- How is AI used in genomics?
- Machine Learning and Deep Learning
- Natural Language Processing (NLP)
- Genetic Variant Analysis
- AI in CRISPR and Gene Editing
- Integration of Genomic Data
- AI-Enhanced Genomic Sequencing
- 10 Use Cases of AI in Genomics
- AI Applications in Functional Genomics
- AI in Clinical and Genomic Diagnostics
- Generative AI for Genomics
- AI in Gene Editing
- AI for Personalized Medicine
- Drug Discovery and Development
- Population Genomics Studies
- AI-Powered Genome Assembly
- Disease Risk Prediction
- AI for Epigenetics Research
- Benefits of AI in Genomics
- Improved Data Analysis
- Quicker Discoveries
- Enhanced Accuracy in Gene Editing
- Personalized Medicine
- Cost Efficiency
- How AI is Transforming Genomic
- AI-Powered Multi-Omics Integration
- Quantum Computing in Genomic Analysis
- AI for Modeling Complex Diseases
- AI-Driven Synthetic Biology
- Challenges Faced When Using AI in Genomics
- Ethical Issues
- Computational Constraints
- Data Privacy
- Looking Forward: The Evolving Landscape of Genomics with AI
- FAQs
AI has become the real MVP, shaking up industries, and genomics is no exception. The tech is revamping the landscape of medical research & operational capabilities in healthcare.
In Genomics – AI is simply playing the role of a pro analyst who turns complex genetic data into groundbreaking insights. AI employs machine learning, data mining, and pattern recognition to analyze large genomic datasets, and that is an advancing field of progress in gene editing, clinical diagnosis, and functional genomics.
From anticipating diseases before they emerge, personalizing medications based on genetic composition, and uncovering life’s mysteries quickly, AI in genomics is speeding this up, resulting in quick, precise, and cheaper discoveries. Thus, Artificial Intelligence in genomics is causing a revolutionary change in the future of healthcare and research.
Market overview: The overall market valuation of AI in genomics was USD 484.1 million in 2022 and is anticipated to increase at a DA of 39.2%, reaching USD 12.5 billion by 2032.
Dive into this blog to uncover 10 epic ways AI is transforming genomics and changing how we decode the secrets of life—one gene at a time. Get ready to see medicine’s future unfold right before you!
Transform complex genetic data into game-changing insights and keep your ops running smoothly.
How is AI used in genomics?
Artificial intelligence is completely changing the field of genomics by using more advanced technology to increase the efficiency of data processing, precision of research, and rapid discoveries. Here is the role of AI for genomics:
Machine Learning and Deep Learning
AI methods such as ML and DL are utilized to analyze large genomic datasets. Machine learning in healthcare is made to detect complex patterns in gene sequences, such as mutation patterns linked to diseases, among others. Deep learning models, especially neural networks, can identify complex associations in high-dimensional genomic information, enhancing the precision of gene variant analysis.
Natural Language Processing (NLP)
Techniques in NLP are employed to extract and examine unstructured genomic data from research articles, medical documentation, and various textual references. This aids in obtaining valuable insights and recognizing connections between genetic variations and clinical conditions. The use cases of NLP in healthcare enhance healthcare operations and patient engagement.
Genetic Variant Analysis
AI aids in analyzing genetic variants by referencing them against established databases like ClinVar or gnomAD. Algorithms assess if a genetic variation is harmful, harmless, or uncertain, helping clinicians make informed choices for diagnosis and treatment.
AI in CRISPR and Gene Editing
AI technologies are improving the accuracy of gene-editing tools like CRISPR. The machine learning algorithms identify the most suitable targets for gene editing, optimally performing the edit and reducing off-target effects. This provides safer and more effective gene therapies.
Integration of Genomic Data
AI assists in merging numerous different sorts of genomic data, such as DNA sequences, RNA expression information, and protein structures. By integrating diverse genomic datasets, AI offers a comprehensive view of biological processes, enhancing disease progression and drug response predictions.
AI-Enhanced Genomic Sequencing
AI speeds up NGS by automating the entire process, including data preprocessing, alignment, and variant detection. This brings mistakes made by human beings to a minimum and shortens the process of data gathering to practical outcomes. AI technologies like machine learning, natural language processing, and deep learning significantly contribute to genomics by advancing data analytics in healthcare, increasing gene-editing accuracy, and providing an in-depth understanding of intricate genetic data.
10 Use Cases of AI in Genomics
AI has profoundly transformed genomics by offering groundbreaking methods for examining intricate datasets, forecasting results, and improving research accuracy. Here are 10 applications of AI for genomics, illustrating the transformative potential of AI.
AI Applications in Functional Genomics
AI applications in functional genomics are crucial for aiding scientists in comprehending the functions of genes and their interactions in biological systems. AI methods, including deep learning architectures, allow forecasting protein-DNA interactions, providing important information about gene functions. These tools enable a more precise identification of regulatory pathways and mechanisms of gene expression.
- AI models can rapidly analyze large volumes of genetic data to pinpoint essential gene functions.
- AI aids in comprehending gene regulation by forecasting protein-DNA interactions.
- Incorporating AI for genomics speeds up research, minimizing the time needed for functional gene examination.
- As AI progresses in functional genomics, it offers quicker and more precise insights, ultimately enhancing the rate at which scientists discover genetic functions and their effects on diseases.
Real Example: AlphaFold by DeepMind
DeepMind’s AlphaFold is an innovative AI technology that makes accurate predictions about protein structure, which is critical to understanding how genes work. From modeling the process of protein folding with AI, AlphaFold is helping scientists better understand the molecular workings behind differing biological functions and diseases. It contributes to functional genomics studies.
AI in Clinical and Genomic Diagnostics
Artificial intelligence is a revolutionary, allied to clinical and genomic diagnostics, that is employed in diagnosing diseases by detecting genetic mutations matching to various diseases. Its technology helps doctors quickly identify diseases, including cancer and other rare genetic diseases, to devise personalized treatment strategies aligned with particular genetic attributes.
- Artificial intelligence utilizes genomic data to find genetic markers associated with diseases.
- Timely identification via AI enhances medical diagnostic precision and therapeutic approaches.
- Artificial intelligence for genomics aids in identifying rare diseases by examining genetic sequences that conventional approaches might overlook.
- Integrating artificial intelligence in clinical genomics allows healthcare providers to identify diseases sooner and more precisely, enhancing patient care and results.
Real Example: IBM Watson for Cancer Treatment
IBM Watson for Oncology utilizes AI to evaluate genetic information and medical research to aid in cancer diagnosis. It detects DNA mutations and suggests tailored treatment choices, enhancing diagnostic precision and assisting physicians in creating efficient treatment strategies for cancer patients.
Generative AI for Genomics
Generative AI for healthcare is crucial for modeling biological processes, speeding up research in drug discovery, and protein modeling. AI tools such as AlphaFold have transformed protein structure prediction, providing excellent accuracy in comprehending protein folding, one of the toughest challenges in genomics.
- Generative AI models do protein folding to improve drug discovery.
- AI tools support modeling biological systems and give insight into complicated genetic mechanisms.
- Generative AI in genomics showcases its transformative role by accurately modeling biological functions and predictions.
AI in Gene Editing
AI is essential in advancing gene editing technologies like CRISPR, enhancing their precision and safety. AI algorithms forecast off-target impacts, enabling scientists to improve the accuracy of gene editing targets. This minimizes the risks linked to genetic modifications and guarantees that gene editing is carried out accurately.
- AI algorithms enhance CRISPR-Cas9 applications by forecasting off-target effects.
- AI-driven tools pinpoint optimal genetic targets, minimizing the chances of unintentional changes.
- Through enhanced accuracy, AI guarantees superior results in genome editing, regardless of whether for research or treatment aims.
- With the assistance of AI, gene editing technologies such as CRISPR are becoming increasingly efficient and dependable, resulting in safer and more precise genetic alterations.
Real Example: CRISPR-Cas9, along with Intel’s AI Integration
The CRISPR-Cas9 gene-editing technology will progressively be integrated with AI. For instance, Intel partners with biotech firms to enhance CRISPR’s accuracy by using AI to predict and minimize off-targeting effects, promoting targeted and efficient genetic modifications.
Bonus Read: Learn How Biotech Firms Use AI in Biotechnology
AI for Personalized Medicine
AI is revolutionizing personalized medicine by customizing therapies according to each person’s biological composition. By examining a patient’s genetic data, AI can recommend the most appropriate treatment, particularly in oncology.
- AI systems examine genetic information to suggest customized treatment alternatives.
- AI-powered platforms are dedicated to formulating personalized oncology therapies for cancer patients.
- As such, AI enhances treatment effectiveness by considering that a patient’s genetic profile is distinctive.
- AI for genomics enables healthcare providers to deliver tailored therapies, enhancing treatment outcomes.
Real Example: Tempus Precision Healthcare System
Tempus uses AI in clinical and genomic diagnostic data to analyze and establish personalized cancer treatment strategies. Analyzing a patient’s genetic makeup allows Tempus to give insight into the most promising therapies, leading to better oncology outcomes.
Drug Discovery and Development
AI shortens cancer research timelines by identifying drug targets within vast genomic datasets, accelerating drug discovery. Thus, AI tools pace up from initial discovery to clinical trials by analyzing huge amounts of genetic material.
- Artificial intelligence examines genomic information to discover new drug targets.
- AI algorithms forecast the efficacy of prospective drug compounds.
- With the help of AI, the timelines for vaccine and drug development are greatly accelerated.
- In particular, the use of AI in drug discoveries expedites pharmaceutical advances for patients’ quicker access to these therapies.
Real Example: Insilico Medicine’s AI for drug discovery
Insilico Medicine uses AI for drug discovery, which can accelerate the drug discovery process for novel drug targets. The AI platform analyzes massive amounts of genomic data to predict how the compound will work. It expedites its development in therapy creation as vaccines to cancer therapies.
Population Genomics Studies
AI facilitates extensive population genomics research by examining and interpreting large datasets to uncover genetic trends associated with diseases. Using AI, scientists can analyze genetic markers in various populations, identifying links between genes and illnesses.
- AI tools examine genetic information from various populations to recognize disease-associated genetic markers.
- The capacity of AI to analyze extensive datasets improves the examination of genetic variation.
- AI for genomics enhances research, allowing for the identification of genetic patterns within extensive populations.
Incorporating artificial intelligence in genomics is crucial for comprehending genetic diversity and pinpointing genetic risk factors for diseases across various populations.
Real Example: 23andMe and AncestryDNA
23andMe and AncestryDNA are well-known instances of utilizing AI in population genomics studies. These firms examine genetic information from millions of individuals to reveal patterns in genetics associated with diseases, lineage, and genetic characteristics, aiding in the exploration of genetic diversity at a population level.
AI-Powered Genome Assembly
AI enhances the genome assembly procedure by increasing the precision and rapidity of sequencing intricate genomes. AI-driven algorithms can forecast sequencing gaps and offer solutions to these issues, guaranteeing a more thorough genome assembly.
- AI-powered tools improve genome sequencing by anticipating missing segments in genetic information.
- AI models minimize errors in genome assembly, resulting in more precise outcomes.
- By incorporating AI, genome sequencing is accelerated and made more dependable.
- Artificial Intelligence in genomics is crucial for improving sequencing technologies, resulting in more accurate and faster genome assembly.
Real Example: Illumina’s Sequencing with AI Enhancements
Illumina is a prominent firm in genome sequencing, employing AI to enhance genome assembly. Their AI-powered tools forecast sequencing gaps and guarantee more precise genome assemblies, particularly for intricate genomes, which greatly minimizes errors in sequencing and improves genomic research.
Disease Risk Prediction
The task of disease risk prediction forms a high core of AI within the prospect of preventative healthcare. AI models project into probability the risk of a person for the development of specific diseases, including but not limited to cancer, heart disease, or diabetes, all through thorough analysis of genetic and lifestyle information.
- AI systems assess genetic variations and lifestyle factors to project probabilities of diseases.
- AI’s ability to predict allows for timely interventions and preventive actions.
- AI-driven personalized healthcare strategies assist in disease prevention before their onset.
- Incorporating AI facilitates more preventive healthcare, allowing people to take charge of their health through tailored risk evaluations.
Real Example: DiabeticU
Developed by Appinventiv, Diabetic U is the most advanced diabetes management app powered by artificial intelligence to make daily diabetes care efficient, personalized, and effective. AI-based insights will enable a better understanding of the condition from the user’s end and promote proactive health management.
There are tools for real-time glucose monitoring, medication tracking, and lifestyle management for an all-rounded approach to diabetes care. It equips users to make informed decisions and prevents complications by including AI-based features such as predictive alerts and health recommendations.
Dive into our success story with Diabeticu, an AI-powered app designed to simplify life for diabetics. See how we transformed challenges into innovative solutions for better health management.
AI for Epigenetics Research
AI also advances epigenetics research, exploring how environmental elements affect gene expression. AI models examine DNA methylation patterns and other epigenetic modifications, assisting researchers in revealing their effects on illnesses such as mental health conditions.
- AI tools analyze epigenetic alterations such as DNA methylation and histone modifications.
- AI aids in recognizing epigenetic markers associated with diseases, enhancing diagnostic precision.
- AI uses in genomics also reach epigenetics, creating new opportunities for gene regulation exploration.
- The use of AI in epigenetics research provides enhanced insights into the mechanisms of gene expression, furthering our knowledge of complicated diseases and conditions.
Real Example: Epigenetic Timekeeper by DNAstack
DNAstack is at the forefront of epigenetics research, employing AI to analyze DNA methylation patterns for insights into aging and various diseases. Their Epigenetic Clock employs AI to examine epigenetic markers in the genome, providing insights into how lifestyle choices and environmental factors influence gene expression and the progression of diseases.
These AI-driven tools and systems transform our comprehension and management of genomic data, steering us toward a future where precision medicine, advanced genetic research, and targeted therapies become standard practice.
Benefits of AI in Genomics
AI reveals substantial advantages for genomics, transforming how genomic data is analyzed and understood. Here’s how it benefits:
Improved Data Analysis
Artificial Intelligence for genomics, especially via machine learning and deep learning techniques, can assess extensive genomic data with increased precision and rapidity. These technologies assist in recognizing genetic patterns associated with illnesses, facilitating earlier diagnoses and more accurate treatments.
Quicker Discoveries
AI speeds up research by automating intricate processes such as data preprocessing, variant identification, and genome sequencing. This shortens the interval from data creation to actionable insights, resulting in quicker advancements in comprehending genetic disorders.
Enhanced Accuracy in Gene Editing
Artificial intelligence for genomics is boosting the precision and effectiveness of gene editing methods, including CRISPR. It forecasts ideal gene targets, reducing errors and enhancing the success rate of gene therapies.
Personalized Medicine
By examining a person’s specific genetic information, AI facilitates customized treatments. This ensures that therapies align with each patient’s genetic characteristics, minimize side effects, and enhance treatment results.
Cost Efficiency
The incorporation of AI minimizes the necessity for manual involvement in genomic studies, decreasing expenses linked to data evaluation and hastening the journey toward bringing genomic-based healthcare innovations to market.
How AI is Transforming Genomic
As AI progresses, its involvement in genomics is growing through new technologies and operational improvements that are set to influence the discipline’s future. Here’s a glance at a few forthcoming trends:
AI-Powered Multi-Omics Integration
The AI and genomics futures combine various omics data genomics, transcriptomics, proteomics, and metabolomics into cohesive, AI-driven systems. By examining these layers collectively, AI will offer enhanced insights into intricate diseases, allowing for a more comprehensive understanding of human biology and disease mechanisms.
Quantum Computing in Genomic Analysis
The integration of quantum computing with artificial intelligence in genomics has the potential to transform the efficiency and precision of genomic data processing. Quantum algorithms can process vast datasets significantly quicker than conventional computers, speeding up operations like genomic sequencing, variant detection, and gene-drug interaction evaluation. This may result in advancements in real-time precision medicine.
AI for Modeling Complex Diseases
AI application in functional genomics will continue to progress with the creation of models that replicate complex diseases on a molecular scale. By examining extensive genetic, clinical, and environmental information, AI for genomics aims to forecast the progression of diseases like cancer, Alzheimer’s, and uncommon genetic conditions, which could result in quicker diagnoses and improved treatment options.
AI-Driven Synthetic Biology
The combination of AI in genomics and synthetic biology is poised to speed up the development of genetically modified organisms and biotherapeutics. The AI model for genomics will enhance the creation of synthetic genes, metabolic pathways, and microbial systems, facilitating the advancement of bioprocesses, vaccines, and various biopharmaceuticals.
In the next few years, the convergence of AI in genomics with new technologies such as quantum computing, synthetic biology, and multi-omics integration will reshape the field, resulting in quicker advancements in personalized medicine, intricate disease therapies, and gene modification.
Challenges Faced When Using AI in Genomics
Despite its potential uses, using AI in genomics presents unique difficulties. Among the primary challenges are:
Ethical Issues
Using artificial intelligence in clinical and genomic diagnostics raises ethical dilemmas regarding data privacy, consent, and the risk of discrimination. Given access to sensitive genetic data, ensuring that AI systems are utilized responsibly and ethically is a significant concern.
Computational Constraints
Genomic information is highly intricate and demands substantial computational resources for analysis. Not every institution possesses the essential infrastructure, which may hinder the broad implementation of AI tools.
Data Privacy
Genomic information is extremely personal, and its utilization must adhere to strict privacy laws. It is crucial to guarantee that artificial intelligence in genomics complies with data privacy regulations and safeguards individuals’ genetic data.
Our powerhouse team of AI pros is locked, loaded, and ready to deliver tailored AI innovations designed to match your exact goals
Looking Forward: The Evolving Landscape of Genomics with AI
Incorporating artificial intelligence in genomics is transforming the domain, creating new possibilities for discovery, precision, and individualized therapies. AI improves data analysis, increases diagnostic accuracy, and speeds up genomic studies, revolutionizing healthcare.
Although it holds promise, issues such as ethics, data privacy, and computational constraints must be resolved to completely utilize AI’s potential in genomics. With the advancement of collaboration between AI and genomics, we anticipate revolutionary findings that may transform medicine and enhance health results worldwide.
Working with an artificial intelligence development company like Appinventiv offers significant advantages for delving deeper into advancements in AI and genomics. Appinventiv is a leader in AI and machine learning solutions, focusing on creating innovative AI-powered applications that boost efficiency and promote innovations across various industries.
Their expertise in healthcare enables them to help you navigate the complexities of AI integration in genomics, offering tailored solutions that accelerate research and improve medical outcomes. Partnering with Appinventiv can be a pivotal step in changing your genomics approach.
FAQs
Q. How is AI used in gene editing?
A. AI is used in gene editing to enhance the accuracy and efficiency of selecting specific genes to be targeted for modification. Machine learning algorithms predict genetic changes’ effects, accelerating the development of better gene therapies.
Q. What are the main advantages of AI in genomics?
A. AI increases the speed of genomic data processing, thus increasing accuracy in gene sequencing, predicting the impact of genetic variations on health, and personalized medicine by identifying genetic predispositions to diseases.
Q. How does AI help with genomic data analysis?
A. AI algorithms scan huge collections of genomic data to determine patterns, mutations, and correlations that may not be so discernible to the human eye. This yields more accurate predictions and insights into genetic diseases and treatments.
Q. What does AI contribute to the identification of new drugs?
A. AI in genomics helps discover drugs by going through genomic data to develop potential drug targets. AI models can tell how genetic mutations alter disease pathways, hence the ability to design more focused research therapies.
Q. Can AI help diagnose genetic disorders?
A. AI diagnosis of genetic disorders can help by using sequencing data to find mutations that cause diseases. Machine learning models can identify rare genetic disorders faster and more accurately than any other method.
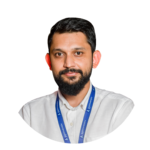
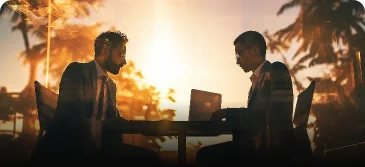
- In just 2 mins you will get a response
- Your idea is 100% protected by our Non Disclosure Agreement.
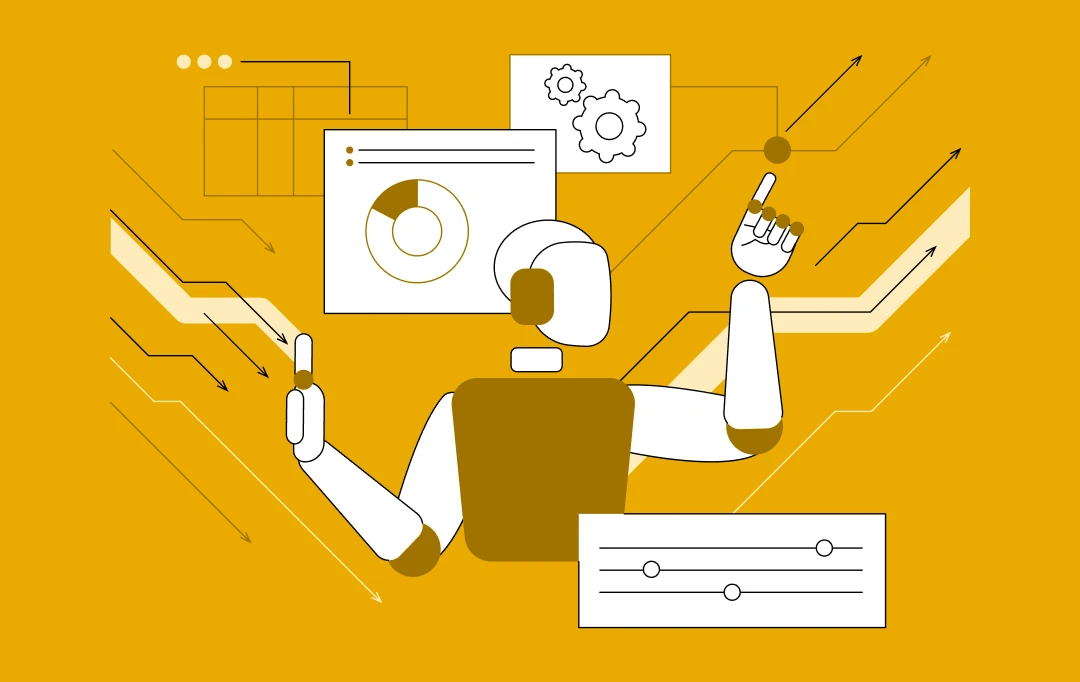
How to Integrate AI with EHR/EMR Systems for Streamlined Healthcare Operations
Key takeaways: Not adopting AI in EHR/EMR today risks falling behind. AI streamlines EHR workflows, cutting clinical and admin delays. Real-world hospitals are already seeing faster, smarter care with AI. Seamless AI integration needs system checks, data prep, and training. AI in EHR tackles real challenges like bias, cost, and compliance. Custom AI integration costs…
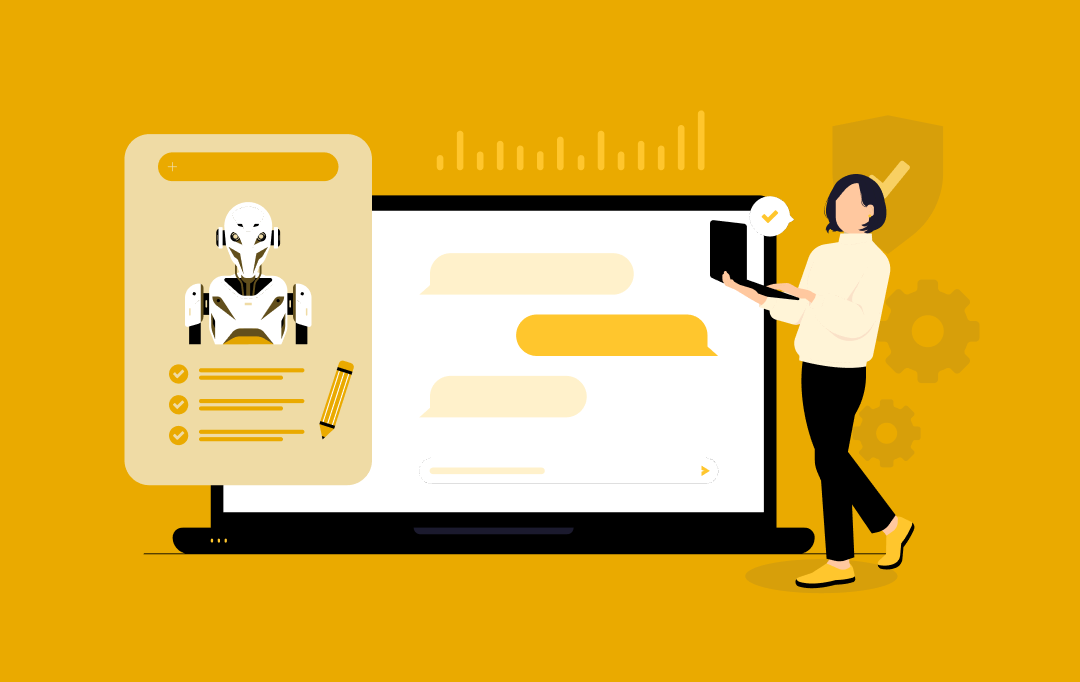
AI Agent-Driven UIs: Replacing App Menus and Buttons
Key takeaways: Challenge: Static menus and buttons also become outdated because people are frustrated by limited, multi-stage navigation. Solution: The AI Agent interacts with the user through a friendly UI that does not require cludgy menus but conversational and context-sensitive navigation. The Way AI Agents Work: AI agents establish a basis of natural language understanding,…
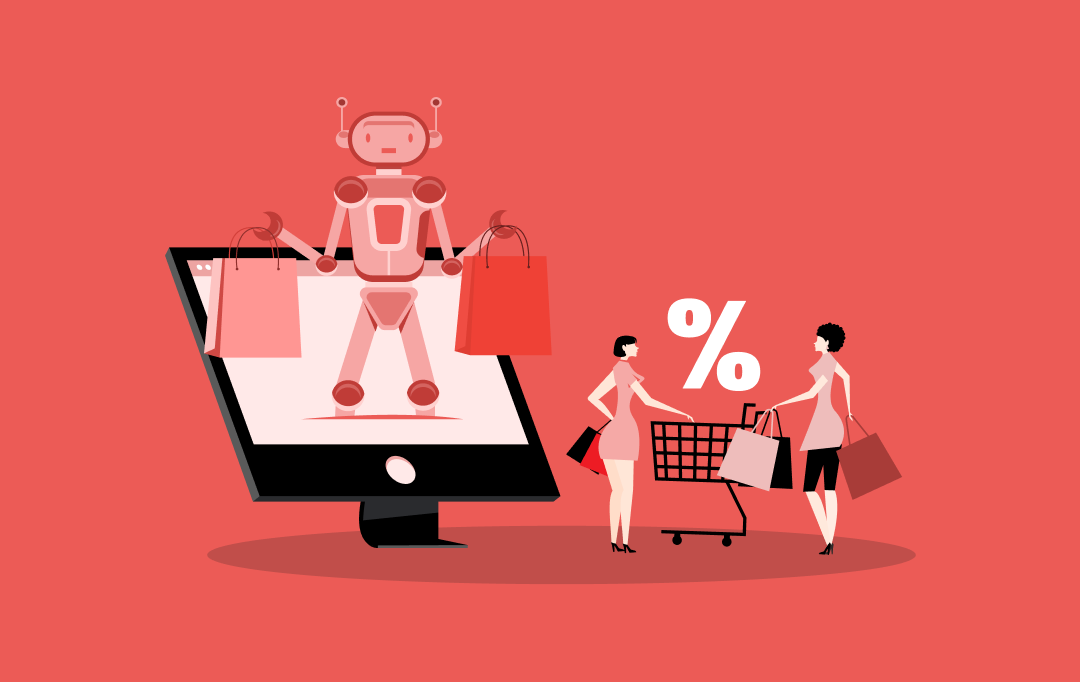
10 Use Cases and Benefits of How AI Agents Are Revolutionizing the Retail Industry
Key takeaways: AI agents in the retail industry are revolutionizing businesses by enhancing customer experience and streamlining operations. From personalized recommendations to predictive analytics, AI offers tangible benefits like increased conversions and reduced costs. AI adoption can lead to scalable growth and a competitive edge in the retail industry. Retailers must consider data strategies, talent…