- What is Multimodal AI and How Does it Work?
- Data Collection and Preprocessing
- Feature Extraction
- Data Fusion
- Model Training
- Generation and Inference
- Recommendations and Enhancements
- Comparing Generative, Unimodal, and Multimodal AI: Key Differences
- Top 11 Multimodal AI Applications and Real-Life Use Cases across Industries
- Healthcare
- Finance
- eCommerce
- Education
- Manufacturing
- Agriculture
- Retail
- Consumer Technology
- Energy
- Social Media
- How Appinventiv Helped Vyrb Secure $1Million+ Funding with Its Multimodal AI Capabilities
- Top Multimodal AI Benefits for Businesses
- Versatility in Real-World Scenarios
- Robust Performance
- Comprehensive Understanding
- Increased Accuracy
- Enhanced Context-Awareness
- Advanced Problem-Solving
- Scalability
- Top Multimodal AI Models Shaping the Future of Artificial Intelligence
- GPT-4
- CLIP
- DALL-E
- MUM
- VisualBERT
- Florence
- Addressing the Challenges in Multimodal AI Implementation
- Computational Complexity and Scalability
- Data Integration and Management
- Interpreting Multimodal Data
- Data Security and Privacy
- The Future Trajectory of Multimodal AI
- Embark on the Multimodal AI Revolution with Appinventiv's Expertise
- FAQs
In a technology-driven world where devices can interpret gestures, recognize faces, and even perceive emotions alongside understanding spoken words, multimodal AI emerges as a transformative field. This technology integrates various artificial intelligence disciplines, combining computer vision, natural language processing, and sensory inputs to create systems that interact with humans and their environment in nuanced and sophisticated ways.
Multimodal AI plays a crucial role in revolutionizing human-technology interaction, enabling natural and intuitive interfaces across diverse applications, from virtual assistants to autonomous vehicles. The current market trajectory highlights its importance; industries are increasingly investing in these technologies to enhance user experiences, streamline operations, and unlock new capabilities that were previously limited by single-mode AI systems.
As per a recent report by Grand View Research, the global multimodal AI market is projected to reach a valuation of $10.89 billion by 2030, driven by factors such as rapid advancements in deep learning algorithms that enhance the accuracy and robustness of multimodal systems.
Additionally, the increasing integration of AI in consumer electronics and the automotive sector is spurring growth in multimodal AI applications. The demand for seamless human-machine interaction across various industries, including healthcare, retail, and entertainment, further propels the adoption of multimodal AI applications.
This blog will delve into multimodal AI comprehensively, covering its definition, operational principles, the distinctions between three main AI models, its types, top 10 multimodal AI use cases, implementation challenges, and its future potential. Let’s explore each of these aspects.
What is Multimodal AI and How Does it Work?
Multimodal AI marks a pivotal evolution in artificial intelligence, allowing it to effectively process and merge data from diverse input formats such as text, images, audio, and video. This integrated capability enhances the AI’s capacity to grasp intricate contexts and provide precise insights and responses.
By synthesizing information across multiple modalities, the multimodal AI applications excel in addressing complex challenges across various fields, from medical diagnosis to autonomous navigation. Such versatility highlights the crucial role of multimodal AI in advancing technology’s ability to comprehend and interact with our environment.
Here’s how multimodal AI models work:
Data Collection and Preprocessing
Multimodal AI systems collect information from various sources, including audio recordings, written documents, photos, and videos. This data is then preprocessed to ensure it is clean, structured, and ready for analysis.
Feature Extraction
The AI extracts each modality’s pertinent features. For instance, computer vision techniques analyze image data, while natural language processing (NLP) methods process text data.
Data Fusion
To generate an extensive understanding of the input, the multimodal AI architecture integrates the elements retrieved from several modalities. Other ways to accomplish this fusion exist, like early fusion, which combines raw data, or late fusion, which combines processed data.
Model Training
The AI model is trained using a sizable and varied dataset containing instances from all pertinent modalities. During the training phase, the AI model is optimized to reliably read and relate data from various sources.
Generation and Inference
After being trained, the multimodal AI can carry out inference, which entails predicting the future or coming up with answers based on fresh, unobserved data. It can, for instance, produce an image description, translate words in a video, or provide pertinent information in response to a stated question.
Recommendations and Enhancements
Through feedback and further training, the multimodal AI applications continuously enhance its comprehension and integration of multimodal data.
Comparing Generative, Unimodal, and Multimodal AI: Key Differences
Different types of AI technologies, such as Generative AI, Unimodal AI, and Multimodal AI, are related but serve distinct purposes. For instance, Generative AI is used for creating new content, such as text or images. Unimodal AI, on the other hand, is designed to process a single type of data, such as text or visual information. Meanwhile, Multimodal AI integrates multiple types of data, providing a more comprehensive analysis and richer insights.
Explore the table below for a detailed comparison of multimodal AI vs generative AI vs unimodal AI.
Features | Generative AI | Unimodal AI | Multimodal AI |
---|---|---|---|
Definition | AI designed to create new data or content | AI that processes and understands a single type of data | AI that integrates and processes multiple types of data |
Primary Use Cases | Automated text generation, image synthesis, content creation | Language translation, image classification, speech recognition | Autonomous driving, healthcare diagnostics, advanced surveillance |
Advantages | High creativity and realistic content generation | High performance in specialized tasks, simplicity | Rich insights and comprehensive understanding |
Training Data | Requires large, diverse datasets of the type it generates | Needs datasets specific to the single data type it processes | Utilizes large, diverse datasets covering multiple data types |
Challenges | Quality control, ethical considerations, computational demands | Limited to a single modality, might miss context from other data types | Integration complexity, higher computational requirements, data synchronization |
Examples | GPT-4, DALL-E, Stable Diffusion | BERT (for text), ResNet (for images) | CLIP, GPT-4 Vision, Perceiver IO |
Top 11 Multimodal AI Applications and Real-Life Use Cases across Industries
Multimodal AI is transforming industries by integrating different data types that overall enhance customer experiences, streamline processes, and open new avenues for innovation. Here’s a look at the top 10 different use cases of multimodal AI showcasing its immense potential across industries.
Healthcare
In the healthcare sector, multimodal AI combines data from various sources, including electronic health records (EHRs), medical imaging, and patient notes, to enhance diagnosis, treatment strategies, and personalized care. This method boosts accuracy and efficiency by merging different data types to offer a comprehensive view of patient health.
By integrating these data sources, multimodal AI can uncover patterns and correlations that might be overlooked when each data type is analyzed separately, resulting in more accurate diagnoses and customized treatment plans. Additionally, this approach enables proactive healthcare through AI by forecasting potential health issues before they become severe, promoting early intervention, and improving patient outcomes.
The best examples of multimodal AI in action can be seen in IBM Watson Health, which integrates data from EHRs, medical imaging, and clinical notes. This combination enables accurate disease diagnosis, predicts patient outcomes, and aids in creating personalized treatment plans.
Another example can also be seen in DiabeticU, a diabetes management app crafted by Appinventiv, aimed at empowering users to take charge of their health journey. With features such as tailored meal plans, medication tracking, exercise routines, blood sugar monitoring, and an AI-driven virtual assistant, the app integrates effortlessly with wearable devices.
Automotive
Automakers use multimodal AI to improve autonomous driving and vehicle safety. By merging data from sensors, cameras, radar, and lidar, multimodal AI enhances real-time navigation, decision-making, and vehicle performance. This integration helps in detecting and responding to complex driving scenarios, such as recognizing pedestrians and interpreting traffic signals, thereby boosting the safety and reliability of autonomous vehicles. It also supports advanced driver-assistance systems like automated emergency braking and adaptive speed control.
One of the main multimodal AI examples is Toyota’s innovative digital owner’s manual, which employs the same to transform the traditional owner’s manual into a dynamic digital experience by integrating large language models with generative AI. This innovative approach allows Toyota to deliver an interactive manual that blends text, images, and contextually relevant information.
Through advanced natural language processing and multimodal generative AI, the system offers personalized responses, visual aids, and real-time updates on vehicle features. This integration enhances the user experience by making it simpler for owners to find and understand essential information about their vehicles.
Finance
In the finance sector, multimodal AI applications enhance risk management and fraud detection by merging diverse data types, such as transaction logs, user activity patterns, and historical financial records. This integration allows for a more thorough analysis, helping to identify unusual patterns and potential threats more effectively, leading to improved fraud detection and risk assessment.
A perfect example of utilization of multimodal AI in FinTech is JP Morgan’s DocLLM. By combining textual data, metadata, and contextual information from financial documents, DocLLM improves the accuracy and efficiency of document analysis. This multimodal approach supports better risk evaluation and compliance, automates document processing tasks, and provides deeper insights into financial risks.
eCommerce
In the eCommerce sector, multimodal AI improves the customer experience by combining data from user interactions, product visuals, and customer reviews. This integration enhances product recommendations, tailors marketing efforts, and optimizes inventory management. By analyzing diverse data sources, eCommerce platforms can provide more accurate suggestions, improve product placement, and boost overall customer satisfaction.
Amazon utilizes multimodal AI to enhance its packaging efficiency. By merging data from product sizes, shipping needs, and current inventory, Amazon’s AI system identifies the best packaging solutions, minimizing waste and excess material. This approach not only improves packaging precision but also aligns with Amazon’s sustainability goals, making their eCommerce operations more efficient and environmentally friendly.
Education
Through the integration of data from multiple sources, including text, video, and interactive content, multimodal AI applications improve learning opportunities in the education industry. Customizing instructional materials to match each student’s needs and learning preferences enhances personalized learning. This approach increases student engagement by using interactive and multimedia-rich content.
For instance, Duolingo uses multimodal AI to improve its language-learning software. Duolingo produces interactive, individualized language courses that adjust based on the learner’s ability level and progress by fusing text, audio, and visual elements. Through a range of learning modes, AI in education helps reinforce language skills, improving the effectiveness and engagement of the educational process.
Also Read- 12 Ways AI in Education is Transforming the Industry
Manufacturing
In manufacturing, multimodal AI streamlines production by integrating data from machinery sensors, production line cameras, and quality control reports. This approach enhances predictive maintenance, quality assurance, and overall production efficiency.
Bosch employs multimodal AI in manufacturing processes by analyzing audio signals, sensor data, and visual inputs. Their AI systems monitor equipment health, predict maintenance needs, and ensure product quality. This integration allows Bosch to improve operational efficiency, reduce downtime, and uphold high manufacturing standards.
Agriculture
In agriculture, multimodal AI enhances crop management and farming efficiency by integrating data from satellite imagery, on-field sensors, and weather forecasts. This comprehensive data analysis allows for precise monitoring of crop health, efficient water and nutrient management, and timely intervention for pest and disease control. By leveraging these diverse data sources, farmers can make more informed decisions, improve resource allocation, and predict agricultural outcomes with greater accuracy.
John Deere leverages multimodal AI to transform its agricultural machinery and practices. The company integrates technologies such as computer vision, IoT, and machine learning to enable advanced capabilities like precision planting and real-time crop monitoring. This data-driven approach optimizes field operations, reduces waste, and enhances crop yields by providing actionable insights based on real-time data.
Retail
Multimodal AI enhances retail efficiency by merging data from shelf cameras, RFID tags, and transaction records. This integration supports better inventory management, accurate demand forecasting, and personalized promotions, leading to streamlined supply chain operations and improved customer experiences.
Walmart applies multimodal AI to refine its supply chain and in-store operations. By combining data from shelf cameras, RFID tags, and transaction records, Walmart improves inventory management, enhances demand forecasting, and customizes promotions. This approach boosts operational efficiency and better serves customer needs.
Also Read: AI in Retail – How Artificial Intelligence is Improving the Retail Shopping Experiences
Consumer Technology
Multimodal AI enhances voice-activated assistants by integrating voice recognition, natural language processing, and visual information. This combination enables devices to deliver interactive and contextually relevant responses, improving user experience and interaction across various applications.
Google Assistant utilizes multimodal AI by merging voice recognition, natural language processing, and visual data to offer a seamless and interactive user experience on smart devices. This integration allows for intuitive voice commands, personalized responses, and enhanced functionality across various tasks and applications.
Energy
Multimodal AI boosts energy sector performance by combining data from operational sensors, geological surveys, and environmental reports. This integration facilitates more effective resource management, optimizes energy production processes, and improves overall operational efficiency. By analyzing diverse data sources, energy companies can make more informed decisions, anticipate potential issues, and adapt strategies in real-time.
ExxonMobil leverages multimodal AI by synthesizing data from operational sensors, geological surveys, and environmental reports. This approach enhances energy production efficiency, improves resource management, and drives operational excellence. By integrating these data streams, ExxonMobil can better predict equipment needs, optimize drilling operations, and respond swiftly to environmental changes, ultimately leading to increased productivity and sustainability.
Social Media
Multimodal AI in social media combines data from various sources like text, images, and video content to improve user interactions and content management. By analyzing these different data types, the AI system more accurately gauges user sentiments, trends, and engagement patterns.
This approach enhances content recommendations, enables targeted advertising, and improves the detection of harmful or inappropriate content. As a result, multimodal AI delivers a more personalized and engaging user experience while optimizing overall platform performance and user satisfaction.
How Appinventiv Helped Vyrb Secure $1Million+ Funding with Its Multimodal AI Capabilities
Appinventiv was instrumental in securing over $1 million in funding for Vyrb by developing an innovative social media app with advanced multimodal AI features. By incorporating voice command features alongside popular assistants like Siri and Google Assistant, we enabled users to create, listen to, and respond to posts effortlessly. We also added an autoplay feature for smooth audio streaming of feeds and expanded voice messaging with speech-to-text capabilities and a voice-focused inbox.
These enhancements contributed to the app’s success, resulting in over 50,000 downloads and $1+ million in funding.
Top Multimodal AI Benefits for Businesses
Multimodal AI has a series of benefits that enhance performance, accuracy, and adaptability across diverse applications, leading to more insightful and effective solutions. Let’s have a look at some of those:
Versatility in Real-World Scenarios
By combining data from multiple sources, multimodal AI can more successfully handle a larger range of real-world applications and is more flexible to accommodate a variety of use cases.
Robust Performance
By combining several modalities, AI becomes more capable of handling a wider range of challenging tasks, which leads to more reliable and flexible AI solutions.
Comprehensive Understanding
Multimodal AI systems integrate information from various modalities, offering a more detailed and complete perspective on the context or issue being addressed.
Increased Accuracy
Compared to single-modality systems, multimodality AI offers a more accurate interpretation of information and lowers errors by combining a variety of data kinds (text, images, audio, etc.).
Enhanced Context-Awareness
By considering various data sources, multimodal AI applications make complex inquiries easier to interpret and produce more contextually relevant answers.
Advanced Problem-Solving
Multimodal AI’s ability to synthesize data from different sources facilitates more effective and innovative solutions to complex problems.
Scalability
The flexibility of multimodal AI enables it to scale across different industries and applications, supporting growth and adaptation as business needs evolve.
Top Multimodal AI Models Shaping the Future of Artificial Intelligence
Multimodality AI uses unique models that extend the capabilities of artificial intelligence. These models combine different types of data to provide advanced insights. Here’s a detailed list of multimodal AI models.
GPT-4
OpenAI created the cutting-edge generative language model GPT-4, which can process and produce text. While it is mainly focused on text production and understanding, it incorporates certain multimodal capabilities, such as image understanding.
GPT-4 is one of the most popular multimodal AI applications, well-known for its sophisticated natural language generation and understanding. It can do various tasks, from answering simple queries to generating complex content.
Also Read: The Breakthrough that is ChatGPT: How Much Does It Cost to Build?
CLIP
Using a sizable dataset of image-text pairings for pretraining, OpenAI’s CLIP algorithm learns to correlate images with words. It comprehends images and produces words about them, and vice versa. CLIP can match images with written descriptions even for tasks on which it was not specifically trained. It excels in zero-shot learning tasks.
DALL-E
Another generative model from OpenAI is DALL-E, which uses textual descriptions to produce visuals. It creates original visual material in response to text cues by fusing natural language understanding with image production.
DALL-E demonstrates the potential of fusing text and image modalities by producing incredibly creative and detailed images. It also exhibits an advanced comprehension of intricate and imaginative instructions by producing intricate and abstract images that flow naturally from the text.
MUM
Developed by Google, MUM (Multitask Unified Model) is engineered to address complex queries by integrating various modalities, such as text and images. It strives to provide nuanced answers by synthesizing information from multiple sources. MUM improves search and information retrieval by offering more detailed and cohesive responses through its ability to merge data from diverse modalities.
Moreover, MUM’s understanding of context across different types of data enhances its ability to deliver precise and relevant results, enriching the user experience in intricate search scenarios.
VisualBERT
By fusing visual characteristics with BERT (Bidirectional Encoder Representations from Transformers), visualBERT is a model that integrates textual and visual data. It is intended for situations where comprehension of both text and visuals is required. Using its multimodal approach to provide greater context and comprehension, VisualBERT is effective in jobs like image captioning and visual question answering.
Florence
Microsoft created the vision-language model Florence, which combines text and image data to carry out operations like image retrieval and visual reasoning. It uses multimodal interactions to improve understanding. Florence is renowned for its capacity to enhance image retrieval and task classification by integrating contextual data from both textual and visual sources.
Florence is an effective tool for intricate visual and textual analysis because of its clever integration of multimodal data. This enables it to produce more accurate and contextually appropriate results.
Addressing the Challenges in Multimodal AI Implementation
Implementing multimodal AI presents several challenges, but there are effective solutions to address these issues. Let’s check out the top challenges of multimodal AI and solutions to overcome those in detail.
Computational Complexity and Scalability
The significant computational resources needed to process massive amounts of multimodal data may hinder real-time processing and scalability.
Solution: Using cloud computing resources in conjunction with other technologies like GPUs and TPUs can improve computational capabilities. Scaling up the processing power also requires distributed computing frameworks and optimized algorithms to effectively handle multimodal AI applications.
Data Integration and Management
One of the main issues in data management and integration is integrating data from many modalities, such as text, photos, and sensor measurements. These data types frequently have different features and formats, making it challenging to synchronize and analyze them effectively.
Solution: Standardizing data formats and creating thorough pretreatment techniques might help ensure a more seamless connection. Accurate and consistent analysis can be ensured by integrating multimodal data streams with the use of data fusion techniques.
Interpreting Multimodal Data
Complex algorithms that can comprehend and correlate many kinds of data are needed to handle the difficulty of interpreting data from various sources.
Solution: Improving interpretation accuracy can be achieved by creating sophisticated machine learning models, such as recurrent neural networks (RNNs) for sequential data and convolutional neural networks (CNNs) for picture data.
Model performance can be further enhanced by incorporating strategies like attention processes and transfer learning.
Data Security and Privacy
Managing sensitive data creates privacy and security issues, especially in industries like finance and healthcare. It is crucial to guarantee safe data transfer and storage.
Solution: Sensitive data can be protected by putting in place robust encryption mechanisms, access controls, and data anonymization strategies. Adhering to regulatory compliances and data privacy standards is also essential to ensure the security of multimodal AI applications.
The Future Trajectory of Multimodal AI
The future of multimodal AI is expected to witness transformative advancements as it integrates diverse data types, such as text, speech, and images, into a unified framework. Popular AI platforms like Gemini illustrate this progress by being able to interpret, explain, and generate high-quality code in major programming languages such as Python, Java, C++, and Go. This capability frees developers to focus on creating more complex and feature-rich applications.
As multimodal AI evolves, it will enhance user interfaces, decision-making, and immersive experiences in areas like virtual reality. This shift moves AI closer to functioning as a knowledgeable and expert assistant rather than just intelligent software, offering more nuanced and contextually aware support. However, these advancements will come with challenges, including managing data integration and maintaining ethical standards.
Also Read: Top AI Trends 2024: Key Developments to Watch
Embark on the Multimodal AI Revolution with Appinventiv’s Expertise
The rise of multimodal AI applications is pivotal as they enable systems to process and integrate diverse types of data into a cohesive understanding. This advancement significantly enhances the sophistication and accuracy of AI interactions, making multimodal AI more intuitive and effective. As this technology continues to evolve, it opens new possibilities for creating highly responsive and context-aware solutions across various industries.
Initiating your journey of building multimodal AI applications with an AI development company like Appinventiv offers an exceptional opportunity to harness this transformative technology. Appinventiv’s expertise in delivering innovative custom AI solutions is evident through its work on projects like JobGet, Mudra, Vyrb, DiabeticU, and YouCOMM.
For JobGet, Appinventiv developed a platform that revolutionizes job searching for blue-collar workers by enabling real-time messaging and scheduling.
Through our dedicated efforts, JobGet has secured $52 million in Series B funding successfully. The app has achieved notable success and now ranks as a top performer in the app stores.
With YouCOMM, we created a multi-request healthcare mobile app featuring a customizable patient messaging system that supports voice and gesture-based interactions with nurses. Our efforts led to the solution being adopted by more than 5 hospital chains.
These successful implementations highlight Appinventiv’s capability to drive impactful AI solutions across various domains, making us your trusted partner for your multimodal AI initiatives. As a trusted and reputed AI development services company, we specialize in creating robust multimodal AI applications that seamlessly integrate various data types, enhancing functionality and user experience across multiple dimensions. Our expertise covers a wide range of multimodal capabilities, including:
- Document-Based Question Answering: Delivering precise answers by analyzing and interpreting textual documents.
- Visual Scene Description: Creating detailed textual descriptions of visual content and scenes.
- Descriptive Video Creation: Generating comprehensive narrations for videos to improve accessibility.
- Visual Question Answering: Answering questions based on the analysis of image content.
- Descriptive Image Generation: Creating images from textual descriptions or other input data.
- Graph-Based Learning: Utilizing graph structures to reveal relationships and insights within complex datasets.
Connect with our experts today and kickstart your multimodal AI journey with Appinventiv.
FAQs
Q. What are the advantages of multimodal AI for a business?
A. Here are some of the top multimodal AI benefits for businesses:
- Versatility in real-world applications
- Enhanced performance across complex tasks
- In-depth understanding of context
- Improved accuracy with diverse data types
- Greater contextual awareness
- Enhanced user experience
- Advanced problem-solving capabilities
- Scalability across various industries
Q. How does multimodal AI work?
A. Multimodal AI works by utilizing specialized models and techniques to manage different types of data. The systems first preprocess and extract features from each modality individually. Next, they employ fusion mechanisms to combine these features into a unified representation. This integrated data is then analyzed to carry out tasks such as classification, generation, or reasoning, effectively leveraging the combined insights from the various modalities.
Some of the crucial components of multimodal AI include feature extraction, data fusion, modality alignment, integration models, and application-specific processing.
Q. How is multimodal AI different from traditional AI?
A. Multimodal AI differs from traditional AI approaches due to its capability to handle and integrate multiple data types simultaneously, including text, images, audio, and video. In contrast, traditional AI models, often known as unimodal, usually focus on a single type of data, such as text or images.
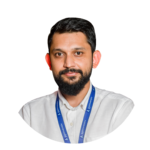
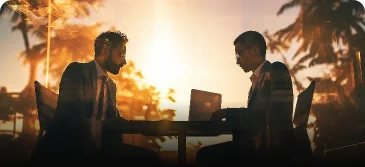
- In just 2 mins you will get a response
- Your idea is 100% protected by our Non Disclosure Agreement.
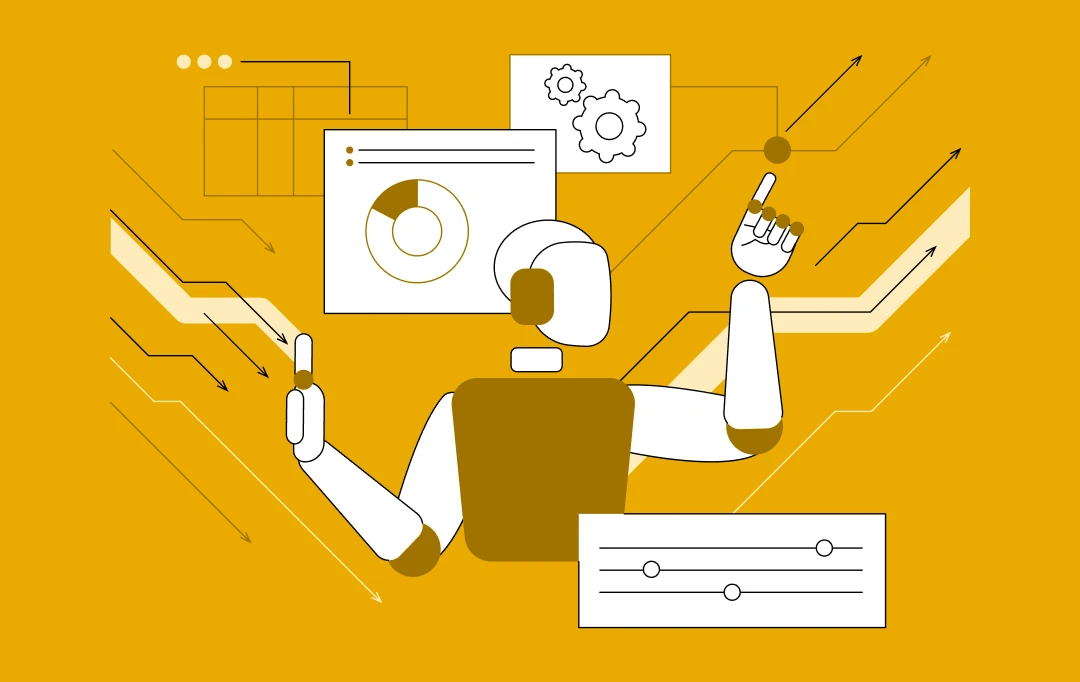
How to Integrate AI with EHR/EMR Systems for Streamlined Healthcare Operations
Key takeaways: Not adopting AI in EHR/EMR today risks falling behind. AI streamlines EHR workflows, cutting clinical and admin delays. Real-world hospitals are already seeing faster, smarter care with AI. Seamless AI integration needs system checks, data prep, and training. AI in EHR tackles real challenges like bias, cost, and compliance. Custom AI integration costs…
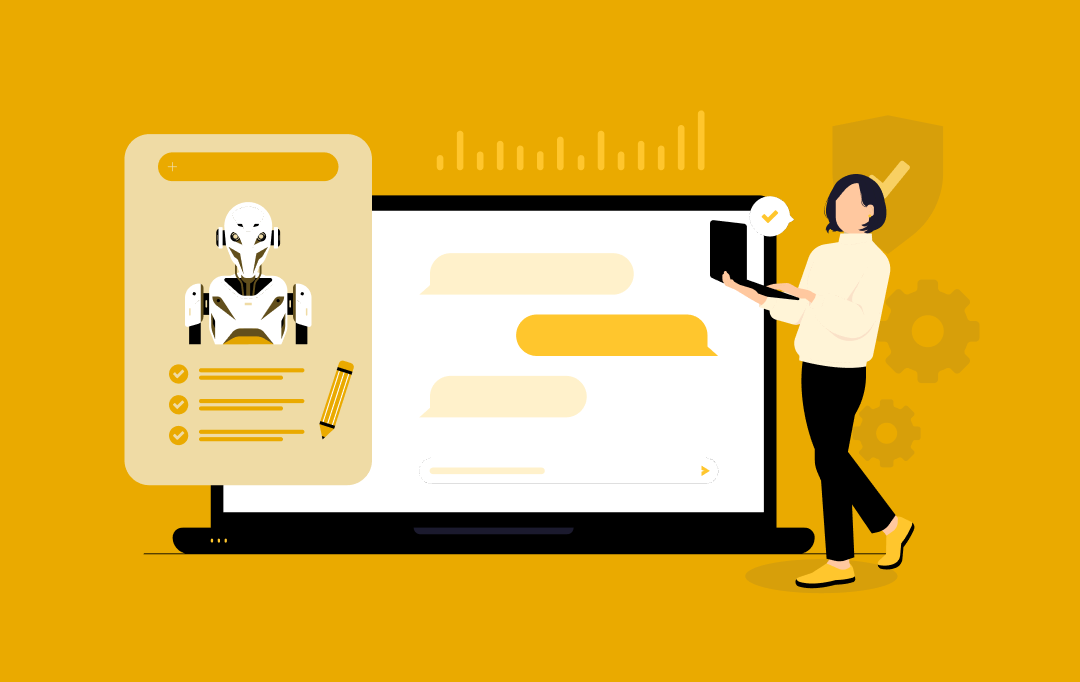
AI Agent-Driven UIs: Replacing App Menus and Buttons
Key takeaways: Challenge: Static menus and buttons also become outdated because people are frustrated by limited, multi-stage navigation. Solution: The AI Agent interacts with the user through a friendly UI that does not require cludgy menus but conversational and context-sensitive navigation. The Way AI Agents Work: AI agents establish a basis of natural language understanding,…
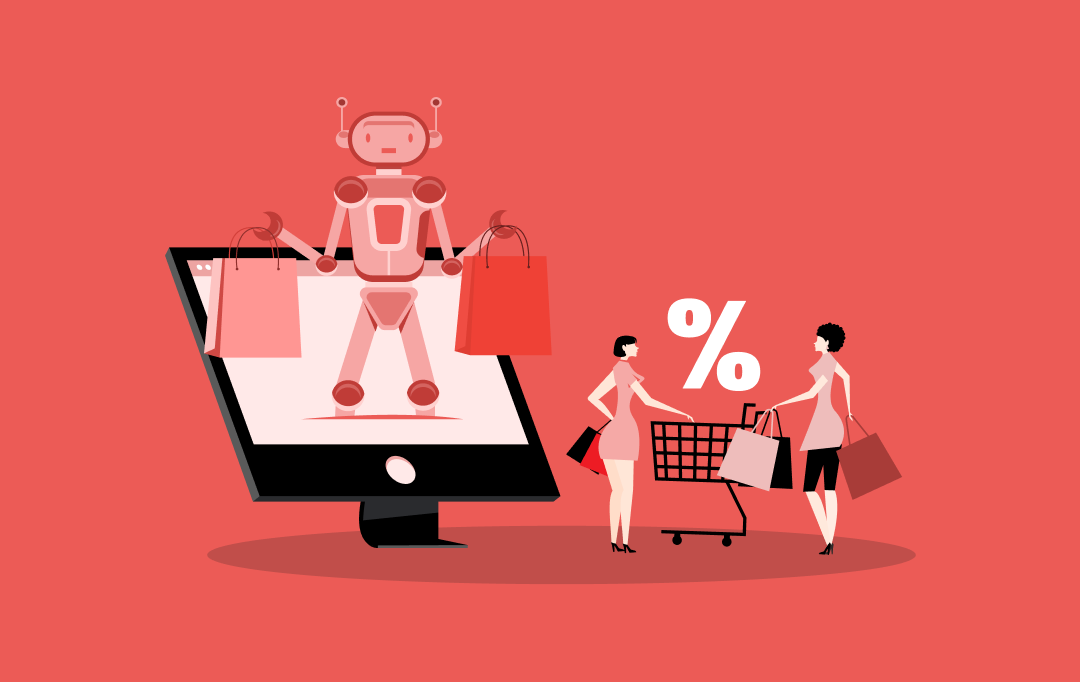
10 Use Cases and Benefits of How AI Agents Are Revolutionizing the Retail Industry
Key takeaways: AI agents in the retail industry are revolutionizing businesses by enhancing customer experience and streamlining operations. From personalized recommendations to predictive analytics, AI offers tangible benefits like increased conversions and reduced costs. AI adoption can lead to scalable growth and a competitive edge in the retail industry. Retailers must consider data strategies, talent…