- Generative AI in Finance: An In-Depth Exploration
- Top Generative AI Use Cases in Finance Services
- Consultancy for Regulatory Code Changes
- Tailored Financial Advice
- Streamlined Search and Synthesis of Financial Documents
- Automated Accounting Processes
- Efficient Financial Report Generation
- Detection of Fraud and Ensuring Data Privacy
- Portfolio and Risk Management Solutions
- Implementation of Algorithmic Trading Strategies
- Optimization of Tax Processes
- Customer Sentiment Analysis
- Credit Risk Assessment
- Development of Chatbots and Virtual Assistants for Finance
- Real-World Examples of Generative AI in Financial Services
- Multiple Generative AI Models Finding Operations in the Finance Sector
- Variational Autoencoders (VAEs)
- Generative Adversarial Networks (GANs)
- Autoregressive Models
- Transformer Models
- Deep Reinforcement Learning (DRL) Models
- PixelCNN
- Flow-based Models
- Variational Autoencoder with Normalizing Flows (VANF)
- How to Incorporate Generative AI into Your Financial Operations - Key Steps
- Needs Assessment and Objectives
- Data Collection and Preparation
- Model Development and Implementation
- Testing and Validation
- Integration into Software Workflow
- Continuous Monitoring and Optimization
- Meeting the Demands: Solutions for Challenges of Generative AI in Financial Services
- Revolutionize Your Finance Business with Appinventiv's Cutting-Edge Generative AI Development Services
- FAQs
Have you ever considered the astonishing precision and growth of the finance industry? It’s a realm where errors are minimal, accuracy is paramount, and progress is perpetual. But what’s really making it all happen behind the scenes? Well, it’s Generative AI.
Generative AI in finance has become a valuable tool of innovation in the sector, offering advantages that redefine how financial operations are conducted and services are delivered.
Its integration into financial institutions profoundly improves efficiency, decision-making, and customer engagement. By automating repetitive tasks and optimizing workflows, Generative AI streamlines operations, reduces errors, and cuts costs, ultimately enhancing businesses’ bottom lines.
The table above illustrates that Generative AI in the financial services sector is expected to experience a CAGR of 28.1% from 2022 to 2032. With this growth trajectory, the market size of generative AI in finance is anticipated to surpass $9.48 billion by 2032.
The increasing market size clearly indicates the significant opportunity available to finance businesses for investment in Generative AI, enabling them to capitalize on its transformative capabilities and unlock new avenues of growth and innovation.
This blog will delve into exploring various aspects of Generative AI in the finance sector, including its use cases, real-world examples, and more. So, without further ado, let’s jump right into the details.
Generative AI in Finance: An In-Depth Exploration
In the finance sector, Generative AI has become a tool that financial institutions cannot afford to overlook. It transforms operations and decision-making processes with unmatched capabilities.
The innovative technology holds the potential to elevate businesses significantly. According to a Deloitte report, advancements in generative AI for finance could boost business productivity growth by 1.5 percentage points. Thus, finance businesses can see substantial gains in productivity and revenue by integrating generative AI into their processes.
The report also dwells on how Generative AI for financial services can enhance enterprise and finance workflows by introducing contextual awareness and human-like decision-making capabilities, potentially revolutionizing traditional work processes. These advancements are made possible by foundation models, which utilize deep learning algorithms inspired by the organization of neurons in the human brain.
Furthermore, according to a report by BCG, finance functions within global companies are embracing the transformative potential of AI tools like ChatGPT and Google Bard. These tools are expected to reshape the future of work within the finance function, revolutionizing processes, enhancing efficiency, and driving innovation, requiring CFOs to gain a nuanced understanding of their impact.
The integration of Generative AI into finance operations is expected to follow an S-curve trajectory, indicating significant growth potential.
Currently, finance teams are actively exploring the capabilities of Generative AI to streamline processes, particularly in areas such as text generation and research.
Looking ahead, Generative AI is poised to revolutionize core operations and reshape business partnering within the finance sector. Furthermore, it is anticipated to collaborate with traditional AI forecasting tools to enhance the capacity and efficiency of finance functions.
Also Read: The Transformative Impact of AI in Finance
Top Generative AI Use Cases in Finance Services
The convergence of Generative AI and finance represents a cutting-edge fusion, transforming conventional financial practices through sophisticated algorithms. The use of Generative AI in finance encompasses a wide range of applications, including risk assessment, algorithmic trading, fraud detection, customer service automation, portfolio optimization, and financial forecasting.
Let’s delve into the multitude generative AI use cases in banking is being leveraged and elevating businesses.
Consultancy for Regulatory Code Changes
Generative artificial intelligence in finance can analyze vast amounts of regulatory data and provide insights to organizations on how to adapt to regulatory code changes efficiently. Interpreting complex regulatory requirements helps businesses stay compliant and mitigate regulatory risks effectively.
Tailored Financial Advice
Generative AI and finance converge to offer tailored financial advice, leveraging advanced algorithms and data analytics to provide personalized recommendations and insights to individuals and businesses. This tailored approach of generative AI finance enhances customer satisfaction and helps individuals make informed decisions about investments, savings, and financial planning.
Streamlined Search and Synthesis of Financial Documents
Generative artificial intelligence in finance simplifies the process of searching and synthesizing financial documents by automatically extracting relevant information from diverse sources. This capability saves time for financial analysts and improves decision-making by providing comprehensive insights.
Automated Accounting Processes
Generative AI in accounting is highly advantageous in automating routine accounting tasks such as data entry, reconciliation, and categorization of financial transactions. Reducing manual effort and minimizing errors increases efficiency and accuracy in financial record-keeping.
Efficient Financial Report Generation
Generative AI has potential to streamline the process of generating financial reports by synthesizing data from multiple sources and presenting it in a structured format. This enables businesses to produce timely and accurate reports for stakeholders, regulatory authorities, and investors.
Detection of Fraud and Ensuring Data Privacy
One of the effective applications of generative AI in finance is fraud detection and data security. Generative AI algorithms can detect anomalies and patterns indicative of fraudulent activities in financial transactions. Additionally, it ensures data privacy by implementing robust encryption techniques and monitoring access to sensitive financial information.
( Also read: AI in Banking – How Artificial Intelligence is Used in Banks)
Portfolio and Risk Management Solutions
Generative artificial intelligence in finance enables sophisticated portfolio optimization and risk management by analyzing historical data, market trends, and risk factors. It helps financial institutions make data-driven decisions to maximize returns while minimizing risk exposure.
Implementation of Algorithmic Trading Strategies
Generative AI algorithms develop and implement algorithmic trading strategies by analyzing market data and identifying profitable trading opportunities. This enhances trading efficiency and enables traders to capitalize on market fluctuations in real-time.
Optimization of Tax Processes
Generative AI automates tax compliance processes by analyzing tax laws, regulations, and financial data to optimize tax planning and reporting. It helps businesses minimize tax liabilities while ensuring compliance with tax regulations.
Customer Sentiment Analysis
Generative AI can analyze customer feedback from various sources, such as social media, surveys, and customer support interactions, to gauge sentiment toward financial products and services. Financial institutions can tailor their offerings and marketing strategies to better meet customer needs and preferences by understanding customer sentiment.
Credit Risk Assessment
One prominent use of generative AI in finance is credit risk assessment. Generative AI algorithms can analyze diverse data sources, including credit history, financial statements, and economic indicators, to assess credit risk for individual borrowers or businesses. This enables lenders to make more accurate and informed decisions regarding loan approvals, interest rates, and credit limits, ultimately minimizing default risks and optimizing loan portfolios.
Development of Chatbots and Virtual Assistants for Finance
Generative AI powers chatbots and virtual assistants that provide personalized customer support, answer queries, and perform routine financial tasks. These AI-driven assistants enhance customer experience and streamline interactions between financial institutions and clients.
Real-World Examples of Generative AI in Financial Services
Let’s delve into how top industry players are harnessing the power of Generative AI in banking and finance to revolutionize their approach, enhance customer experiences, and drive profitability.
Through a detailed exploration, we’ll uncover the optimistic impact of Generative artificial intelligence in finance.
JP Morgan
JPMorgan Chase, a leading global financial institution, has demonstrated a strong commitment to innovation through its proactive investment in cutting-edge AI technologies. Among these advancements, Generative AI stands out as a pivotal tool leveraged by the brand to elevate various facets of its operations.
From refining risk management frameworks to enhancing trading strategies and elevating customer service experiences, Generative AI plays a multifaceted role within JPMorgan’s ecosystem.
Goldman Sachs
Goldman Sachs, renowned for its prowess in investment banking and asset management, has embraced the transformative potential of AI and machine learning technologies, including Generative AI.
By incorporating Generative AI into its trading operations, Goldman Sachs endeavors to optimize investment strategies, improve risk management protocols, and stay ahead of market trends
Morgan Stanley
Morgan Stanley, a stalwart in wealth management and financial services, is at the forefront of exploring AI-driven innovations to enhance its competitive edge. With a keen focus on leveraging Generative AI, Morgan Stanley aims to bolster its fraud detection capabilities, optimize portfolio management processes, and provide personalized financial advice to its clients.
Multiple Generative AI Models Finding Operations in the Finance Sector
The FinTech industry thrives on innovation, constantly seeking new ways to enhance its approach and drive profitability. Generative AI models play a pivotal role in this quest for advancement, offering a range of valuable tools and techniques that finance businesses leverage to achieve their goals.
Let’s delve into each of these models and explore how they contribute to the success of the FinTech sector.
Variational Autoencoders (VAEs)
VAEs are neural network architectures that learn to encode and decode high-dimensional data, such as images or text. They are widely used for generating realistic and diverse outputs.
Generative Adversarial Networks (GANs)
GANs consist of two neural networks, a generator and a discriminator, that are trained together competitively. GANs excel at generating realistic images, videos, and other forms of data.
Autoregressive Models
Autoregressive models, such as autoregressive moving average (ARMA) and autoregressive integrated moving average (ARIMA), predict future values in a time series based on past observations. They are commonly used for time series forecasting tasks.
Transformer Models
Transformer models, like OpenAI’s GPT (Generative Pre-trained Transformer) series, are based on a self-attention mechanism that allows them to process data sequences more effectively. These models are versatile and can generate text, images, and other types of data.
Deep Reinforcement Learning (DRL) Models
DRL models combine deep learning with reinforcement learning techniques to learn complex behaviors and generate sequences of actions. They are often used in robotics and other dynamic environments.
PixelCNN
PixelCNN is a type of autoregressive model designed specifically for generating high-resolution images pixel by pixel. It captures the spatial dependencies between adjacent pixels to create realistic images.
Flow-based Models
Flow-based models are generative models that transform a simple probability distribution into a more complex one through a series of invertible transformations. These models are used for image generation, density estimation, and data compression tasks.
Variational Autoencoder with Normalizing Flows (VANF)
VANF combines the strengths of variational autoencoders (VAEs) and normalizing flows to generate high-quality, diverse samples from complex data distributions. It leverages normalizing flows to model complex latent space distributions and achieve better sample quality.
How to Incorporate Generative AI into Your Financial Operations – Key Steps
Let’s delve into grasping the holistic and strategic approach required for integrating Generative AI in financial services. Through a comprehensive understanding of systemic methodologies and partnering with a reliable development firm, businesses can effectively leverage Generative AI’s transformative potential to drive innovation and achieve their goals.
Needs Assessment and Objectives
Begin by initiating a comprehensive research phase to delve deep into the intricacies of finance projects. This involves conducting a meticulous needs assessment to precisely identify and define the challenges and objectives at hand.
Data Collection and Preparation
In the data collection phase, gather financial data comprehensively from various sources. Next, meticulously cleanse and preprocess the data to remove errors and standardize formats. Augment the dataset with additional relevant features to enhance its richness and diversity. Ensure regulatory compliance throughout these processes to uphold data integrity.
Model Development and Implementation
With a solid dataset in hand, it’s time to embark on the development and implementation of Generative AI models tailored specifically to finance projects. This stage involves deploying the right algorithms and methodologies to address the identified challenges and meet the defined objectives.
Testing and Validation
After completing model development, establish rigorous testing and validation protocols. This involves subjecting Generative AI models to exhaustive testing across diverse finance use cases and scenarios. Identify and address any potential shortcomings or discrepancies to ensure model robustness before deployment.
Integration into Software Workflow
Collaborate closely with software engineers to seamlessly integrate models into existing software workflows, ensuring UI/UX interaction and enhanced operational efficiency in the finance domain.
Continuous Monitoring and Optimization
Embrace continuous monitoring and improvement post-deployment to adapt to evolving finance trends. Implement real-time performance tracking, data analysis, and iterative enhancements to maintain the models’ effectiveness and relevance.
Meeting the Demands: Solutions for Challenges of Generative AI in Financial Services
Let’s embark on a comprehensive exploration of the formidable challenges encountered by finance businesses as they venture into the realm of Generative AI. We’ll delve deep into these challenges, unveiling innovative solutions poised to overcome these obstacles and pave the way for transformative advancements in the finance industry.
Data Quality and Bias
Generative AI models are susceptible to biases in the data they are trained on. This can lead to unfair outcomes in areas like loan approvals, credit scoring, or algorithmic trading. Biased data can perpetuate historical inequalities and lead to discriminatory practices.
Solutions
- Implement robust data cleaning techniques to identify and remove biases from training data sets.
- Incorporate diverse data sources to represent a broader spectrum of financial information.
- Develop fairness metrics and monitoring tools to track potential bias in model outputs.
- Employ human oversight and review processes for critical financial decisions generated by AI.
- Prioritize responsible AI principles and strategy in order to avoid data bias and ensure fair and equitable outcomes.
Explainability and Interpretability
Generative AI models can be complex, making understanding how they arrive at specific outputs difficult. This lack of transparency can be problematic for financial institutions that need to justify recommendations or decisions made by AI.
Solutions
- Utilize explainable AI (XAI) techniques to provide insights into the model’s reasoning process. This can help build trust and ensure regulatory compliance.
- Develop human-readable interpretations of the model’s outputs to facilitate understanding for financial professionals.
- Prioritize the development of interpretable Generative AI models specifically designed for financial applications.
Integration and Change Management
Integrating Generative AI models with existing financial systems can be complex. Additionally, financial institutions need to prepare their workforce for AI integration, addressing potential job displacement concerns and reskilling needs.
Solutions
- Develop a phased approach to AI adoption, starting with pilot projects to test integration feasibility.
- Invest in employee training programs to equip staff with the skills needed to work effectively with Generative AI.
- Foster a culture of innovation and collaboration to ensure smooth integration of AI into financial workflows.
- Openly communicate the potential benefits of AI to address workforce concerns and encourage positive change.
Model Generalizability and Adaptability
Financial markets are constantly evolving, and historical data might not always be a perfect predictor of future trends. Generative AI models trained on static data sets might struggle to adapt to these changes, leading to inaccurate or outdated outputs.
Solution
- Continuously update training data with new information to ensure model generalizability.
- Develop adaptive Generative AI models that can learn and adjust their outputs based on real-time data streams.
- Employ transfer learning techniques to leverage existing models’ knowledge in new financial scenarios.
(Also read: Preventing AI Model Collapse: Addressing the Inherent Risk of Synthetic Datasets)
Limited Data Accessibility
Generative AI models thrive on large, high-quality data sets. Financial data can be expensive to acquire, fragmented across different institutions, and subject to strict privacy regulations. This limited data access can hinder the development and effectiveness of Generative AI models in finance.
Solution
- Explore alternative data sources like social media sentiment or economic indicators to supplement traditional financial data.
- Develop data collaboration agreements between financial institutions to share anonymized data for model training while adhering to privacy regulations.
- Leverage synthetic data generation techniques to create artificial but realistic financial data sets for training purposes, ensuring compliance with data privacy rules.
Global Regulatory Fragmentation
The regulatory landscape for AI, particularly concerning Generative AI use in finance, still evolves and varies across different countries. This lack of consistent global regulations creates uncertainty for international financial institutions and discourages widespread technology adoption.
Solution
- Advocate for clear and consistent global regulations for Generative AI in finance through industry collaboration and engagement with regulatory bodies.
- Develop compliance frameworks that ensure Generative AI models adhere to existing financial regulations and emerging guidelines.
- Stay informed about evolving market regulations and adapt AI practices accordingly to maintain compliance.
Revolutionize Your Finance Business with Appinventiv’s Cutting-Edge Generative AI Development Services
As a dedicated financial application development company, Appinventiv offers a comprehensive approach to incorporating Generative AI into finance businesses, characterized by meticulous project planning, in-depth research analysis, and cutting-edge technology solutions. Our team of thought leaders combines exceptional service with expertise in the field, providing a tailored experience for both veteran and new clients.
With our extensive experience in developing AI-driven solutions, we design and implement custom Generative AI solutions tailored to the unique needs of each finance project. Our approach allows businesses to leverage generative AI in business applications, streamlining complex processes and generating innovative content automatically. This technology not only boosts productivity but also enhances decision-making, providing a competitive edge in today’s fast-paced market environment.
As a Generative AI development company, we prioritize thought leadership, continuously seeking ways to push the boundaries of what’s possible with leveraging Generative AI in finance.
Reach out to us to create innovative finance apps empowered with Generative AI solutions, enriching engagement and elevating user experiences in the financial sector.
FAQs
Q. How can Generative AI be used in finance?
A. Generative AI offers numerous applications in finance, ranging from customer engagement to risk management. It can be utilized to analyze customer sentiment, generate personalized financial advice, and automate investment strategies.
Additionally, Generative AI assists in generating synthetic financial data for training predictive models, optimizing portfolio management, and streamlining financial document processing.
Q. How can Generative AI assist in generating synthetic financial data for training predictive models?
A. Generative AI in finance plays a crucial role in generating synthetic data for training predictive models by mimicking the patterns and characteristics of real-world financial data. Through techniques such as Generative Adversarial Networks (GANs) and Variational Autoencoders (VAEs), Generative AI can create synthetic datasets that closely resemble actual financial data while preserving privacy and confidentiality.
Q. What are the primary challenges that businesses face when integrating Generative AI into their finance projects?
A. Businesses encounter several challenges when adapting Generative AI in finance projects, including data privacy concerns, model interpretability, integration with legacy systems, compliance with regulations, talent acquisition, and cost analysis. Addressing these challenges with the help of an expert technology partner like Appinventiv is essential for successful implementation and innovation in finance
Implementing robust data encryption techniques for enhanced privacy, developing explainable AI models for better interpretability, and offering comprehensive training programs to bridge talent gaps are potential solutions to these challenges.
Q. How does generative AI in finance work?
A. Generative AI in finance leverages sophisticated algorithms to process large datasets, uncover patterns, and produce new data or insights. It aids in developing predictive models, automating financial reports, identifying anomalies, and refining trading strategies. By simulating different scenarios, generative AI improves decision-making, enhances risk management, and bolsters fraud detection, providing financial institutions with a robust tool for innovation and efficiency.
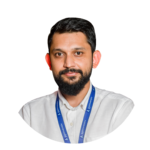
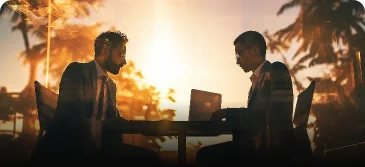
- In just 2 mins you will get a response
- Your idea is 100% protected by our Non Disclosure Agreement.
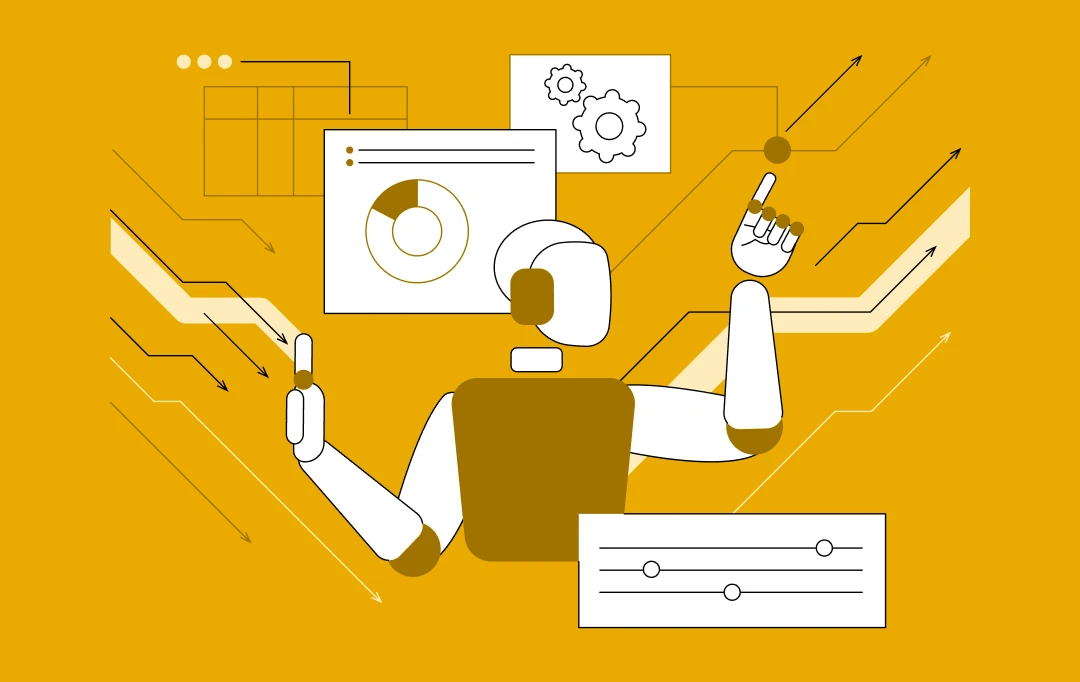
How to Integrate AI with EHR/EMR Systems for Streamlined Healthcare Operations
Key takeaways: Not adopting AI in EHR/EMR today risks falling behind. AI streamlines EHR workflows, cutting clinical and admin delays. Real-world hospitals are already seeing faster, smarter care with AI. Seamless AI integration needs system checks, data prep, and training. AI in EHR tackles real challenges like bias, cost, and compliance. Custom AI integration costs…
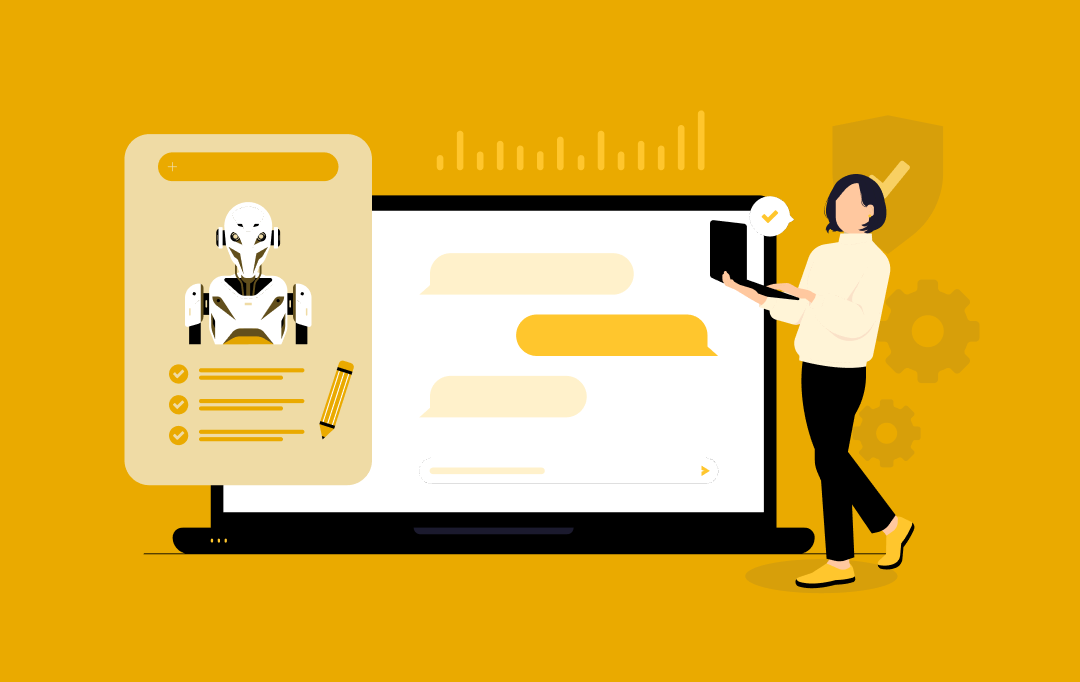
AI Agent-Driven UIs: Replacing App Menus and Buttons
Key takeaways: Challenge: Static menus and buttons also become outdated because people are frustrated by limited, multi-stage navigation. Solution: The AI Agent interacts with the user through a friendly UI that does not require cludgy menus but conversational and context-sensitive navigation. The Way AI Agents Work: AI agents establish a basis of natural language understanding,…
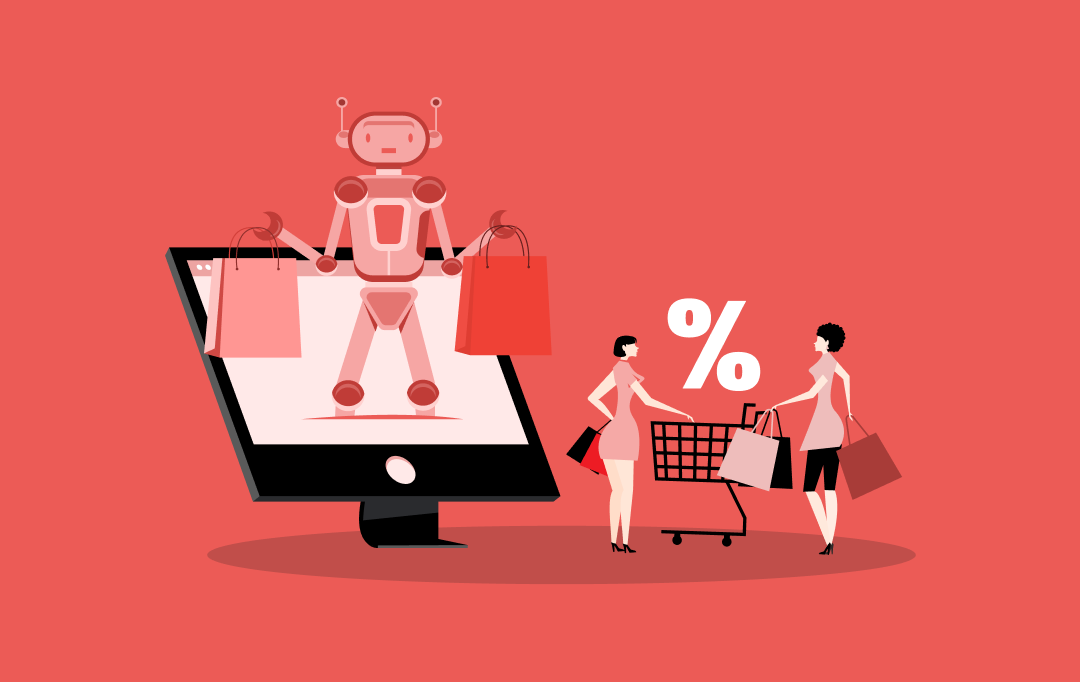
10 Use Cases and Benefits of How AI Agents Are Revolutionizing the Retail Industry
Key takeaways: AI agents in the retail industry are revolutionizing businesses by enhancing customer experience and streamlining operations. From personalized recommendations to predictive analytics, AI offers tangible benefits like increased conversions and reduced costs. AI adoption can lead to scalable growth and a competitive edge in the retail industry. Retailers must consider data strategies, talent…