- How is Artificial Intelligence Utilized in the Pharmaceutical Industry?
- Benefits of AI in the Pharmaceutical Industry: Enhancing Efficiency and Innovation
- Accelerated Drug Discovery
- Personalized Medicine & Care
- Enhanced Clinical Trials
- Optimized Drug Manufacturing
- Advanced Biomarker Discovery
- Improved Patient Monitoring and Adherence
- Top 10 Use Cases of AI in the Pharmaceutical Industry
- Predictive Toxicology
- Drug Repurposing
- Supply Chain Optimization
- Real-World Evidence Generation
- AI-Assisted Formulation Development
- Virtual Screening
- Patient Recruitment
- Regulatory Compliance
- Automated Literature Review
- Pharmacovigilance
- Real-Life World Examples of AI in the Pharmaceutical Industry
- Challenges While Leveraging AI in Pharmaceutical
- Generative AI in Pharma: The Future of Intelligent Drug Discovery and Beyond
- How Appinventiv Can Help You In Developing AI-based Platforms for Your Pharmaceutical Business
- FAQs
- Q. Why is AI important in the pharmaceutical industry?
- Q. What is the future of AI pharma in drug discovery?
- Q. What are the ethical considerations when using AI for patient data analysis in pharma?
- Q. How can AI assist in improving the accuracy and efficiency of pharmaceutical marketing strategies?
- Q. What are the top AI adoption trends in pharma for 2025?
- Q. How much cost and time can AI save pharmaceutical companies?
The pharmaceutical sector is evolving rapidly, with artificial intelligence playing a crucial role in transforming its capabilities. According to a PwC report, pharmaceutical companies could potentially increase their global operating profits by an additional $254 billion by 2030, driven by AI-based use cases.
This projected AI-driven value comprises $155 billion in the US, $52 billion in emerging markets, $33 billion in Europe, and $14 billion in other regions. This significant growth underscores the vast opportunities in leveraging AI for advancing the pharma industry.
AI in the pharmaceutical industry is revolutionizing drug discovery by sifting through extensive data rapidly, optimizing clinical trials, and enabling tailored patient care. The technology accelerates research processes, boosts accuracy, and enhances overall efficiency, leading to the development of quicker and more effective treatments.
In this article, we’ll dive deep into the exciting world of AI in pharmaceuticals, examining its benefits, diverse applications across the industry, and real-world examples that highlight its transformative impact.
How is Artificial Intelligence Utilized in the Pharmaceutical Industry?
Artificial intelligence (AI) is transforming the pharmaceutical industry by enhancing various stages of drug discovery, development, and production. Machine learning algorithms help researchers analyze vast datasets, identifying patterns and potential drug candidates faster than traditional methods.
AI can also predict how different compounds will behave in the body, reducing the time and cost involved in preclinical testing. By automating repetitive tasks and improving the accuracy of predictions, AI accelerates the process of bringing new drugs to market.
AI-powered tools also enhance personalized medicine by enabling the development of treatments tailored to individual patients based on genetic data, improving therapeutic outcomes and reducing adverse effects. This technology is reshaping the industry, making drug development more efficient and accessible.
Benefits of AI in the Pharmaceutical Industry: Enhancing Efficiency and Innovation
Integrating artificial intelligence in the pharmaceutical industry can provide companies with a significant edge by speeding up drug development, refining clinical trials, and advancing personalized treatments. This adoption not only drives innovation but also positions companies to attract leading professionals and excel in a fast-paced market.
Let’s dive into the impact of AI in the pharmaceutical industry and the extensive benefits it offers.
Accelerated Drug Discovery
AI-driven models can analyze vast amounts of chemical and biological data to identify potential drug candidates at a speed and accuracy unmatched by traditional methods. By predicting how different molecules will interact with biological targets, AI reduces the need for extensive and costly laboratory experiments. This not only shortens the drug discovery phase but also increases the chances of finding viable drug candidates, thereby significantly reducing the overall time and cost required to bring new treatments to market.
Personalized Medicine & Care
Pharma AI allows for the development of highly personalized treatment plans by integrating and analyzing diverse data sources, including genetic information, patient history, and lifestyle factors. Through advanced algorithms, AI can predict how individual patients will respond to different therapies, enabling healthcare providers to tailor treatments that are more effective and have fewer adverse effects.
This personalized approach improves patient outcomes by ensuring that treatments are specifically designed to meet the unique needs of each patient, rather than relying on a one-size-fits-all method.
Enhanced Clinical Trials
AI pharma can revolutionize the design and execution of clinical trials by improving patient selection, predicting better outcomes, and optimizing trial protocols. By analyzing historical trial data and real-time patient information, AI can identify the most suitable candidates for trials, reducing the time and cost associated with recruitment.
Furthermore, Pharma AI can monitor patient adherence and outcomes in real-time, enabling faster identification of potential issues and allowing for dynamic adjustments to the trial protocol. This leads to more efficient trials, with a higher likelihood of success.
Optimized Drug Manufacturing
Artificial intelligence in the pharmaceutical industry can enhance drug manufacturing processes by predicting equipment failures, optimizing production schedules, and ensuring consistent product quality. By analyzing data from sensors and manufacturing systems, artificial intelligence in drug manufacturing can identify patterns that indicate potential issues, allowing for preventive maintenance and reducing downtime.
Additionally, AI pharma can optimize production parameters to maximize yield and minimize waste, ensuring that drugs are produced efficiently and to the highest standards. This results in cost savings and a more reliable supply of high-quality medications.
Advanced Biomarker Discovery
AI and pharma together empower the identification of novel biomarkers, which are essential for diagnosing diseases, predicting treatment responses, and developing targeted therapies. By analyzing vast and complex biological datasets, AI in the pharmaceutical industry can uncover intricate patterns and markers that are often missed by traditional methods.
This capability allows for more precise and personalized medical interventions, significantly enhancing the effectiveness of treatments. Furthermore, it reduces the likelihood of adverse reactions, ensuring better patient outcomes and advancing the development of next-generation therapies.
Improved Patient Monitoring and Adherence
Artificial intelligence tools can monitor patient mental health and treatment adherence through wearable devices and mobile applications. By continuously collecting and analyzing data, AI can detect early signs of adverse reactions or health deterioration, enabling timely interventions.
Additionally, AI solutions for pharma include personalized reminders and support to help patients adhere to their prescribed treatment regimens. The utilization of AI for pharmaceutical industry improves overall treatment effectiveness and reduces the risk of complications, leading to better patient outcomes and lower healthcare costs.
Top 10 Use Cases of AI in the Pharmaceutical Industry
AI in pharma industry assists companies to widen the horizon of their methodology in order to gain competitive advantages. Let’s explore how pharmaceutical artificial intelligence helps pharma companies elevate their operations, enhance productivity, and drive innovation across the industry.
Predictive Toxicology
AI-driven predictive toxicology leverages machine learning algorithms to assess the safety profiles of drug candidates early in the development process. By analyzing chemical structures and biological interactions, these models can identify potential toxic effects before clinical trials commence.
This foresight allows pharmaceutical companies to eliminate hazardous compounds early, thereby reducing the risk of adverse outcomes and minimizing the financial and temporal investments associated with late-stage failures.
Drug Repurposing
The application of AI in drug repurposing involves mining extensive biomedical databases to uncover new therapeutic applications for existing medications. Machine learning models can identify previously unrecognized interactions between drugs and various biological targets, facilitating the discovery of alternative uses. This strategy not only accelerates the development timeline but also capitalizes on established safety profiles, making it a cost-effective approach to expanding treatment options.
Supply Chain Optimization
AI and pharma go hand in hand to revolutionize operations beyond drug discovery. AI enhances pharmaceutical supply chain management by predicting demand fluctuations, optimizing inventory levels, and streamlining distribution logistics. Advanced analytics and machine learning algorithms analyze historical sales data, market trends, and external factors to forecast future needs accurately. This precision ensures that medications are consistently available to patients while reducing excess inventory and minimizing waste, ultimately improving operational efficiency and reducing costs.
Real-World Evidence Generation
Utilizing AI to generate real-world evidence involves analyzing vast amounts of data from sources such as electronic health records, insurance claims, and patient registries. Machine learning techniques can identify patterns and correlations that provide insights into drug effectiveness and safety in diverse populations.
This evidence supports regulatory decision-making, informs clinical guidelines, and enhances post-market surveillance, ensuring that treatments deliver the desired outcomes in real-world settings.
AI-Assisted Formulation Development
AI-assisted formulation development utilizes artificial intelligence to predict the behavior of different combinations of active ingredients and excipients. By simulating various formulation scenarios, AI can identify optimal compositions that enhance drug stability, efficacy, and manufacturability.
This capability accelerates the formulation process, reduces the need for extensive laboratory testing, and ensures the development of high-quality pharmaceutical products.
Virtual Screening
In the realm of virtual screening, AI technologies analyze extensive chemical libraries to identify promising drug candidates. Machine learning models evaluate the interactions between numerous compounds and biological targets, prioritizing those with the highest potential for efficacy. This computational approach significantly reduces the time and resources required for experimental screening, enabling researchers to focus on the most viable candidates for further development.
Patient Recruitment
AI in the pharmaceutical industry optimizes patient recruitment for clinical trials by analyzing diverse datasets to identify individuals who meet specific inclusion and exclusion criteria. The utilization of machine learning in the pharmaceutical industry assess electronic health records, genetic information, and demographic data to pinpoint suitable candidates efficiently. This targeted recruitment approach enhances the speed and accuracy of enrolling participants, thereby accelerating the initiation and progression of clinical trials.
Regulatory Compliance
Ensuring regulatory compliance is streamlined through AI by automating the analysis of documents and processes against established guidelines. Natural language processing (NLP) and machine learning algorithms can review submissions, identify discrepancies, and ensure adherence to regulatory standards.
Additionally, AI systems can monitor regulatory changes and predict future requirements, enabling pharmaceutical companies to maintain compliance proactively and avoid costly penalties.
Automated Literature Review
AI facilitates automated literature reviews by employing machine learning and natural language processing to scan, categorize, and summarize vast volumes of scientific research. This capability added to an AI pharmaceutical software allows researchers to stay current with the latest advancements and integrate relevant findings into their projects efficiently.
By automating the time-consuming process of literature review, AI enables faster knowledge acquisition and informed decision-making in drug development.
Pharmacovigilance
AI enhances pharmacovigilance by systematically analyzing reports of adverse drug reactions from multiple sources, including social media, electronic health records, and patient feedback platforms. Advanced algorithms detect emerging safety signals and trends, enabling timely identification and response to potential issues. This proactive approach ensures ongoing monitoring of drug safety post-market, safeguarding patient health and maintaining public trust in pharmaceutical products.
These use cases illustrate the diverse applications of artificial intelligence in the pharmaceutical industry, highlighting how advanced technologies can drive innovation, efficiency, and safety throughout the drug development and distribution processes.
Real-Life World Examples of AI in the Pharmaceutical Industry
Here are some real-life examples of AI applications in the pharmaceutical industry that are revolutionizing drug development, optimizing clinical trials, and enhancing patient care. These innovations are driving efficiency and improving outcomes across the sector. Let’s explore!
AstraZeneca
AstraZeneca is leveraging AI technology to advance its drug discovery efforts. Using AI platforms, AstraZeneca can analyze extensive scientific literature and clinical data to uncover novel drug targets and potential treatments. This approach has already led to the discovery of new drug candidates for complex diseases such as chronic kidney disease and idiopathic pulmonary fibrosis.
AI enables AstraZeneca to apply a data-driven methodology to drug development, accelerating the process and improving the chances of finding effective treatments for challenging conditions.
GlaxoSmithKline (GSK)
GlaxoSmithKline is incorporating AI into its drug discovery pipeline to enhance its research capabilities. By utilizing AI to design and optimize new drug candidates, GSK has achieved notable successes, including identifying a promising molecule that progressed to clinical trials.
This AI-driven approach allows GSK to streamline the drug development process, reducing timeframes and increasing the likelihood of successful outcomes. The integration of AI into GSK’s R&D efforts aims to accelerate the availability of innovative treatments and improve patient care.
Novartis
Novartis is utilizing AI to revolutionize its approach to drug development and personalized medicine. By analyzing large and complex datasets with AI technologies, Novartis gains valuable insights into disease mechanisms and patient responses. This enables the creation of more personalized treatment plans and enhances the efficiency of drug development.
AI helps Novartis refine its strategies, leading to more targeted therapies and improved patient outcomes. The use of artificial intelligence in the pharmaceutical industry is prominent in AI in Novartis’s AI-based Novartis’s research efforts representing significant advancement in the industry’s approach to developing new therapies.
Sanofi
Sanofi is at the cutting edge of integrating AI and machine learning into its drug discovery and development processes. AI technologies enable Sanofi to optimize the identification of drug candidates and enhance the efficiency of its research efforts. By predicting how compounds interact with biological targets, AI helps streamline the drug discovery pipeline and accelerate the development of new treatments.
This innovative approach allows Sanofi to bring new therapies to market more quickly and address unmet medical needs with greater effectiveness.
Challenges While Leveraging AI in Pharmaceutical
Let’s examine the challenges that businesses encounter when implementing AI in the pharmaceutical industry, such as reproducibility, regulatory hurdles, and ethical dilemmas. Addressing these issues thoughtfully is crucial for ensuring responsible and effective use of AI technology.
Challenge – Reproducibility of results
The integration of AI in drug discovery raises concerns about the reproducibility of results. AI models trained on specific datasets may not perform consistently across different populations or under varying conditions, leading to unreliable drug development outcomes.
Solution
Pharmaceutical companies should standardize AI training datasets and validate models across diverse patient demographics and conditions. Establishing industry-wide benchmarks for AI reproducibility and transparency in methodology can help mitigate these challenges and ensure consistent performance across the board.
Challenge – Lack of high-quality medical data
AI-driven clinical trials can be hindered by the lack of high-quality, labeled medical data, which is crucial for training accurate models. This scarcity of data can result in suboptimal AI performance, potentially impacting trial outcomes and patient safety.
Solution
To address data scarcity, companies should invest in collaborative data-sharing initiatives that maintain patient privacy. Additionally, synthetic data generation and advanced data augmentation techniques can be employed to create robust training datasets, ensuring that AI models are well-equipped to handle diverse clinical scenarios.
Challenge – Privacy of sensitive patient data
The use of AI in personalized medicine poses ethical challenges, particularly concerning the privacy of sensitive patient data. AI systems that process vast amounts of personal health information can inadvertently expose patients to privacy breaches or misuse of their data.
Solution
Pharmaceutical companies must prioritize data security by implementing advanced encryption methods and strict access controls. Regular audits and compliance with global data protection regulations, such as GDPR, can help safeguard patient information, ensuring that privacy concerns are adequately addressed in AI-driven personalized medicine.
Challenge – Bias in decision-making
AI’s reliance on historical data for decision-making can perpetuate existing biases in the pharmaceutical industry, leading to skewed results in drug efficacy or adverse effects, especially for underrepresented groups.
Solution
To combat AI bias, pharmaceutical companies should actively seek diverse data sources and include representative samples from all demographics in AI training datasets. Regularly auditing AI systems for bias and integrating feedback loops can help identify and correct biases, promoting fair and equitable outcomes in drug development and patient care.
Challenge – AI compliance and the legal implications
The regulatory landscape for AI in pharmaceuticals is still evolving, leading to uncertainties about compliance and the legal implications of AI-driven decisions in drug approval and patient treatment.
Solution
Pharmaceutical companies should engage with regulatory bodies early in the AI development process to ensure compliance with emerging guidelines. Staying informed about regulatory updates and contributing to the development of AI-specific regulations can help companies navigate the evolving legal landscape and reduce the risk of non-compliance.
Also read: AI Regulation and Compliance in EU: Charting the Legal Landscape for Software Development
Generative AI in Pharma: The Future of Intelligent Drug Discovery and Beyond
As pharmaceutical companies continue overcoming the current challenges of AI adoption, a new frontier is taking shape — Generative AI (Gen AI). Unlike traditional AI, which analyzes existing data, Gen AI can create entirely new molecular structures, simulate complex biological interactions, and generate synthetic data to accelerate innovation.
This marks a significant shift in how pharma R&D operates. Gen AI can help scientists design novel compounds, predict protein folding, and even generate clinical trial protocols that reduce the time and cost of development. It brings a level of creativity and automation that was previously unattainable.
What Can Businesses Expect from Gen AI in Pharma?
Faster drug discovery: Gen AI can generate thousands of compound variations in minutes, narrowing down candidates far more efficiently than traditional methods.
- Synthetic data for model training: It enables the creation of high-quality synthetic medical data to overcome privacy and scarcity concerns.
- Personalized medicine design: Gen AI models can simulate individual responses to drug candidates, helping create more personalized and effective treatment plans.
- Streamlined regulatory documentation: Automating the generation of compliant documentation for trials and approvals can drastically reduce administrative load.
According to a McKinsey report, Generative AI could unlock $60 billion to $110 billion in annual economic value for the pharmaceutical and medical-product industries. This impact is expected from productivity boosts across the entire pharma value chain — from faster identification of drug candidates to improved approval timelines and more efficient marketing strategies.
Gen AI isn’t just the next step in pharmaceutical AI — it’s a paradigm shift that transforms how innovation happens. Businesses that begin experimenting now will be better positioned to lead the future of life sciences.
How Appinventiv Can Help You In Developing AI-based Platforms for Your Pharmaceutical Business
Appinventiv, a leading AI development company, is dedicated to helping businesses in the pharmaceutical industry leverage the power of artificial intelligence. With our deep expertise in AI, we develop tailored solutions that accelerate drug discovery, optimize clinical trials, and streamline operational processes.
Our advanced analytics tools help pharmaceutical companies uncover critical insights from complex datasets, improving decision-making and driving better outcomes. We also focus on AI-driven automation to enhance productivity and reduce costs, ensuring your operations run smoothly and efficiently. Additionally, Appinventiv prioritizes compliance and security, ensuring that all AI solutions meet regulatory requirements and safeguard sensitive pharmaceutical data.
Over our decade-long experience, we have successfully driven hundreds of healthcare projects, driving innovation and improving patient care. Some of our notable collaborations include YouComm, a patient communication platform, Health-e-People, a solution enhancing patient engagement and data sharing, DiabeticU, a diabetes management platform, and Soniphi, a personal wellness system.
By partnering with Appinventiv, businesses in the pharmaceutical sector can harness cutting-edge AI technology to innovate and stay ahead in a highly competitive industry.
FAQs
Q. How is AI used in the pharmaceutical industry?
A. Artificial intelligence in pharma is used in different ways in the industry, including:
- Drug Discovery and Development: AI accelerates the drug discovery process by analyzing vast datasets to identify potential drug candidates, predict their effectiveness, and optimize chemical structures. This leads to faster and more cost-effective drug development.
- Clinical Trials: Artificial intelligence in pharma helps design and manage clinical trials by selecting suitable participants, predicting outcomes, and monitoring data in real-time. This results in more efficient and successful trials.
- Personalized Medicine: AI in the pharmaceutical industry analyzes patient data, including genetic information, to develop personalized treatment plans, ensuring that therapies are tailored to individual needs.
- Predictive Analytics: AI in the pharmaceutical industry is used to forecast disease progression, patient responses to treatments, and potential side effects, allowing for better decision-making and improved patient outcomes.
Q. Why is AI important in the pharmaceutical industry?
A. AI is important in the pharmaceutical industry because it enhances efficiency, reduces costs, and accelerates the development of new drugs. By automating complex processes, AI allows researchers to focus on innovative solutions, leading to breakthroughs in medicine. Additionally, AI enables the creation of personalized treatments, improving patient outcomes and advancing the overall healthcare industry.
Q. What is the future of AI pharma in drug discovery?
A. The future of AI in the pharmaceutical industry is expected to revolutionize drug discovery by accelerating the identification of new drug candidates. AI algorithms will analyze complex biological data to predict potential drug interactions and efficacy, leading to faster and more accurate discoveries. This will significantly shorten development times and reduce costs.
Q. What are the ethical considerations when using AI for patient data analysis in pharma?
A. Using AI for patient data analysis in the pharmaceutical industry raises several ethical considerations:
- Data Privacy and Security: Protecting patient data from unauthorized access and breaches is critical. AI systems must comply with strict regulations like GDPR and HIPAA to ensure that sensitive information is securely handled and anonymized where necessary.
- Bias and Fairness: AI algorithms may unintentionally incorporate biases present in the data, leading to unfair treatment of certain patient groups. Ensuring that AI models are trained on diverse datasets and regularly audited for bias is essential to maintain fairness.
- Informed Consent: Patients must be fully informed about how their data will be used by AI systems. Transparent communication regarding data collection, analysis, and usage helps in gaining patient trust and ensuring ethical compliance.
- Accountability: Determining who is responsible when AI-driven decisions negatively impact patient outcomes is a key ethical concern. Clear guidelines on accountability and oversight are necessary to address this issue.
- Transparency: AI algorithms should be transparent and explainable, especially when used in decision-making processes that affect patient care. Stakeholders should understand how AI conclusions are reached to ensure trust in the system.
Q. How can AI assist in improving the accuracy and efficiency of pharmaceutical marketing strategies?
A. AI enhances pharmaceutical marketing by enabling targeted marketing through advanced patient segmentation and personalized messaging. Predictive analytics help forecast market trends and adjust strategies proactively. AI also optimizes content by assessing its effectiveness and generating tailored material. Accurate sales forecasting through AI improves resource allocation and inventory management. Additionally, AI-driven CRM tools offer insights into customer behavior, fostering more personalized interactions and increasing customer loyalty.
Q. What are the top AI adoption trends in pharma for 2025?
A. AI adoption in pharma is accelerating rapidly in 2025, driven by both innovation and necessity.
- Generative AI in early-stage R&D – Pharma companies are increasingly using Gen AI to design novel drug candidates, simulate protein-ligand interactions, and identify promising compounds in silico, reducing the need for extensive lab trials.
- AI-powered clinical trials – AI is streamlining trial recruitment by identifying ideal patient cohorts, predicting dropout rates, and optimizing trial site selection based on real-world data, speeding up trial timelines and improving success rates.
- Synthetic data and privacy-safe modeling – With increasing concerns around patient data privacy, synthetic data generation is on the rise, helping AI models train effectively while staying compliant with GDPR and HIPAA.
- Regulatory automation with AI – Companies are using AI to generate regulatory documents, analyze compliance risks, and prepare submissions faster, reducing manual workload and approval delays.
- Precision and personalized medicine – AI algorithms are being used to match treatments to individual genetic profiles and health records, making therapies more effective and minimizing adverse effects.
Q. How much cost and time can AI save pharmaceutical companies?
A. AI can substantially reduce both the time and cost involved in pharmaceutical operations, though the actual savings vary depending on the specific application, scale, and maturity of implementation. For instance, AI can potentially cut drug discovery timelines by 30–50% and lower R&D costs by 20–40% in many cases. From accelerating target identification to automating clinical trial design and regulatory documentation, the benefits are wide-ranging—but not uniform. Since outcomes depend heavily on how AI is applied, partnering with an experienced AI development firm can offer clearer insights, realistic timelines, and tailored cost-saving strategies for your use case.
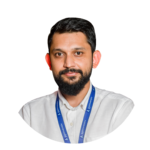
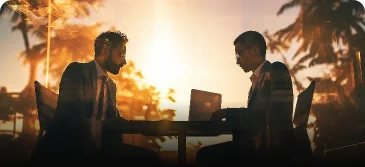
- In just 2 mins you will get a response
- Your idea is 100% protected by our Non Disclosure Agreement.
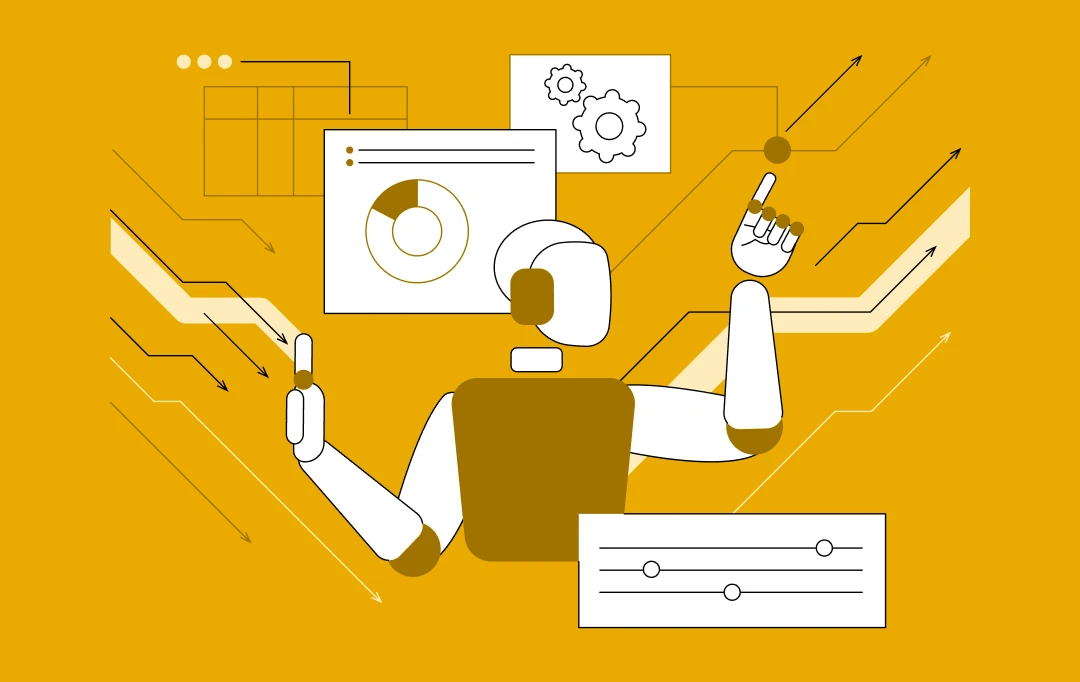
How to Integrate AI with EHR/EMR Systems for Streamlined Healthcare Operations
Key takeaways: Not adopting AI in EHR/EMR today risks falling behind. AI streamlines EHR workflows, cutting clinical and admin delays. Real-world hospitals are already seeing faster, smarter care with AI. Seamless AI integration needs system checks, data prep, and training. AI in EHR tackles real challenges like bias, cost, and compliance. Custom AI integration costs…
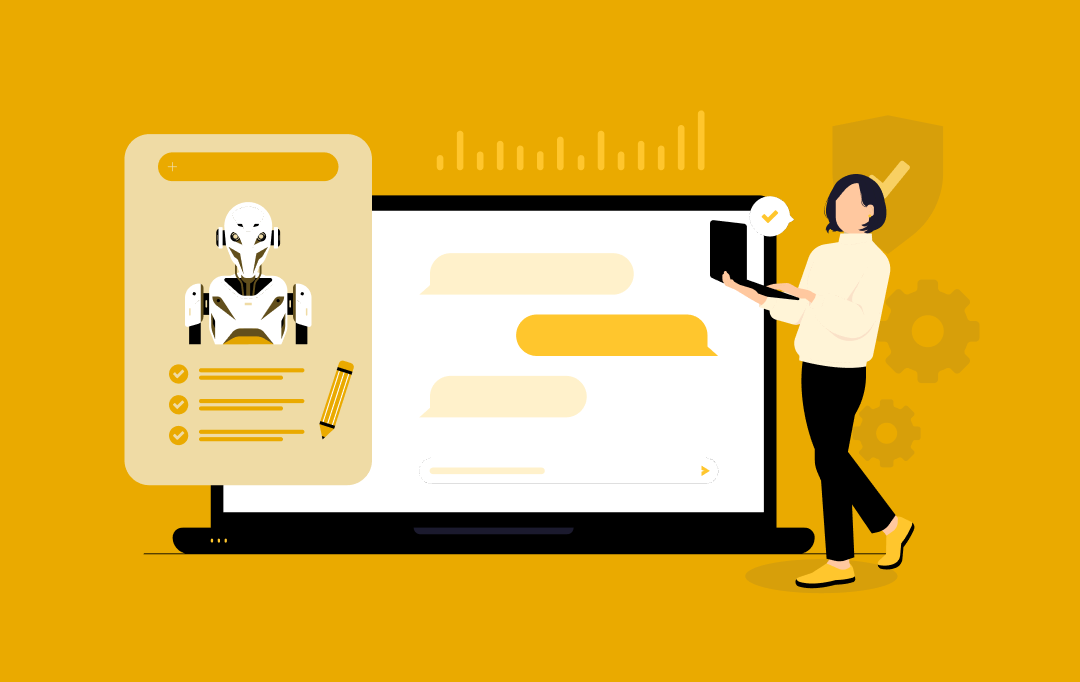
AI Agent-Driven UIs: Replacing App Menus and Buttons
Key takeaways: Challenge: Static menus and buttons also become outdated because people are frustrated by limited, multi-stage navigation. Solution: The AI Agent interacts with the user through a friendly UI that does not require cludgy menus but conversational and context-sensitive navigation. The Way AI Agents Work: AI agents establish a basis of natural language understanding,…
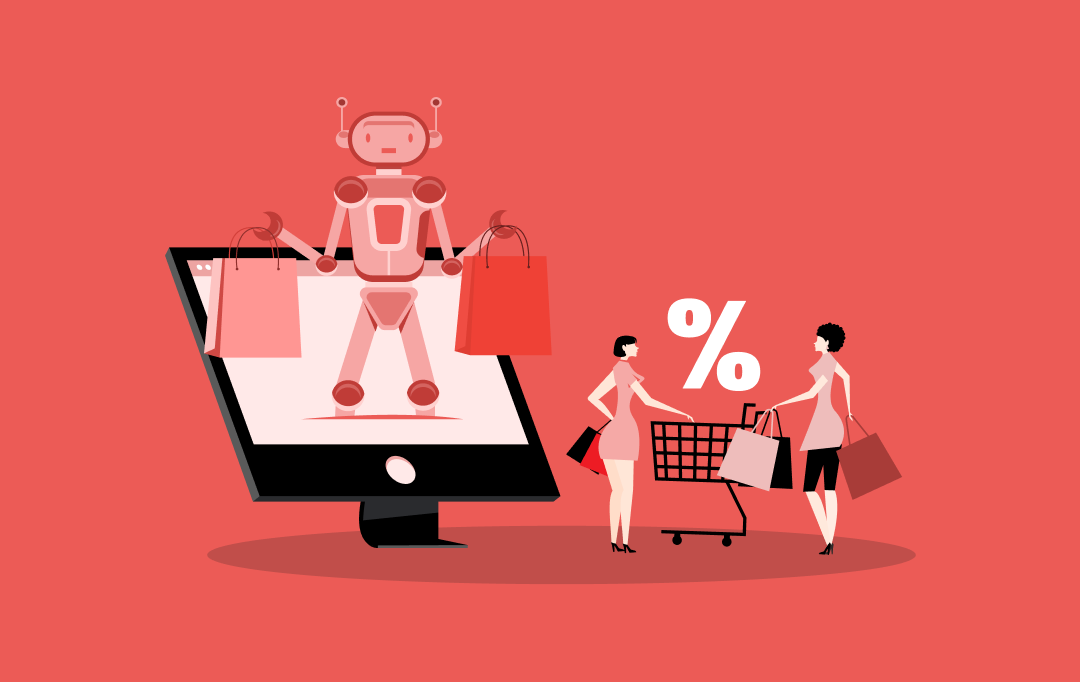
10 Use Cases and Benefits of How AI Agents Are Revolutionizing the Retail Industry
Key takeaways: AI agents in the retail industry are revolutionizing businesses by enhancing customer experience and streamlining operations. From personalized recommendations to predictive analytics, AI offers tangible benefits like increased conversions and reduced costs. AI adoption can lead to scalable growth and a competitive edge in the retail industry. Retailers must consider data strategies, talent…