- Understanding the Importance of Healthcare AI Solutions for Enterprises
- Navigating the AI Challenges in Healthcare
- Integration of AI with Existing Systems
- Data Quality and Accessibility
- Data Security and Privacy
- Bias and Discrimination
- Regulatory Frameworks and Compliances
- Scalability and Upgrades
- Development and Deployment Costs
- Patient Trust and Perception
- Acceptance and Adoption of AI in Healthcare
- Technical Complexity and Skill Gaps
- Real-World Examples of Healthcare Organizations Leveraging AI Successfully
- Google's AMIE: Redefining Clinical Conversations with AI
- Mayo Clinic’s OPUS: Transforming Ophthalmic Care with AI
- Cleveland Clinic’s AI-Powered Patient Flow Optimization
- Partner with Appinventiv to Leverage AI’s Full Potential in Healthcare
- FAQs
Artificial intelligence (AI) in healthcare has a virtually limitless potential to transform traditional healthcare systems, offering breakthroughs like early cancer detection, personalized medicine, and operational streamlining. For instance:
- AI-powered diagnostic tools like Google’s DeepMind can detect over 50 eye diseases with 94% accuracy, revolutionizing ophthalmology. (Moorfields)
- A 2024 study by researchers at the National Cancer Institute (NCI) reveals how AI in oncology can analyze data from individual tumor cells to predict a patient’s response to specific cancer drug treatments.
- Another study by Google Health showcased the effectiveness of their AI model in breast cancer detection. This AI system matched the diagnostic accuracy of trained radiologists and reduced false positives and negatives, surpassing the performance of six expert radiologists in mammogram analysis.
- Generative AI in healthcare enables the creation of synthetic datasets to train models without compromising patient privacy.
However, the journey to these advancements is not a bed of roses.
The evolution is fraught with several ethical, legal, and operational challenges that necessitate careful navigation.
For example, while AI can significantly improve diagnostic accuracy, models often encounter biases due to unrepresentative training data, leading to potential misdiagnoses in underserved populations. Moreover, compliance with regulations like HIPAA and GDPR adds layers of complexity, slowing innovation.
These hurdles highlight the need for robust success strategies to navigate AI challenges in healthcare while ensuring compliance with stringent healthcare standards. By addressing these barriers, organizations can unlock AI’s full potential to improve patient care, enhance efficiency, and drive digital transformation in healthcare.
Let’s explore how AI is revolutionizing healthcare and addressing some of its critical challenges with strategic solutions.
Understanding the Importance of Healthcare AI Solutions for Enterprises
AI’s role in healthcare is immeasurable and undeniable. According to Grand View Research, the global AI market in healthcare was valued at $19.27 billion in 2023 and is projected to grow at a CAGR of 38.5% from 2024 to 2030. One prime factor driving this market growth is AI’s potential to make the sector more personalized, predictive, preventative, and interactive.
Enterprises adopting AI in healthcare are witnessing profound improvements in radiology, disease diagnosis, drug discovery, predictive analytics, personalized treatment planning, and virtual assistance.
- Enhanced diagnostics, driven by AI’s ability to analyze vast amounts of data, leads to more accurate and timely disease detection. For example, AI-powered tools have demonstrated remarkable success in diagnosing conditions like breast and skin cancer at early stages, often outperforming human doctors in accuracy.
- Precision medicine is another promising application, as AI can analyze individual patient data to recommend tailored treatments that increase the likelihood of successful outcomes. This personalized approach ensures patients receive the most effective care based on their unique genetic makeup and health history.
- AI also impacts mental health, where AI-driven applications are helping diagnose and manage mental health disorders more effectively. AI Chatbots like Google’s AMIE and virtual assistants offer mental health support through cognitive-behavioral therapy (CBT) techniques and provide real-time monitoring for conditions like depression and anxiety.
In addition to clinical benefits, AI is streamlining administrative workflows in healthcare, reducing the burden of repetitive tasks like data entry and scheduling. This allows healthcare professionals to focus more on patient care and decision-making.
Moreover, predictive analytics in healthcare improve patient outcomes by anticipating complications and personalizing care plans. For instance, predictive algorithms have been shown to identify high-risk patients earlier, allowing for timely interventions that can save lives and reduce hospital readmissions.
AI will continue its transformative role in healthcare, becoming a fully mature medical assistant.
However, while AI’s role in health care is vast and growing, its implementation is challenging. Let’s explore some of the key challenges of technology in healthcare that organizations face as they strive to integrate AI into their medical settings.
Navigating the AI Challenges in Healthcare
Implementing AI in healthcare comes with its own set of challenges that need to be navigated carefully. One requires technical and strategic solutions to overcome the ethical and privacy challenges of AI in healthcare. Let’s dive deeper into some of the negative impacts of AI in healthcare that organizations must overcome.
Integration of AI with Existing Systems
Challenge
Implementing AI in healthcare workflows and IT infrastructure remains a major hurdle. Indeed, integrating AI into healthcare systems, such as electronic health records (EHRs) and imaging technologies, smoothly without causing disruptions and inefficiencies to the existing system is a real challenge. Also, many AI tools fail to align with clinicians’ day-to-day practices, leading to underutilization.
Success Strategies
To ensure seamless integration of AI in healthcare, businesses should facilitate collaboration between clinical, IT, and AI teams. This involves thoroughly assessing current systems, identifying integration points, and adopting detailed implementation strategies. Furthermore, leveraging interoperability standards and open APIs is crucial to enhancing the compatibility of AI tools within existing technological infrastructures.
Data Quality and Accessibility
Challenge
AI’s success hinges on high-quality, accessible data. Healthcare data is projected to grow at a CAGR of 36% by 2025, driven by technological advancements like IoT, EHRs, and AI-powered analytics. This explosive growth in healthcare data poses great opportunities but simultaneously introduces challenges for real-world AI implementation.
The sheer volume of data doesn’t inherently ensure its accessibility and quality. Healthcare data is often siloed across disparate systems, leading to inconsistencies, inaccuracies, and gaps.
These inaccuracies can adversely affect the reliability of AI models, leading to inaccurate insights. For instance, missing or incorrect patient information can distort predictive models, impacting outcomes.
Success Strategies
Addressing this requires interoperable frameworks like HL7 FHIR standards that enable seamless data exchange, ensuring AI models have the complete datasets necessary for reliable predictions.
Appinventiv developed Health-e-People – a healthcare app that helps users seamlessly manage their health data. It allows users to access scattered data from their medical apps and devices while enabling easy communication with healthcare professionals. This platform turns the complex healthcare ecosystem into an interconnected community, making it easier for users to take charge of their health with the support of medical experts and loved ones.
Data Security and Privacy
Challenge
AI systems process sensitive patient data, making them a prime target for cyberattacks. In a striking example, the US Department of Health and Human Services confirmed on October 2024 that the UnitedHealth data breach impacted 100 million individuals, marking it as the largest healthcare data exposure in US history.
This breach highlights the growing risks associated with cybersecurity incidents in healthcare, especially as AI systems become more integrated into patient care. For instance, secure data transfer is critical not only for AI-driven diagnostics but also for Medical Courier Apps, which ensure the safe and timely delivery of sensitive medical specimens and records between healthcare facilities. 37% of Americans believe using AI in healthcare can worsen the security of patients’ records.
Success Strategies
To address security and privacy concerns with AI in healthcare, organizations must ensure that their systems and patient data are protected from unauthorized access or malicious attacks.
They can employ a combination of data security measures, including encryption technology, authentication protocols, federated learning techniques, and data breach prevention. Beyond stringent security measures, healthcare providers must adhere to regulatory compliance such as HIPAA and GDPR.
Bias and Discrimination
Challenge
AI models often inherit biases present in their training data. A study revealed that a skin cancer diagnostic algorithm demonstrated high accuracy for patients with lighter skin tones but significantly underperformed for those with darker skin, highlighting biases in training datasets.
Similarly, research emphasizes the importance of achieving gender balance in medical imaging datasets for AI systems used in computer-aided diagnosis. Failing to address this balance consistently decreases diagnostic accuracy for underrepresented genders.
Success Strategies
Thus, to achieve accuracy and remove discrimination based on gender, skin tone, etc., it is essential to identify and mitigate these biases when developing and deploying AI algorithms. Healthcare providers require diverse datasets for algorithm training, continuous monitoring, and fairness assessments during development.
Regulatory Frameworks and Compliances
Challenge
One major hurdle in implementing AI in healthcare is navigating complex regulatory and ethical considerations. Implementing AI in healthcare requires navigating stringent regulatory and moral challenges.
Global frameworks such as the FDA’s regulatory approach for Software as a Medical Device (SaMD) ensure AI tools undergo rigorous premarket reviews and postmarket monitoring to maintain safety and effectiveness. Similar guidelines from bodies like the EMA and Japan’s PMDA, alongside HIPPA and GDPR’s strict data protection standards, highlight the global emphasis on compliance.
Success Strategies
Healthcare organizations can mitigate these challenges by developing governance frameworks, working closely with regulatory bodies and ethics committees, and employing robust testing and validation mechanisms. Clear communication about AI’s role and impact on decision-making reinforces accountability and supports the ethical deployment of these technologies.
Scalability and Upgrades
Challenge
With ceaseless AI innovation in healthcare and a rapid surge in data volumes, scalability and upgrades of AI medical tools have become integrally essential. A static AI model that fails to keep pace with the emerging AI trends can soon be obsolete and irrelevant to medical use.
Scaling AI solutions across healthcare networks requires extensive infrastructure investments and technical expertise. Deploying an AI diagnostic tool across multiple hospital branches demands uniform data formats, hardware compatibility, and ongoing maintenance.
Moreover, it upgrades risk system downtime or retrains models on newer datasets. Solutions like cloud-based AI platforms can offer flexibility and reduce infrastructure costs while ensuring smoother updates.
Success Strategies
Healthcare organizations must adopt a continuous learning approach, regularly retraining AI models with fresh data and insights to maintain their relevance and accuracy. Implementing rigorous monitoring mechanisms allows for the timely identification of areas for improvement and quick fine-tuning, ensuring sustained efficiency and innovation in healthcare solutions.
Also read: 10 Use Cases and Benefits of AI in Medical Billing
Development and Deployment Costs
Challenge
Appinventiv developed DiabeticU, a cutting-edge diabetes management app that utilizes AI to provide real-time responses to user queries, connect individuals with diabetes professionals, and facilitate a supportive community.
By empowering users to take control of their well-being, the app promotes a healthier lifestyle and demonstrates the transformative potential of AI in healthcare.
However, building an AI-driven healthcare app at such an advanced level demands substantial investments in advanced technology infrastructure, rigorous regulatory compliance, and comprehensive staff training; these high development and deployment costs can often deter smaller organizations from adopting AI.
Success Strategies
Smaller hospitals and clinics can overcome these financial barriers by partnering with a reputed healthcare software development company that uses open-source AI frameworks like TensorFlow and PyTorch to reduce the implementation cost and avoid wasteful steps.
Patient Trust and Perception
Challenge
Patient trust is a major barrier to the widespread adoption of AI in healthcare. Many patients remain skeptical about the accuracy and transparency of AI-powered decisions, particularly when they feel a machine might replace human judgment in critical areas like diagnostics or treatment planning.
This skepticism can stem from concerns over data privacy, algorithmic bias, or the perception that AI lacks the empathy and nuanced understanding that human clinicians offer.
A recent study found that 57% of individuals expressed concern about AI’s potential impact on the personal connection between patients and healthcare providers. They believe AI-driven tools, such as those used for diagnosing diseases or recommending treatments, could harm the patient-provider relationship.
Success Strategies
To address these limitations of AI in healthcare, healthcare providers should emphasize transparency in AI processes, ensuring that patients understand how decisions are made and how AI complements rather than replaces human care.
Acceptance and Adoption of AI in Healthcare
Challenge
Clinician resistance remains a major stumbling block when implementing AI in healthcare. A 2023 report published in the International Journal of Medical Informatics highlights that many medical professionals are hesitant to embrace AI due to the demand for acquiring new skills and managing more complex responsibilities.
This reluctance highlights the urgent need for targeted training programs and clear communication about AI’s potential to enhance, rather than replace, clinical expertise.
Success Strategies
To navigate the acceptance and adoption challenges of artificial intelligence in healthcare, businesses can demonstrate the direct benefits of AI that closely match human activities—like virtual assistants for appointment scheduling, predictive analytics for patient monitoring, and so on. Clear communication and transparency in AI’s role in decision-making can build trust and encourage adoption among medical staff.
Technical Complexity and Skill Gaps
Challenge
Developing and deploying AI technology in healthcare requires interdisciplinary technical expertise in healthcare machine learning, data science, medicine, and IT. However, there is a significant shortage of professionals with these combined skills to implement and maintain AI systems in healthcare settings. Healthcare organizations often lack in-house AI experts who can efficiently manage end-to-end implementation of AI systems.
Success Strategies
To bridge this skill gap, healthcare organizations must upskill in-house staff and incorporate external expertise, such as consulting with AI development services providers and hiring a CAIO.
Real-World Examples of Healthcare Organizations Leveraging AI Successfully
Healthcare organizations increasingly leverage AI to improve patient care, streamline operations, and enhance diagnostic accuracy. Let’s explore a few real-world examples where some renowned enterprises are successfully navigating the AI challenges in healthcare:
Google’s AMIE: Redefining Clinical Conversations with AI
Google’s Articulate Medical Intelligence Explorer (AMIE), a large language model (LLM) represents a groundbreaking advancement in healthcare AI. This AI-driven chatbot is meticulously trained on real-world medical datasets, enabling it to exhibit advanced levels of medical reasoning, clinical summarization, and conversational capabilities. Unlike many conventional chatbots, AMIE’s diagnostic accuracy and conversation quality keep pace with human doctors, strengthening Google’s position in the AI race.
Mayo Clinic’s OPUS: Transforming Ophthalmic Care with AI
Mayo Clinic’s OPUS (Ophthalmic Precision Using Sub-millimeter Analysis) leverages advanced AI to enhance ophthalmic diagnostics and treatment. Using cutting-edge imaging and machine learning, OPUS delivers precise, personalized care for conditions like retinal diseases. This innovation aligns with Mayo Clinic’s broader AI strategy to revolutionize early disease detection and improve patient outcomes across diverse populations.
Cleveland Clinic’s AI-Powered Patient Flow Optimization
Cleveland Clinic has emerged as a leader in leveraging AI to address healthcare challenges, particularly data quality and operational efficiency. By implementing AI-driven solutions to analyze patient flow and optimize scheduling, the clinic has successfully reduced wait times by 10%, enhancing the patient experience and operational effectiveness.
Partner with Appinventiv to Leverage AI’s Full Potential in Healthcare
Implementing AI in healthcare is not without its challenges. However, by partnering with Appinventiv, you can efficiently address AI challenges in healthcare and leverage its limitless potential.
Our 9+ years of experience in delivering healthcare software development services positions us as the ideal partner to help you overcome the risks of AI in healthcare. With a proven track record of delivering 3000+ successful projects, including Soniphi, Health-e-People, YouComm, DiabeticU, etc., we are committed to overcoming AI in healthcare challenges while revolutionizing patient care and optimizing healthcare operations.
Let’s collaborate to create tailored healthcare AI solutions for enterprises that improve patient outcomes, ensure compliance, and drive innovation. Together, we can shape the future of healthcare for a smarter, healthier tomorrow.
FAQs
Q. What is the cost of implementing AI in healthcare?
A. The cost of implementing AI in healthcare can vary significantly depending on various factors, such as project complexity, the level of problems with AI in healthcare, the chosen technology stack, and the expertise of the development team.
On average, developing and deploying healthcare AI solutions for enterprises costs between $30,000 and $300,000 or more.
Share your project idea with our skilled AI developers to get a more precise estimate for AI implementation costs in healthcare.
Q. What are the key challenges of AI implementation in healthcare?
A. Some of the most common AI challenges in healthcare are:
- Data Quality and Accessibility
- Data Security and Privacy
- Bias and discrimination in AI algorithms
- Regulatory Frameworks and Compliances
- Integration of AI with Existing Systems
- Scalability and Upgrades
- Development and Deployment Cost
- Patient Trust and Perception
- Acceptance and Adoption of AI In Healthcare
- Technical Complexity And Skill Gaps
To gain an in-depth understanding of these AI in healthcare challenges, please refer to the above blog.
Q. How is AI being used to solve problems in healthcare?
A. AI can be used in various ways to solve problems in healthcare:
- Enhanced Diagnostics: AI revolutionizes early disease detection with applications in radiology and pathology.
- Personalized Medicine: AI tools analyze the sheer volume of medical data to offer personalized treatment plans.
- Operational Efficiency: AI optimizes appointment scheduling, resource allocation, and workflow management.
- Virtual Health Assistants: AI-driven apps help patients with tasks like appointment scheduling, medication reminders, and symptom tracking, improving patient engagement and satisfaction.
Q. What strategies can healthcare enterprises adopt to overcome AI challenges?
A. Enterprises can adopt the following strategies to overcome the issues with AI in healthcare:
- They should facilitate smooth collaboration among clinical, IT, and AI development teams to ensure that the AI tools meet the practical needs of healthcare professionals.
- They can further invest in staff training to demonstrate AI’s potential to enhance medical expertise and overcome clinician resistance.
- Healthcare enterprises must implement robust data protocols to ensure that AI systems comply with regulatory standards such as HIPAA. This helps mitigate security risks and builds patient trust.
- AI technology in healthcare should be developed with interoperability in mind to improve data sharing and integration across systems.
Q. What are the risks associated with AI in patient care?
A. AI has shown great promise in transforming healthcare, but its implementation in patient care comes with a set of risks that need careful consideration. Below are some of the most common risks of AI in healthcare:
- Inaccurate Diagnoses and Treatment Recommendations
- Lack of Human Touch and Empathy
- Ethical Issues and Bias
- Overreliance on AI
- Data Privacy and Security Concerns
- Regulatory and Compliance Issues
- Ethical Issues and Bias
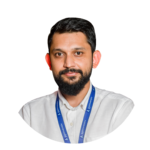
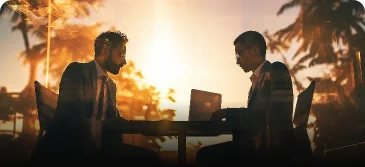
- In just 2 mins you will get a response
- Your idea is 100% protected by our Non Disclosure Agreement.
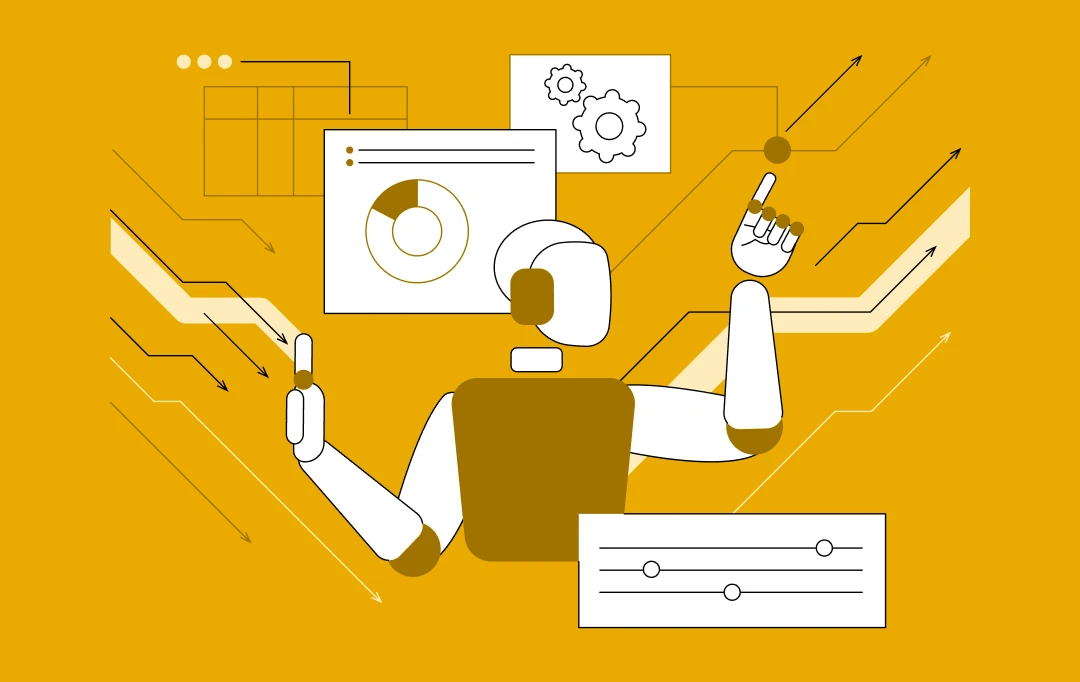
How to Integrate AI with EHR/EMR Systems for Streamlined Healthcare Operations
Key takeaways: Not adopting AI in EHR/EMR today risks falling behind. AI streamlines EHR workflows, cutting clinical and admin delays. Real-world hospitals are already seeing faster, smarter care with AI. Seamless AI integration needs system checks, data prep, and training. AI in EHR tackles real challenges like bias, cost, and compliance. Custom AI integration costs…
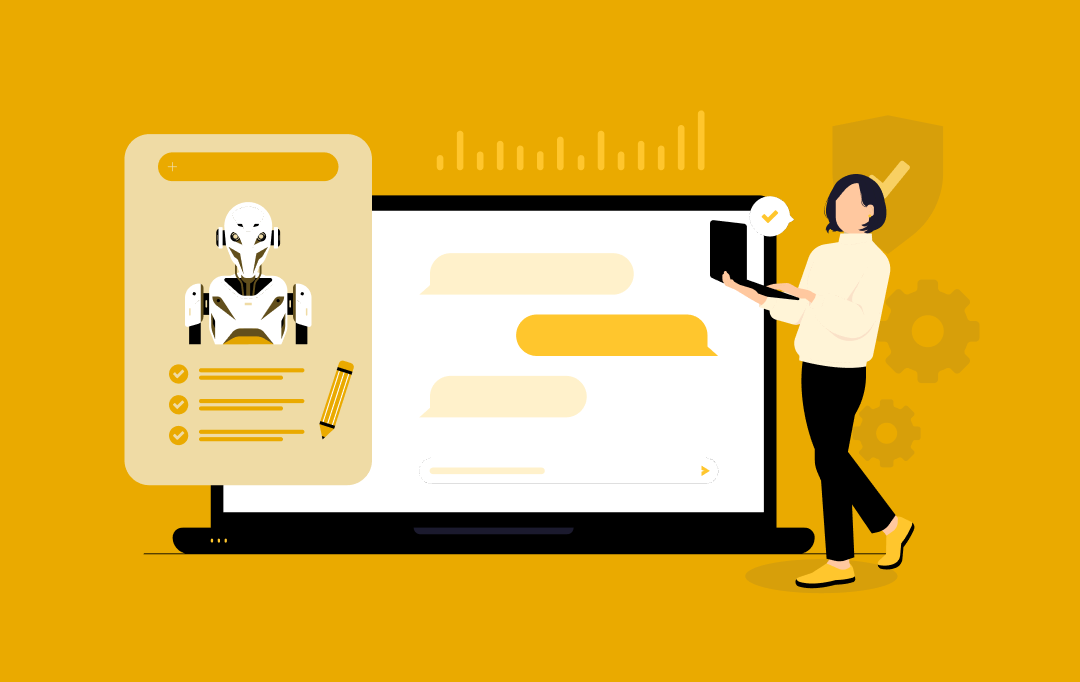
AI Agent-Driven UIs: Replacing App Menus and Buttons
Key takeaways: Challenge: Static menus and buttons also become outdated because people are frustrated by limited, multi-stage navigation. Solution: The AI Agent interacts with the user through a friendly UI that does not require cludgy menus but conversational and context-sensitive navigation. The Way AI Agents Work: AI agents establish a basis of natural language understanding,…
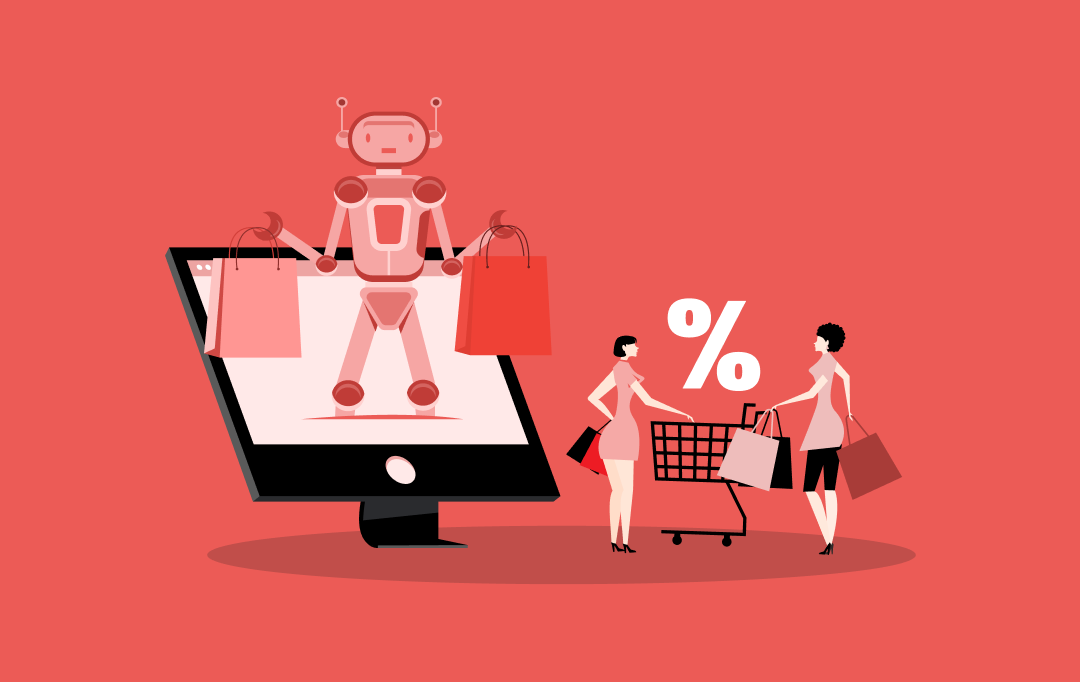
10 Use Cases and Benefits of How AI Agents Are Revolutionizing the Retail Industry
Key takeaways: AI agents in the retail industry are revolutionizing businesses by enhancing customer experience and streamlining operations. From personalized recommendations to predictive analytics, AI offers tangible benefits like increased conversions and reduced costs. AI adoption can lead to scalable growth and a competitive edge in the retail industry. Retailers must consider data strategies, talent…