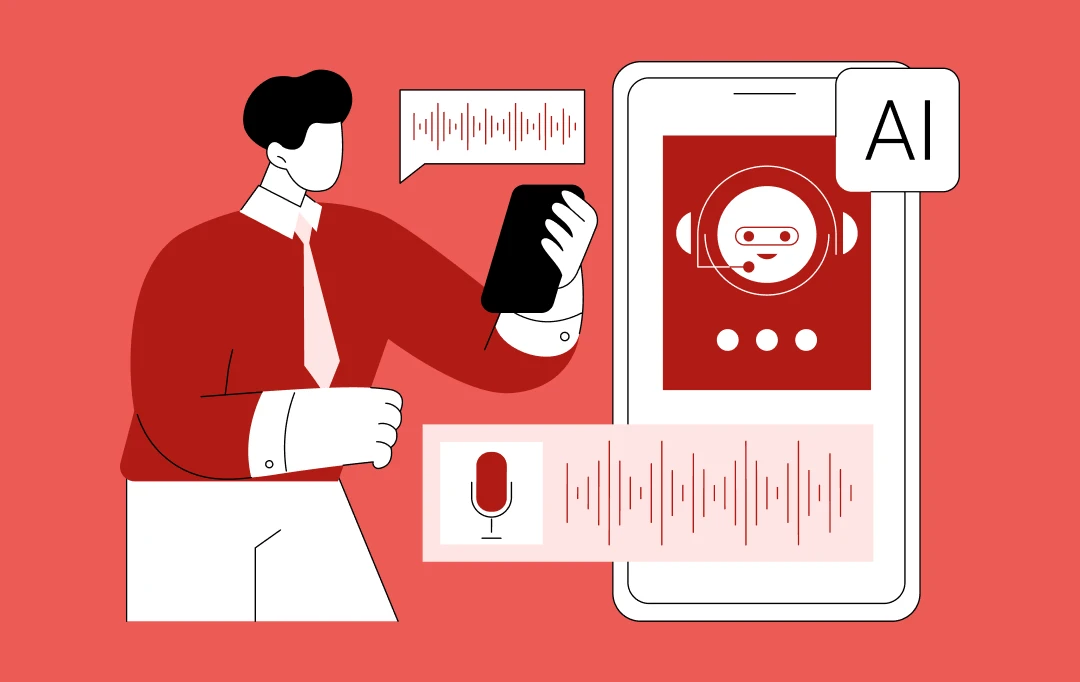
How to Build an AI-Powered Language Learning App? Features, Process, Costs
Key takeaways: AI is transforming language learning with personalized, engaging experiences. Building an AI language learning app involves research, design, AI integration, and testing. Key features include speech recognition, adaptive learning, and gamification. Development costs range from $50,000 to $250,000 based on complexity. Advanced features like NLP, AR, and real-time AI tutors enhance the app’s…