- Brief Overview of Machine Learning and How it Works in eCommerce
- 10 Benefits of Utilizing Machine Learning in eCommerce
- Dynamic Pricing
- Enhanced Search Functionality
- Customer Segmentation
- Churn Prediction
- Automated Marketing
- Inventory Management
- Contextual Shopping
- Improved Customer Service
- Fraud Detection
- Trend Analysis
- eCommerce Machine Learning Use Cases with Real-Life Examples
- Amazon’s Personalized Recommendation Engine
- eBay’s Localized Shopping Experience
- Alibaba’s Dynamic Pricing Strategy
- Walmart’s Inventory Management Optimization
- Anheuser-Busch’s Fleet Optimization
- Etsy’s Violation Detection System
- Best Practices for Implementing Machine Learning in eCommerce
- Set Clear Objectives
- Gather High Data Quality
- Select Appropriate ML Models Based on Business Needs
- Implement Customization Strategies
- Focus on Continuous Improvement for ML Models
- Future Innovations in Machine Learning for eCommerce
- Challenges of Implementing Machine Learning in eCommerce and Solutions to Overcome Those
- Integration with Existing Systems
- Scalability Issues
- Privacy and Compliance
- Model Accuracy and Bias
- Transform Your eCommerce Strategy with Appinventiv’s ML Expertise
- FAQs
eCommerce has come a long way from its humble beginnings of simple online storefronts and basic transaction processing. Today, it’s a dynamic realm where machine learning is dramatically reshaping the landscape. No longer just about browsing and buying, modern eCommerce harnesses the power of sophisticated algorithms to deliver hyper-personalized shopping experiences.
As machine learning delves into vast troves of consumer data, it’s enabling businesses to predict preferences, optimize inventory, and create highly targeted marketing strategies. The technology not only enhances operational efficiency but also transforms how brands connect with their customers, making every interaction feel uniquely tailored. As we advance further into this digital age, machine learning stands as a pivotal force, driving innovation and redefining the future of online retail.
According to a report by Statista, the global market for machine learning is anticipated to reach $503.40 billion by 2030, during the forecast period 2020- 2030. The market is primarily driven by the increasing utilization of AI and machine learning in eCommerce, finance, transportation, healthcare, manufacturing, and other industries.
Also, the demand for data-driven decision-making, advancements in computing power, and the proliferation of big data fuel market growth as businesses seek to enhance efficiency, personalization, and innovation through machine learning technologies.
This is evident in the fact that the use of ML for eCommerce has brought transformative changes in various businesses. For instance, Amazon is continuously enhancing its recommendation engine using machine learning to provide more personalized product suggestions.
Similarly, Shopify expanded the availability of its AI and ML-powered tools, including the Sidekick assistant and an image-generation feature, to more users recently. This move is part of Shopify’s strategy to enhance its eCommerce platform’s appeal and drive revenue growth.
There are numerous machine learning use cases in eCommerce that have revolutionized the industry. However, before we dive into those details later in the blog, let’s first take a closer look at how ML in eCommerce actually works.
Brief Overview of Machine Learning and How it Works in eCommerce
Machine learning is transforming eCommerce by creating a more responsive and adaptive shopping experience. It enables systems to understand and predict customer needs in real-time, seamlessly integrating personalized experiences into every interaction.
Through continuous learning from user data and behavior, machine learning models dynamically adjust to shifting trends, providing relevant and easy-to-understand recommendations that enhance the overall shopping journey.
In eCommerce, supervised learning leverages labeled data to improve customer experiences and optimize operations. For example, platforms analyze customer behavior and purchase history to generate personalized product recommendations. This approach also supports dynamic pricing strategies, where models predict optimal prices based on market trends and competition, driving increased sales and profitability.
Unsupervised learning involves analyzing unlabeled data to uncover hidden patterns, like identifying different customer segments. By grouping customers with similar behaviors, businesses can more effectively target marketing efforts and promotions. It also plays a crucial role in detecting anomalies, like fraudulent activities, by spotting unusual patterns in transaction data. Together, these techniques enhance personalization, efficiency, and security in eCommerce.
Reinforcement learning in eCommerce involves training models to make decisions by rewarding them for achieving desired outcomes, such as increasing sales or customer engagement. This approach is particularly useful for optimizing long-term strategies, like personalized marketing campaigns or dynamic pricing, where the model continuously learns from its actions to maximize overall success.
Leveraging eCommerce machine learning empowers strategic decision-making, helping businesses stay competitive and agile. This leads to a more intelligent and efficient eCommerce ecosystem that evolves alongside its customers.
10 Benefits of Utilizing Machine Learning in eCommerce
ML applications in eCommerce are transforming the industry, from enhancing personalization to optimizing business operations. Let’s have a look at some of the top benefits of ML in eCommerce.
Dynamic Pricing
Machine learning for eCommerce enables dynamic pricing by analyzing real-time data on demand, competitor pricing, and inventory levels. This approach helps companies stay competitive, optimize revenue, and adapt to market conditions through automated price adjustments.
For example, machine learning algorithms can automatically lower prices during periods of low demand or raise them when demand is high, helping businesses maximize revenue while keeping customer appeal intact.
Enhanced Search Functionality
Machine learning in eCommerce improves search functionality by comprehending the context and intent of user searches. It improves search algorithms based on user behavior, past searches, and product attributes to provide more accurate and relevant results.
Owing to this more efficient and intuitive search experience, users can find what they need more quickly and with less hassle, raising customer satisfaction and conversion rates.
Customer Segmentation
Using a range of factors, including engagement levels, demographics, and purchase patterns, ML-powered customer segmentation separates the client base into discrete groups. Businesses can develop customized offers and targeted marketing campaigns based on each group’s unique requirements and preferences by using this segmentation.
By providing customers with relevant deals and information, businesses can improve customer loyalty, increase engagement, and increase the efficacy of marketing initiatives.
Churn Prediction
Machine learning for eCommerce algorithms analyze interaction and behavior patterns to pinpoint customers at risk of churning. Businesses can target retention tactics to address possible concerns by recognizing early warning indications of churn, such as decreased engagement or changes in purchasing habits.
Proactive strategies like tailored offers and customer service interventions increase retention rates and lessen the negative effects of client attrition on income.
Automated Marketing
Through data analysis on customer responses, engagement, and campaign performance, machine learning optimizes marketing initiatives. Through the automation of marketing strategy adjustments based on this information, businesses can guarantee that their efforts are more focused and efficient.
Since major campaigns are customized to the interests and habits of particular client segments, engagement rates, and conversion success increase, improving the return on marketing investment.
Inventory Management
Predictive analytics driven by machine learning examine past sales data, seasonality, and market patterns to forecast demand trends and optimize inventory levels. This feature ensures that inventory aligns with present and projected demand, helping avoid overstock and stockouts.
Effective inventory control lowers carrying costs, cuts waste, and boosts the performance of the supply chain as a whole, improving product availability and saving money.
Contextual Shopping
By evaluating real-time data on user behavior, location, and preferences, machine learning improves contextual shopping by providing extremely relevant product recommendations. With this strategy, the purchasing experience is customized to each customer’s unique context, including past searches, current trends, or location-specific demands.
Machine learning assists in presenting tailored offers and products that are more likely to satisfy a customer’s urgent needs. This degree of customization guarantees a more user-friendly and fulfilling buying experience, increasing customer satisfaction and loyalty.
At Appinventiv, we developed Edamama, an eCommerce app crafted to provide mothers with a highly personalized shopping experience and superior customer service.
The platform excels by offering tailored product recommendations based on a child’s gender, age, and product ratings upon registration. Our efforts helped Edamama secure $5 million in funding, deliver over 20,000 SKUs, and serve more than 100,000 mothers.
Improved Customer Service
Chatbots and virtual assistants driven by machine learning offer quick, context-aware assistance to clients, managing a variety of questions and problems without the need for human participation.
These AI-powered solutions may respond to requests individually, quickly manage routine inquiries, and give round-the-clock assistance. By cutting response times and enhancing the consistency of client interactions, businesses can improve overall customer satisfaction and free up human agents to work on more difficult tasks.
Fraud Detection
Machine learning in eCommerce helps in monitoring user behavior and transaction trends to spot anomalies that might indicate fraud. Through real-time pattern recognition and historical data analysis, these algorithms can identify potentially fraudulent transactions before they are executed.
In the end, this proactive strategy contributes to a more secure shopping environment by preventing financial losses, safeguarding sensitive consumer information, and maintaining the integrity of the business’s operations.
Also Read: Financial Fraud Detection Using Machine Learning
Trend Analysis
Machine learning examines vast amounts of data to identify new trends and changes in customer preferences. Machine learning models can provide important information about how the market is changing by processing data from various sources, including market research, social media, and sales records. Businesses can utilize this information to modify their product offerings, marketing plans, and inventory control to keep ahead of trends and seize new opportunities.
eCommerce Machine Learning Use Cases with Real-Life Examples
There are a multitude of use cases and real-life examples that showcase how machine learning is revolutionizing eCommerce. Let’s explore how leading companies leverage machine learning to drive innovation and enhance customer experiences.
Amazon’s Personalized Recommendation Engine
Amazon’s recommendation engine, driven by Amazon Personalize, utilizes advanced machine learning techniques to provide highly individualized product recommendations. It analyzes users’ past interactions, such as their browsing history, search activities, and previous purchases, to gain insights into their preferences and behaviors. With the support of AWS’s scalable infrastructure, Amazon Personalize processes and interprets large volumes of data to deliver timely and relevant recommendations.
This system enhances user engagement by presenting tailored content that aligns with each user’s unique interests, while continuously adapting to their evolving preferences. The scalability of Amazon Personalize ensures that it can efficiently manage data for businesses of various sizes, boosting conversion rates and customer satisfaction by offering a more relevant and personalized shopping experience.
eBay’s Localized Shopping Experience
eBay is revolutionizing search experiences through advanced machine learning technology, enhancing both accuracy and relevance. Initially applied in Russia and now expanding to France, Italy, and Spain, eBay uses automatic machine translation to provide localized shopping experiences. By translating search queries and inventory from various languages, eBay ensures that users receive relevant results regardless of their location. This technology also extends to optimizing search functionality by leveraging machine learning for tasks like item categorization, price prediction, and attribute extraction.
The flagship application, Best Match, uses these algorithms to balance factors like item popularity, value, and return policies to present the most relevant deals. As machine learning technology evolves, eBay is leveraging open-source libraries and advanced computing to rapidly develop and refine search models, marking a significant leap in how search experiences are tailored and optimized.
Alibaba’s Dynamic Pricing Strategy
Alibaba Cloud integrates machine learning to enhance its range of services, improving cloud infrastructure, customer support, and data analytics capabilities. Alibaba utilizes machine learning to implement dynamic pricing strategies that adjust in real-time based on market demand and competitive analysis, enabling the company to maximize revenue and remain competitive.
This technology analyzes vast amounts of data, including competitor pricing and consumer behavior, to set optimal prices that attract customers while optimizing profit margins. By continuously adjusting prices, Alibaba ensures it offers competitive rates and captures value from changing market conditions. This adaptive approach helps Alibaba maintain its edge in a fast-paced eCommerce environment.
Walmart’s Inventory Management Optimization
Walmart utilizes machine learning for eCommerce strategies to streamline its inventory management and optimize supply chain operations. By analyzing sales data and market trends, Walmart can dynamically adjust its inventory levels, ensuring product availability while avoiding overstock situations. Machine learning-driven predictive analytics helps forecast demand and optimize stock levels, reducing the risks of stockouts and excess inventory. This improves supply chain management and cost efficiencies by maintaining balanced stock levels and minimizing waste.
To enhance its AI capabilities, Walmart has developed the Element platform, which simplifies the implementation of AI and machine learning at scale. This platform provides data scientists, engineers, and developers with the tools needed to build and deploy machine learning models efficiently. Element allows Walmart to gain valuable insights into competitor pricing, product assortment, and marketing strategies, facilitating better decision-making.
Walmart also utilizes virtual try-ons that help customers visualize and choose the right product for themselves.
Shoppers can virtually try on clothes by either uploading a photo of themselves or selecting a model with similar height, body shape, and skin tone in the app.
Anheuser-Busch’s Fleet Optimization
To enhance its eCommerce logistics and manage its expanding customer base, Anheuser-Busch, the world’s largest brewing company, adopts a machine learning-driven fleet optimization system for its last-mile delivery operations. This system utilizes historical data, such as traffic patterns, parking availability, and weather conditions, to identify the most efficient and cost-effective delivery routes. It then provides real-time navigation and dock location details to drivers and dispatchers through a dedicated app.
As a result of implementing this advanced system, Anheuser-Busch achieved an impressive 80% reduction in late deliveries. The improved route optimization not only cuts down on delays but also reduces operational costs, showcasing the powerful impact of machine learning on modernizing logistics and enhancing delivery performance.
Etsy’s Violation Detection System
Etsy harnesses the power of machine learning in eCommerce to keep its marketplace safe and vibrant, which serves over millions of active buyers and sellers. To ensure a positive experience, Etsy uses advanced technologies, including Convolutional Neural Networks (CNNs) and Large Language Models (LLMs).
CNNs like EfficientNet help sift through the images of listings to spot any that might breach policies, while LLMs such as BERT and its lighter counterpart, ALBERT, analyze the text to catch any violations. This combination helps Etsy thoroughly review both the visual and textual aspects of listings.
Etsy’s method involves constantly updating its data with carefully labeled examples of what does and doesn’t violate policies. This allows the machine learning models for eCommerce to learn and improve over time. They use techniques to handle tricky cases and regularly test their models to ensure they work well in real-life situations. This approach has helped Etsy identify and remove over 100,000 policy violations in the past year, reflecting their commitment to creating a safe and trustworthy space where creative businesses can thrive.
Also Read: An Explorative Guide to LLM Fine Tuning – Implications, Applications, Methods, Best Practices
Best Practices for Implementing Machine Learning in eCommerce
Implementing ML in eCommerce can significantly enhance various aspects of the business, from personalized customer experiences to optimized inventory management. Here are some best practices to follow when integrating ML into an eCommerce platform.
Set Clear Objectives
Start by identifying the specific business goals you aim to achieve by implementing ML in eCommerce, such as boosting conversion rates, enhancing customer service, or streamlining supply chain processes. Set clear, measurable KPIs to monitor and assess the effectiveness of your machine learning eCommerce projects.
For example, if your focus is on improving customer experience, relevant metrics might include higher customer satisfaction scores or lower bounce rates. Well-defined objectives and KPIs will steer your ML efforts and ensure they align with overall business goals.
Gather High Data Quality
Working with high-quality data is essential to achieving successful machine learning eCommerce outcomes. Gather and manage data from various touchpoints, including customer interactions, purchase records, and site activity.
Ensure that this data is thoroughly cleaned and preprocessed to eliminate inconsistencies, duplicates, or errors that could undermine model performance. Additionally, stringent data privacy measures should be implemented to adhere to regulations, protect customer information, and uphold trust.
Select Appropriate ML Models Based on Business Needs
Choosing the right machine learning models for eCommerce depends on what you need them to do. For instance, collaborative filtering algorithms are a great choice if you want to build a recommendation system. For identifying fraud, anomaly detection models can be very effective.
Evaluate your models using performance metrics like accuracy and precision to ensure they fit your business needs. Cross-validation is also important to confirm that your models perform well with new data and don’t just work well with the data you trained them on.
Implement Customization Strategies
Customization is a powerful way to use machine learning in eCommerce. Using algorithms like content-based filtering, you can set up recommendation systems that suggest products based on what users have looked at or purchased before.
Additionally, dynamic pricing strategies powered by ML should be considered, which adjust prices in real-time based on demand, competitor prices, and inventory levels. This approach helps create a customized shopping experience and can boost revenue.
Focus on Continuous Improvement for ML Models
Machine learning models need constant updates and refinements to stay effective and up-to-date. Regularly retrain your models with fresh data to stay in tune with shifting customer behaviors and market trends.
Set up feedback loops to collect input from users and stakeholders, which can help guide necessary adjustments and improvements. This ongoing process ensures that your ML systems continue delivering accurate results and remain valuable as your business and the eCommerce environment change.
Future Innovations in Machine Learning for eCommerce
The future of ML in eCommerce looks incredibly promising, with advancements set to reshape the industry. We’ll see more advanced recommendation systems that use deep learning to offer highly personalized product suggestions based on real-time behavior and preferences.
Hyper-personalized recommendations will evolve to offer even more precise product suggestions by gaining a deeper insight into individual customer preferences and behaviors. Additionally, visual search capabilities will allow customers to locate products using images, making the search process more intuitive and user-friendly.
The impact of machine learning in eCommerce is profound, shaping the future of online shopping with enhanced personalization and efficiency. Chatbots will become more intuitive, providing more natural and effective customer support. As these technologies develop, eCommerce will benefit from even more tailored shopping experiences, streamlined operations, and deeper insights into consumer behavior, driving significant growth and innovation.
Challenges of Implementing Machine Learning in eCommerce and Solutions to Overcome Those
Businesses often encounter a few challenges while merging machine learning and eCommerce into their existing processes. Let’s have a look at some of those challenges with solutions.
Integration with Existing Systems
Integrating machine learning models with existing eCommerce platforms can be tricky and potentially disruptive.
Collaborate with skilled developers or consultants to achieve smooth integration. Utilize APIs and modular approaches to connect ML models with your current systems seamlessly.
Scalability Issues
As your eCommerce business grows, scaling ML models to handle increased data and traffic can be challenging.
Opt for scalable machine learning solutions and cloud-based services that expand with your needs. Regularly review and adjust your models to manage larger datasets and more complex tasks efficiently.
Privacy and Compliance
Managing personal data while complying with privacy laws is a significant concern.
Implement strong data protection measures and ensure compliance with applicable regulations. Use techniques like anonymization and secure storage to protect customer data and uphold privacy standards.
Model Accuracy and Bias
Ensuring that ML models are accurate and free from bias is crucial, as biased models can harm customer experiences and lead to unfair practices.
Continuously monitor and assess model performance. Use diverse and representative datasets for training, and apply methods to detect and reduce bias in your models.
Transform Your eCommerce Strategy with Appinventiv’s ML Expertise
Integrating machine learning into your eCommerce business can make a huge difference, and Appinventiv is here to help you do it right. Our experts, with next-gen machine learning development services, can create customized ML solutions tailored to your needs, whether that’s for enhancing product recommendations, optimizing pricing, or improving demand forecasting.
Our experience and expertise ensure that your ML models will be accurate, effective, and smoothly integrated into your current systems. As a top provider of eCommerce software development services, we have collaborated with leading brands such as Adidas, IKEA, Edamama, and 6 Street, enhancing their platforms with innovative features and driving user engagement.
With our support, you can confidently navigate the future of AI in eCommerce, knowing you have a reliable partner to guide you through every stage of your machine learning journey.
Partner with us to transform your vision into reality and propel your business forward today!
FAQs
Q. What is machine learning in eCommerce?
A. ML in eCommerce involves using algorithms to analyze data and make smart decisions automatically. It allows systems to learn from past interactions and improve their performance over time. This technology helps deliver more personalized shopping experiences, such as tailored product recommendations and dynamic pricing, making online shopping more efficient and enjoyable.
Q. How is machine learning used in the eCommerce industry?
A. Machine learning in eCommerce enhances customer experiences and operational efficiency. It powers recommendation engines that suggest products based on what users have browsed or bought before, adjusts prices dynamically based on market conditions, and forecasts demand to keep inventory levels optimal. ML in eCommerce also enables real-time customer support through chatbots and analyzes customer feedback to identify trends and improve services.
A. What are some of the top examples of ML in eCommerce?
Q. Here are some of the top examples of ML in eCommerce:
- Fraud detection
- Chatbots and virtual assistants
- Visual search
- Personalized recommendations
- Dynamic pricing
- Customer segmentation
- Inventory management
- Sentiment analysis
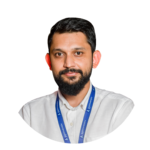
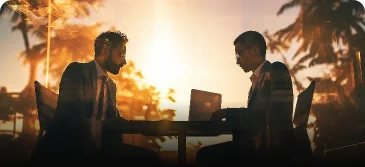
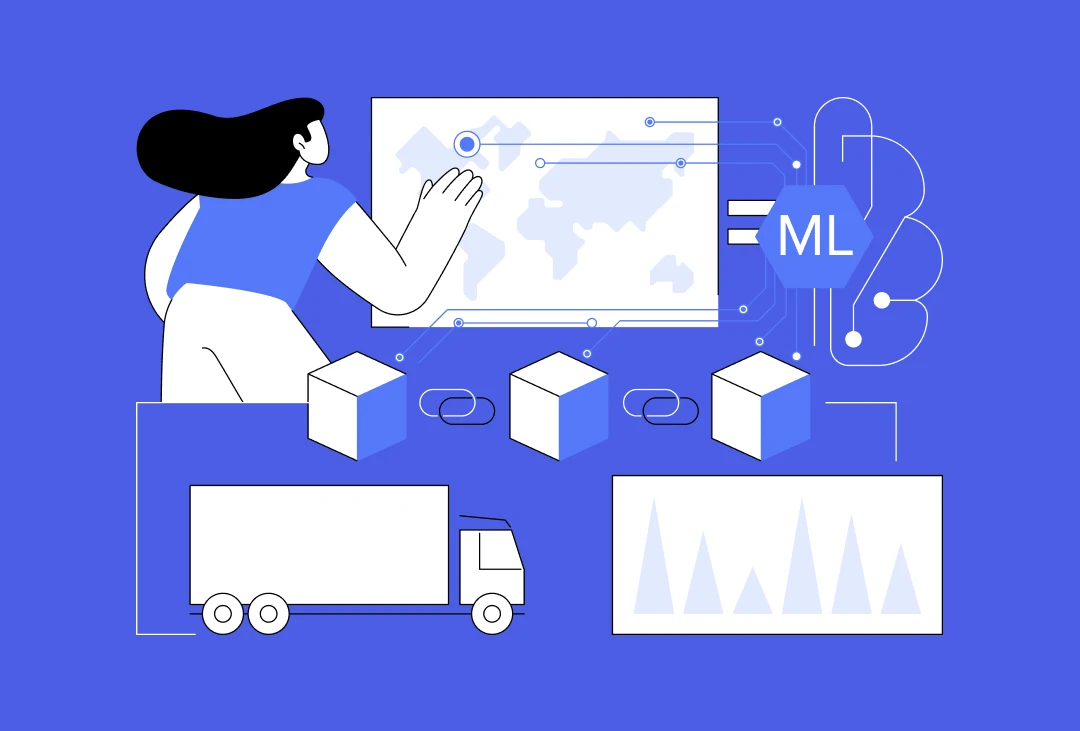
10 Use Cases and Examples of How Machine Learning is Transforming the Logistics Industry
The widespread adoption of ML has resulted in the explosion of data from sensors, customer interactions, and digital platforms across different domains. More and more businesses are leveraging machine learning in logistics to improve their strategic decision-making expertise centered around tailoring customer experience, improving productivity, increasing engagement, and preventing fraud. According to the McKinsey Global…
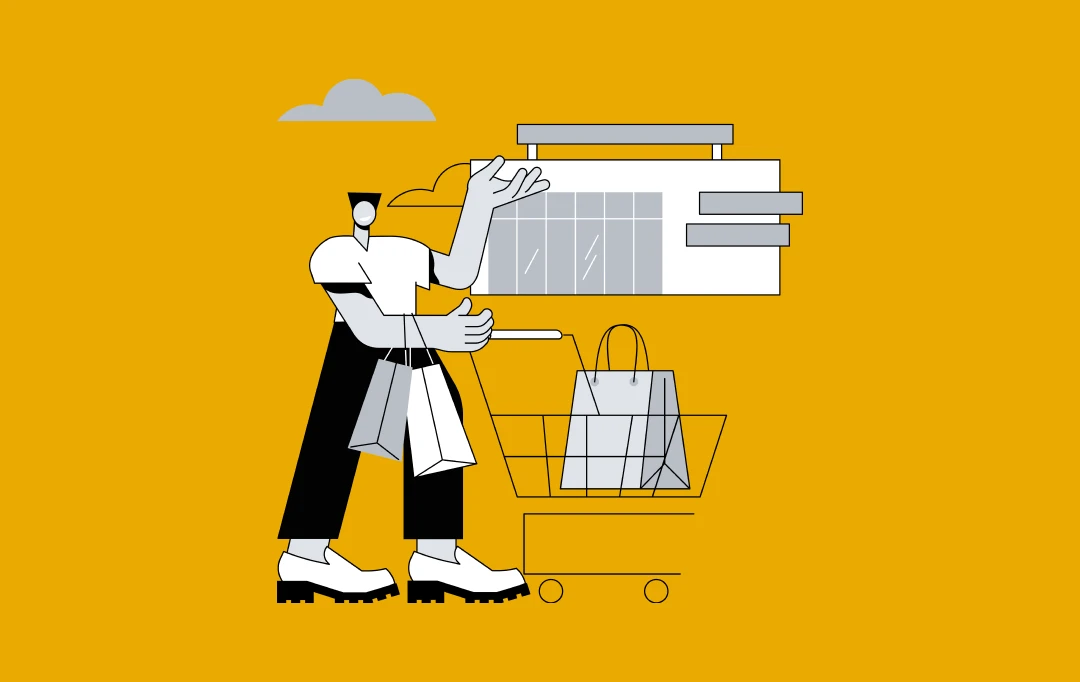
How Machine Learning in Retail is Redefining the Sector - Key Business Opportunities and Challenge
In the last few years, the retail industry has faced some critical challenges like the global pandemic — COVID-19, supply chain disruptions, curfews, energy crunches, sustainability pressures, cybersecurity threats, eCommerce apocalypse, and so on. Despite these obstacles, the industry has shown remarkable resilience, transforming its operations to meet the demands of a rapidly changing retail…
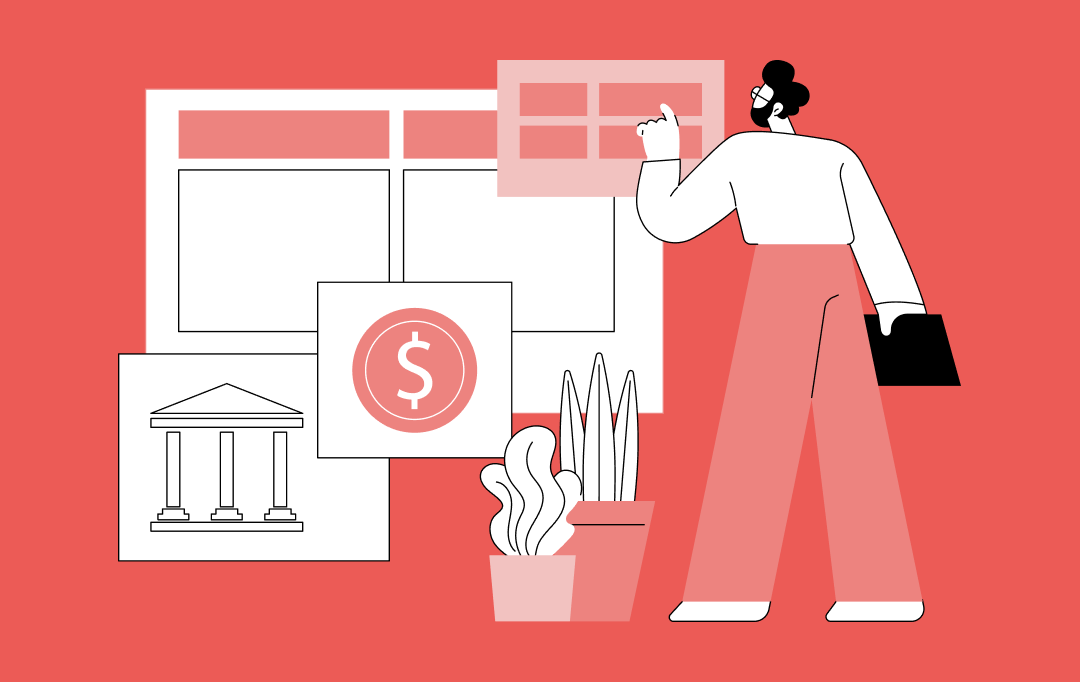
Machine Learning in Banking - Use Cases and Implementation Process
The speed at which fintech transformation is happening is nothing short of a never-seen-before revolution. With the advent of technologies like blockchain, artificial intelligence, metaverse, and edge computing, the fintech space - as we know it - has drastically changed. This transformation's intrinsic change can be seen in how comfortable users have gotten with on-the-move…