- How is Machine Learning Used in Healthcare?
- Top Applications of Machine Learning in Healthcare
- Personalized Treatment
- Fraud Detection and Prevention
- Detecting Diseases in Early Stages
- Robot-Assisted Surgery
- Analyzing Errors in Prescriptions
- Assisting in Clinical Research and Trials
- Drug Discovery and Development
- Automating Image Diagnosis
- What are the Benefits of ML in Healthcare?
- Faster Data Collection
- Cost-Efficient Process
- Predictive Analytics
- Patient Education and Engagement
- Challenges of Adopting Machine Learning in the Healthcare Industry
- Patient Safety
- Biases in Dataset
- Privacy Concerns
- The Future of Machine Learning for Healthcare
- How Can Appinventiv Help You Adopt ML in Healthcare?
- FAQs
The healthcare industry has long been an early adopter of technological advancements and the COVID-19 pandemic has further pushed the sector to actively adopt modern technologies.
Technologies like artificial intelligence and machine learning are driving massive innovation and improvement in the healthcare industry, including the advancements in clinical operations, drug development and delivery, surgery, medical assistance, and data management.
According to Statista, AI in the global healthcare market was worth over $11 billion in 2021 and is estimated to reach $187 billion by 2030, increasing at a CAGR of 37% from 2022 to 2030.
So what are the factors driving this massive growth of AI and machine learning in healthcare? What are the use cases and applications of machine learning for healthcare? What does adopting machine learning technology mean for healthcare businesses?
Let us find out answers to all such questions in the blog below.
Related Article: How is Artificial Intelligence Transforming the Healthcare Industry
How is Machine Learning Used in Healthcare?
Machine learning is driving massive improvement in the healthcare industry. It is expediting advancements in areas including, clinical operations, drug discovery, and surgery. Most importantly, machine learning in healthcare can detect early indicators of a disease more accurately, helping reduce the number of admissions and readmissions in hospitals and clinics.
The technology examines satellite data, social media reports, news, and even video sources to determine whether the ailment is controllable or will become lethal. Machine learning for healthcare opens a world of endless possibilities, freeing up medical professionals to find the best treatment plan and patient care rather than managing data.
Top Applications of Machine Learning in Healthcare
From maximizing hospital efficiency to making an accurate diagnosis, the role of machine learning in healthcare has proven to be a boon for medical professionals and patients alike. Here are some major machine learning applications in healthcare, redefining the industry and creating a better future for patients.
Personalized Treatment
Offering personalized treatments is one of the key use cases of machine learning in healthcare. It allows healthcare organizations to deliver personalized care by analyzing patients’ medical history, symptoms, and tests. Doctors can use ML in medicine and healthcare to develop customized treatments and prescribe medicines that target specific diseases in each patient.
Additionally, machine learning for healthcare can assist doctors in finding out if the patient is ready for necessary changes in medication. It helps induce the right treatment from the beginning.
Fraud Detection and Prevention
According to NHCAA (National Health Care Anti-Fraud Association), healthcare frauds cause billions of dollars of financial loss yearly, which is approximately 3 to 10% of annual healthcare expenditures. This converts into around $300 billion loss annually.
Using machine learning for healthcare, businesses can detect invalid insurance claims before they are paid for and speed up approval, processing, and payment of valid ones. Besides detecting insurance fraud, the machine learning application in healthcare can also prevent the theft of patient data.
For example, a leading healthcare organization, Harvard Pilgrim Health, embraces AI and ML technologies to root out healthcare fraud. It leverages ML-based fraud detection systems to identify claims and detect suspicious behavior.
Detecting Diseases in Early Stages
Healthcare providers need to detect many diseases in the early stages to identify the best treatment plan and help patients secure a good way of living. ML provides better assistance to doctors in the early detection of diseases by comparing new data with old data on a particular ailment. Based on data comparison, doctors can make informed decisions and design the best treatment plans accordingly.
Robot-Assisted Surgery
ML-powered surgical robots have revolutionized surgical processes in terms of accuracy and speed. These systems can perform complicated surgical procedures with reduced risk of blood loss, side effects, and pain. Additionally, post-surgery recovery is much faster and easier.
Maastricht University Medical Center’s surgical robot uses an ML-powered surgical robot to suture small blood vessels; no thicker than 0.03 millimeters.
Read here to know how the future of work will be molded by robots.
Analyzing Errors in Prescriptions
Prescription errors are one of the most fatal medical mistakes that lead to patient deaths in many instances. It involves prescribing the wrong drug or dose or failing to account for drug interactions. In the US alone, around 7,000 to 9,000 patients die annually because of prescription errors.
To reduce such medical mistakes, healthcare machine learning solutions can be a savior.
Machine learning models for healthcare analyze historic EHR data and compare new prescriptions against it. Those prescriptions that deviate from typical patterns get flagged so that doctors can review and adjust them.
For instance, Brigham and Women’s Hospital uses an ML-powered system to pinpoint prescription errors. Over a year, the system identified 10,668 potential errors, and 79% of them were clinically valuable, so the hospital managed to save $1.3 million in healthcare-related costs. Along with cost savings, an ML-powered error detection system increases the quality of care by preventing drug overdosing and health risks.
Also Read: EHR Optimization Guide to Make Your Healthcare Offering Efficient
Assisting in Clinical Research and Trials
Clinical research and trials are costly and lengthy processes that ensure the safety and effectiveness of medical solutions. However, there are cases when the solution needs to be released quickly – like the vaccines for COVID-19.
Machine learning for healthcare can shorten this process. These algorithms can help determine the best sample for the trial, gather more data points, analyze the ongoing data from the trial participants, and reduce data-based errors.
Drug Discovery and Development
It is one of the main benefits of machine learning in healthcare. ML can discover new drugs that offer great economic value for pharmaceuticals, hospitals, and new treatment avenues for patients. It also makes the process of drug creation faster and extremely cost-effective.
For example, Atomwise is a pharmaceutical company that uses supercomputers, which roots out therapy from molecular structure databases. In 2015, Atomwise used its deep learning technology to discover the current market medicines that could be redesigned for treating the Ebola virus. They successfully found two drugs that could help reduce the risks of the epidemic.
The analysis that would have taken several years happened in one day through Atomwise ML-based technology.
Also Read: How digital transformation is reshaping the healthcare industry?
Automating Image Diagnosis
Hospitals and clinics use ML to recognize abnormalities in medical images, such as MRI or radiology scans that are managed through a radiology information system. Image recognition assists doctors in diagnosing liver and kidney infections, identifying tumors, improving cancer prognosis, and more.
The best example of ML-powered visual perception is the tool utilized by the UVA University Hospital. By harnessing the capabilities of ML algorithms, the tool analyzes biopsy images of children to differentiate between celiac disease and environmental enteropathy, doing it as reliably as doctors do.
What are the Benefits of ML in Healthcare?
As you can see, there are a wide range of use cases of machine learning in healthcare. From assisting doctors in detecting diseases even before they occur, researching medicines, designing personalized treatment plans, and saving lives to reducing costs and more, the importance of machine learning in healthcare is widespread. Here’s a list of just some of the major advantages of machine learning in healthcare:
Faster Data Collection
The healthcare industry uses IOT connected medical devices to gather real-time data, which ML can quickly process and adapt to. This is precisely why the US Food and Drug Administration (FDA) has actively integrated AI and ML into medical device software.
Cost-Efficient Process
Machine learning for healthcare helps improve the efficiency and speed of medical services, which can lead to significant cost savings. For example, ML can quickly scan EHRs to manage patient records, schedule appointments, and automate various processes. This type of machine learning application in healthcare can help reduce the time, money, and resources spent on repetitive tasks.
Predictive Analytics
Machine learning in healthcare can help anticipate patient health deterioration, disease progression, and readmission risks, allowing caregivers to intervene proactively and reduce hospital readmissions.
Also read: Predictive Analytics In Healthcare – 10 Use Cases And Real-World Examples
Patient Education and Engagement
ML-driven chatbots and virtual assistants provide patients with accurate medical information, medication reminders and answer patient queries, enhancing patient engagement and adherence to treatment plans.
Challenges of Adopting Machine Learning in the Healthcare Industry
The wide implementation of innovative technologies like AI and ML comes with several challenges. From the lack of quality data to patient safety, several barriers come on the road to successfully adopt healthcare machine learning solutions.
Patient Safety
The decisions machine learning algorithms make completely rely on the data it has been trained on. Unfortunately, medical data is not always as precise and standardized as it needs to be. There are gaps in records, inaccuracies in profiles, and other shortcomings. So if the input data is unreliable or wrong, there is a high chance that the result will also be wrong, which can lead to incorrect treatment of patients, further worsening their condition or even causing death.
Also Read: How data analytics in healthcare is reducing costs?
Biases in Dataset
Machine learning algorithms, in any industry, are trained on large amounts of data. Without proper supervision and training, these algorithms can carry and propagate the biases contained within the dataset. This could lead to sever consequences especially in the healthcare sector.
Privacy Concerns
Another key challenge of implementing ML in healthcare is the amount of data collected that contains sensitive or confidential information. This, in turn, requires additional security measures to be implemented. So, it is crucial to partner with a reputed ML and AI development company that can offer top-notch security options to ensure your customer data is appropriately handled.
You may like reading: Digital Twins In Healthcare – A New Era Of Innovation For Businesses
The Future of Machine Learning for Healthcare
The future of machine learning in healthcare is promising. Despite some challenges, ML helps resolve current healthcare problems and anticipate future hardships, preventing outbreaks worldwide. Furthermore, it assists in enhancing the patient experience, medical practice of clinicians, and pharmaceutical operations.
The technology is accountable for analyzing hundreds of distinct data points, personalizing treatment plans, predicting risks and results, and performing various other tasks. AI and machine learning for healthcare also provides earlier warnings for conditions like seizures or sepsis, which require intensive analysis of highly complex datasets.
In the coming years, professionals can use ML in conjunction with nanotechnology to improve medicine delivery. Machine learning in the healthcare industry can also enable “virtual biopsies” and advance the innovative field of radiomics. ML-powered technologies can enable doctors to minimize the risk during operations by going down to the minutest treatment details. Also, programmed robots could assist doctors in the surgery room in the future.
Healthcare machine learning solutions can be extremely advantageous for any doctor, clinician, researcher, patient. Every day, the technology creates a breakthrough, and with each breakthrough, a new application emerges, solving a genuine problem or creating a milestone. The advancement of machine learning in healthcare is continually rising, and it seems to reach greater heights in the coming years.
How Can Appinventiv Help You Adopt ML in Healthcare?
Use cases of machine learning in healthcare are expanding by leaps and bounds, and the healthcare industry is keeping a close eye on this trend. Accordingly, organizations across the globe are leveraging AI-driven solutions and ML models to improve healthcare delivery.
Interested in empowering your medical operations with the capabilities of AI and ML? Partner with us for next-gen healthcare IT consulting services and machine learning solutions that speed up time-to-value.
With a team of 1200+ software developers and a proven track record of delivering 3000+ successful projects, Appinventiv can be your trusted tech partner to bring your ML in healthcare vision to life.
We have worked on a multitude of healthcare projects over the past, integrating modern technologies including machine learning, IoT, blockchain, and more.
For instance, we developed Soniphi, the first resonant frequencies-based personal wellness system that provides a complete well-being analysis report on your personal healthcare app. Apart from Sophini, we developed Health-e-People, an integrated platform for connecting various health devices and records on one interface for comprehensive healthcare monitoring. The solution also lets users draw predictive analysis from the records and connects the users with caregivers.
So, if you are also looking to leverage the full potential of artificial intelligence and machine learning in healthcare that can cater to your needs in the most tech-friendly manner, get in touch with our experts now.
FAQs
Q. What are the benefits of machine learning in healthcare?
A. By leveraging the power of machine learning, the healthcare industry can improve overall healthcare outcomes. Here are some key advantages of using machine learning in healthcare:
- Accurate diagnosis and personalized treatment
- Improved patient care
- Efficient healthcare operations
- Cost reduction
- Predictive analytics and disease prevention
- Drug discovery and development
- Remote care and constant monitoring
Q. How is machine learning used in healthcare?
A. Machine learning in the healthcare industry is used for various purposes, including disease diagnosis, drug discovery, treatment planning, effective patient care, and so on. In addition, it helps identify patterns, detect anomalies, and predict outcomes, enabling healthcare professionals to make more accurate diagnoses and prescribe more personalized treatment plans, resulting in improved patient care. Furthermore, machine learning in healthcare is used to streamline administrative tasks and enhance operational efficiency.
Q. What is the future of machine learning in the healthcare industry?
A. The future of machine learning in the healthcare industry is transformative. With the ever-increasing advancements in artificial intelligence and the easy availability of healthcare data, machine learning can revolutionize how healthcare professionals conduct research, treat patients, and perform operational tasks, resulting in unprecedentedly enhanced efficiency, increased productivity, and better patient outcomes.
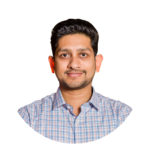
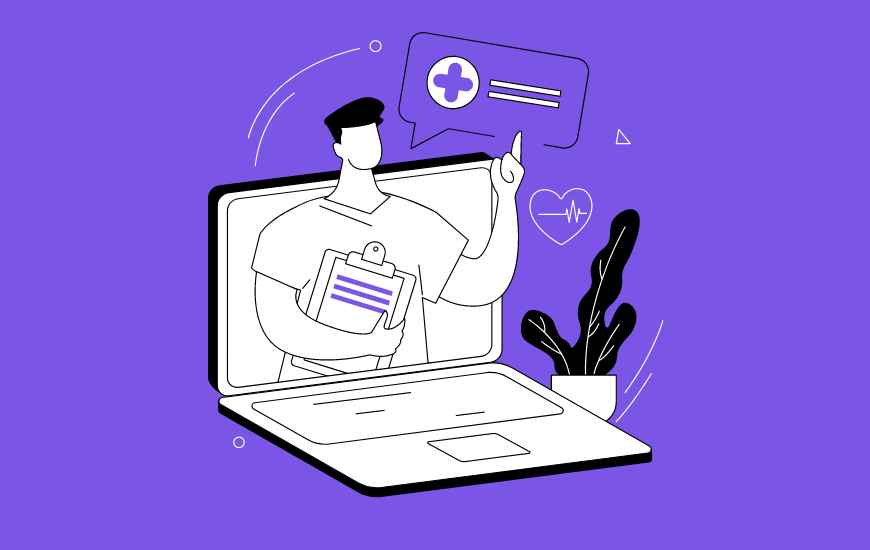
How Generative AI is Reshaping the Healthcare Industry - 10 Applications and Use Cases
Ever pondered about the game-changing impact of generative AI in healthcare? It's truly remarkable how this advanced technology is transforming diagnostics, treatment personalization, and medical research, leading to better outcomes for patients and a more efficient healthcare system overall. Generative AI is catalyzing a profound transformation within the healthcare industry, heralding a new era of…
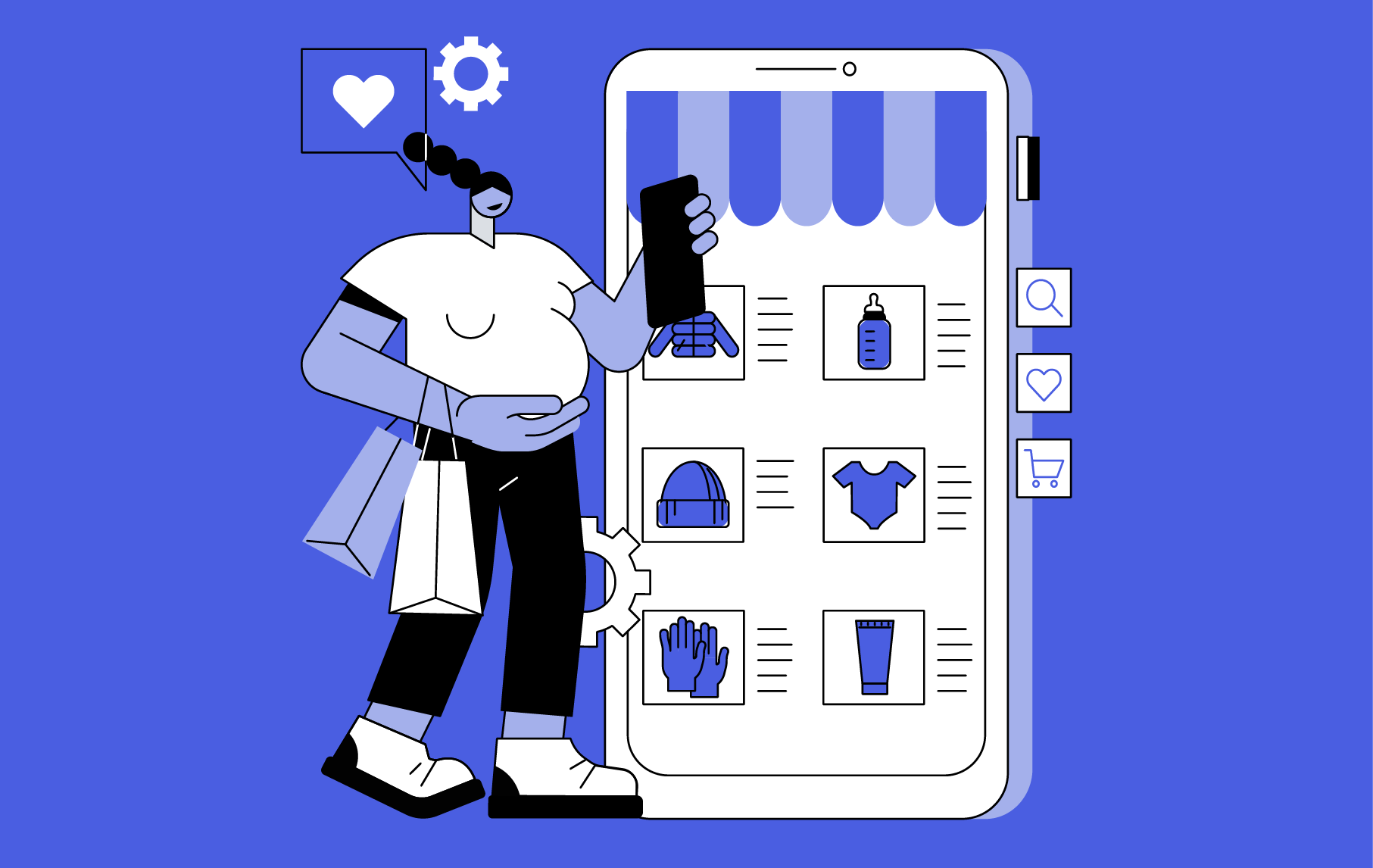
How Much Does It Cost to Build an AI-Based Shopping App Like Target?
eCommerce is a burgeoning phenomenon, redefining the way we buy and sell goods of daily necessities. As the demand for online shopping continues to skyrocket, businesses across industries have understood that the revolution comes via intuitive applications, prompting a reimagining of traditional sales strategies. But shifting from offline brick-and-mortar shops to online stores is that…
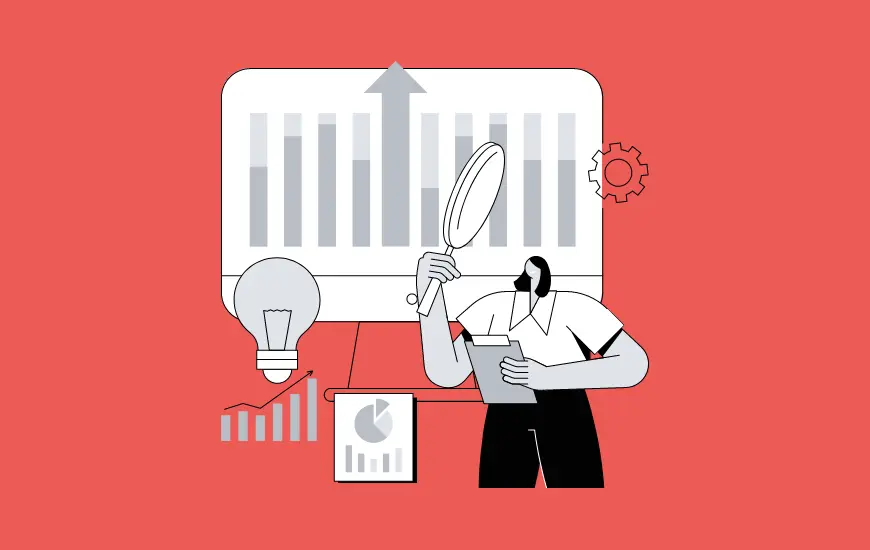