- What is big data?
- How is big data analytics for manufacturing generated?
- Transformative Role of Big Data Analysis for Manufacturing: Key Applications and Advantages
- 1. Greater competitive edge
- 2. Less downtime
- 3. Greater CX
- 4. Supply chain management
- 5. Production management
- 6. Agile response to fluctuation in market demand
- 7. Speeding up the assembly
- 8. Identification of hidden risk in process
- 9. Product customization made feasible
- 10. Improvement of yield and throughput
- 11. Price optimization
- 12. Image recognition
- What are some of the top real-world big data in manufacturing use cases?
- How to incorporate big data in the manufacturing space?
- 1. Establish the business KPIs
- 2. Analyze the issues in manufacturing
- 3. Perform a cost-benefit analysis of the project
- 4. Incorporate big data in the manufacturing process
- Future of Big Data Analytics in the Manufacturing Industry
- Final thoughts
- FAQs
In the last 20 years, manufacturers – through the implementation of Six Sigma and Lean approaches – have been able to lower waste in the production processes and have improved the product yield and quality. But the volatile nature of the present day manufacturing segment, specially around chemicals, pharmaceuticals, and mining, has led to the need of having a more granular approach for identifying and correcting the flaws in the process.
Big data in manufacturing is one such solution. And there are signs that validate it, one being that the global big data in manufacturing domain is projected to reach $9.11 billion by 2026. The use case of the technology has been playing a big role in shaping this market growth. In this article, we are going to explore the role of big data analytics in manufacturing and how it is making the entire domain smarter and more efficient.
What is big data?
The technology can be defined as high velocity, high volume, and high variety data sets that help with processing information which enhances insights, helps with decision making, and automates processes.
Another way to define big data can be that it is an Industry 5.0 technology which consists of a diverse and complex set of data which is gathered through multiple resources and requires an advanced processing approach like cloud computing or machine learning to provide key business insights.
The technology is majorly made of three key elements –
Variety – There is a wide range of data available to businesses but they can be categorized into unstructured, semi-structured, and structured data.
Velocity – It refers to the rate at which the data gets received. Usually, the data is stored in memory but there are real-time processing mechanisms active in businesses as well.
Volume – The technology processes a big amount of information which comprises a range of structured, semi-structured, and unstructured data.
Now that we have looked at what big data stands for, it is time to look into how the data is generated by the manufacturing industry.
How is big data analytics for manufacturing generated?
In addition to the general sources of generating data like loyalty programs, online marketing analysis, and social media monitoring, the industry is using a range of software to collect the information.
The software sets ranging from CRP, MES, and CMMS, etc. are integrated with the machines to generate big data in the manufacturing space.
The data sets which these software and machines generate then can be used to form patterns, identify the problem areas, and come up with data-backed solutions.
Now to generate this massive amount of data, the industry requires a robust set of intuitive technology stack. At Appinventiv, we make use of some of the best in class industrial data analysis tools:
Transformative Role of Big Data Analysis for Manufacturing: Key Applications and Advantages
The benefits of big data in manufacturing ranges from several preventive level advantages to aiding predictive decisions. Let us look into the different ways that highlight the importance of data analytics in the manufacturing industry.
1. Greater competitive edge
The manufacturing industry has been the center of technological innovations. Whether it is mobile connectivity, industrial IoT or next-gen hardware, the data that is generated through all the different mediums helps raise competitiveness to the next level. The data leads to greater insights in the market trends, better understanding of the customer needs, and forecasts into the future trends. In short, it provides everything that gives manufacturing houses a massive competitive edge.
2. Less downtime
Downtime of hardware can be a real productivity hazard in the manufacturing domain. It doesn’t just hamper employees’ time but also requires a lot of maintenance and troubleshooting. Now, the solution that the industry has found for the issue is using industrial data analysis to perform preventive and predictive maintenance on their hardware. It helps the manufacturers keep a track of hardware’s quality assessment by analyzing their efficiency and working on a daily basis.
3. Greater CX
Manufacturing houses are now using advanced sensors to provide big-data powered alerts to the field technicians concerning the maintenance requirements, they are using RFID tags to monitor the condition of units, and making use of data-driven reports that offer accurate suggestions for improving the customer services.
4. Supply chain management
Big data analytics in manufacturing gives manufacturers the ability to track down the location of the products. This capability of tracking down the product location using technologies like radio frequency transmission devices, and barcode scanners, solves the issue of products getting lost or becoming difficult to trace. What this means for customers is that businesses are able to give them a more realistic delivery timeline.
5. Production management
One of the key productivity signs of a manufacturing house is determining what the market needs are and what volume of goods they need to create.
Back in the day when big data in manufacturing did not exist, businesses relied on human estimates that led to goods either getting produced in excess or shortage. Big data helps with giving businesses important predictive insights that helps them make the choice better.
Also read: Big Data Analytics in the Oil and Gas Industry – Benefits, Use Cases, Examples, Challenges
6. Agile response to fluctuation in market demand
The incorporation of real time manufacturing analytics specifically in the CRM system can help manufacturing houses forecast the future in real-time. The analysis of CRM data can showcase the difference in the order and consumption patterns that can be used for driving the adjustment in production. Moreover, the big data-driven intelligence gathered from the CRM can help with knowing what the customers are asking for and then preparing the production in a cycle in a way that the time to respond minimizes.
7. Speeding up the assembly
With big data analytics in manufacturing, businesses have gotten the capabilities of segmenting their production and identifying the units that get manufactured faster. This helps the manufacturing houses know where they have to focus their efforts to get maximum production. It would also help them identify the areas they are most efficient in, along with the ones they need to work on.
8. Identification of hidden risk in process
The analysis of data around the equipments’ past failures enables the manufacturers to forecast its lifecycle and set up the correct predictive maintenance schedules, which are either usage based or time based. All this, in turn, helps detect the gaps, lower the wastage and downtime, and help businesses create a recovery plan in case an unexpected failure occurs.
Moreover, big data when combined with AI enables the manufacturers to automate the processes so that they self-optimize without the involvement of a human intervention.
Also Read : How AI in Manufacturing is Revolutionizing the Industry: Key Use Cases and Examples
9. Product customization made feasible
Historically, manufacturing units have focused on producing at scale and left the customization to enterprises serving the concentrated market. Data analysis for manufacturing makes customization possible at the manufacturing stage by predicting its demand and then giving the manufacturers lead time to produce customized products at a great scale.
Using big data, manufacturers are able to streamline their manufacturing process by eliminating wastage and predicting the demand. This streamlining helps them with time they require to do mass personalization of the products.
10. Improvement of yield and throughput
Big data technology helps manufacturers find hidden patterns in the processes, enabling them to pursue their continuous improvement initiatives with better certainty. The result of this can be seen in a rise in the throughput and yield.
11. Price optimization
The price point of a product can be decided with the help of big data. The technology can collect and analyze the data from multiple stakeholders like customers, suppliers, etc. to determine the best price point that suits both customers and businesses.
12. Image recognition
A manufacturing house can find a range of image recognition specific use cases for big data. Let’s see an example. Suppose you require a specific spare part but you don’t know what it’s called or how much it costs. A big data powered image recognition software can help businesses capture the image and give the details back to the manufacturers.
Now that we have looked into the wide set of reasons as to why big data is important in manufacturing, let us look at some real-world cases of where businesses have adopted the technology for an evident rise in production efficiency.
What are some of the top real-world big data in manufacturing use cases?
The manufacturing industry has made it evident that there are a number of benefits that big data offers to the domain. But how are those benefits actually being leveraged in the real world? Let us find out through some real-world examples of companies.
Company | Outcome of Using Big Data |
---|---|
Colfax | – Detection of anomalies and patterns in applications – Increase in asset utilization |
National Engineering Industries Limited (NEI) | – Increase in visibility around shop floor, line, plant, and the enterprise performance – Avoidance of unplanned breakdown through proactive actions |
Kia Motors | – Forecast of maintenance costs and failure rates – Reduction of production time – Categorization and extraction of complaints from customer surveys to uncover the quality issues |
Siemens Healthineers | – Prediction of product failure – 36% less system downtime |
Deutsche Bahn | – Reduction of 25% in maintenance costs – Reduction in delay-causing failures |
Now that we have looked into the real-world use cases of big data in the manufacturing domain, let us look into the ways the technology can be adopted in the industry.
How to incorporate big data in the manufacturing space?
While every project is different, there are some steps which are common in every project that calls for the adoption of big data in manufacturing.
1. Establish the business KPIs
The beginning of a big data project should start with knowing what is expected from its inclusion. You will only be able to validate the profit and feasibility of the technology in your manufacturing business when you know the key performance indicators to measure them against.
2. Analyze the issues in manufacturing
The next step would be getting details around your current manufacturing requirements and needs. Only when you know how your manufacturing unit is performing today, will you be able to find scope for big data inclusion. An analysis of your current status will also help you build a strong quality improvement process.
3. Perform a cost-benefit analysis of the project
Once you have set the KPIs for the technology and analyzed the issues in business, the next step would be to know the cost of the project. When estimating this price point, account for all development, integration, and maintenance of the project. Once done, measure this cost against the possible perks that the manufacturing unit can expect.
4. Incorporate big data in the manufacturing process
Once you have identified the processes which you will be incorporating big data in and have analyzed the cost, benefit analysis, the next step would be partnering with a reliable big data company. They will help you with a seamless integration of the technology in the manufacturing houses.
Future of Big Data Analytics in the Manufacturing Industry
The future of Big Data Analytics in the manufacturing industry promises significant advancements, reshaping how operations are managed and optimized. As technology advances and data grows more extensive in the digital landscape, we can anticipate the emergence of Big data-powered applications and their continued evolution. Here are some projected advancements to be seen in the coming years, spanning the full range of Big Data applications in manufacturing:
- AI-powered analytics will drive more intelligent decision-making, enabling manufacturers to optimize processes and predict outcomes with greater accuracy.
- The integration of Big Data across all stages of manufacturing will streamline workflows and drive operational excellence.
- Digital twins will become increasingly prevalent, offering real-time simulations that mirror physical production environments, enhancing efficiency and innovation.
- Closed-loop manufacturing systems will facilitate continuous self-optimization, allowing processes to automatically adjust and improve based on real-time data feedback.
- The integration of Big Data across all stages of manufacturing will streamline workflows and drive operational excellence, while a strong emphasis on sustainability will utilize data to minimize waste and reduce energy consumption.
- Additionally, the manufacturing workforce will evolve, with a growing demand for data-savvy workforce, skilled in leveraging these advanced technologies to maintain a competitive edge.
Final thoughts
Big data in manufacturing, as highlighted throughout this article, plays a pivotal role in boosting production efficiency, predicting anomalies more accurately, and securing a competitive advantage. However, simply applying it within traditional systems can be challenging and often inadequate. For optimal results, it is essential to avail Big Data integration services with technologies like IoT and AI. This approach enhances system capabilities and fosters innovation in manufacturing processes.
What manufacturers truly need to gain advantage from the technology is the support of a data analytics service powerhouse like Appinventiv. If you are looking to modernize your manufacturing house, reach out to us.
FAQs
Q. What are the challenges of implementing Big Data in manufacturing?
A. Implementing Big Data in manufacturing presents several challenges, including the integration of diverse data sources across various systems, ensuring data quality and consistency, and managing cybersecurity risks associated with large-scale data handling. Additionally, there is a critical need for skilled data analysts and developers to leverage the data effectively. The initial investment in Big Data infrastructure and analytical tools can also be substantial.
However, these challenges can be addressed through phased implementation. If you are unsure how to address these challenges effectively, contact a reputed big data service provider now.
Q. What is Big Data analytics in manufacturing?
A. Big Data analytics in manufacturing is about using advanced analytical tools and techniques to process and analyze large volumes of data generated from production lines, equipment, and supply chains. This ‘big data analytics for manufacturing helps businesses gain valuable insights into operational efficiency, predict maintenance needs, optimize processes, and drive innovation in product development.
Q. What are the applications of Big Data in the manufacturing industry?
A. Big Data analytics for manufacturing has various use cases. Here are some of the most common applications of big data in the manufacturing industry:
- Supply chain management
- Production management
- Agile response to fluctuation in market demand
- Speeding up the assembly
- Identification of hidden risks in the process
- Product customization made feasible
- Improvement of yield and throughput
- Price optimization
- Image recognition and so on
To gain an in-depth understanding of big data in the manufacturing industry, please refer to the above blog.
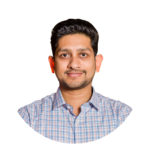
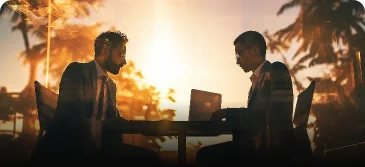
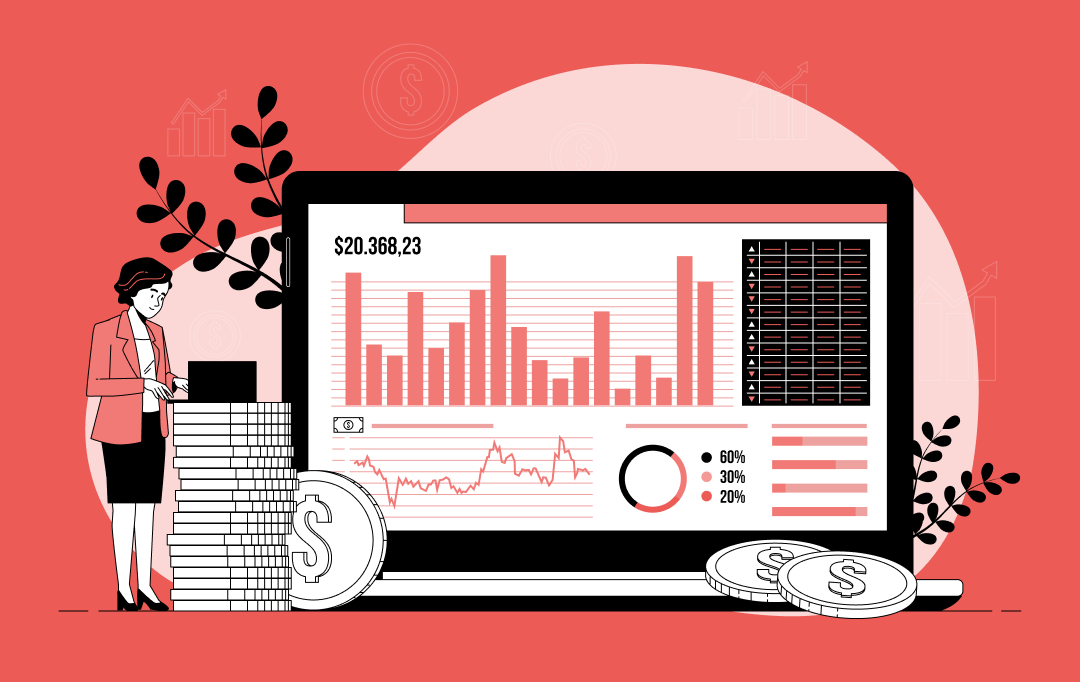
Unleashing the Role of Data Analytics in Driving Smarter Banking Decisions
The use of data analytics in banking is transforming how this data is interpreted and applied. Banks are using this data to make decisions that matter—fraud checks, loan approvals, or marketing campaigns. The focus has shifted: from storing data to using it smartly, and from pushing products to understanding people—being customer-centric. This shift is also…
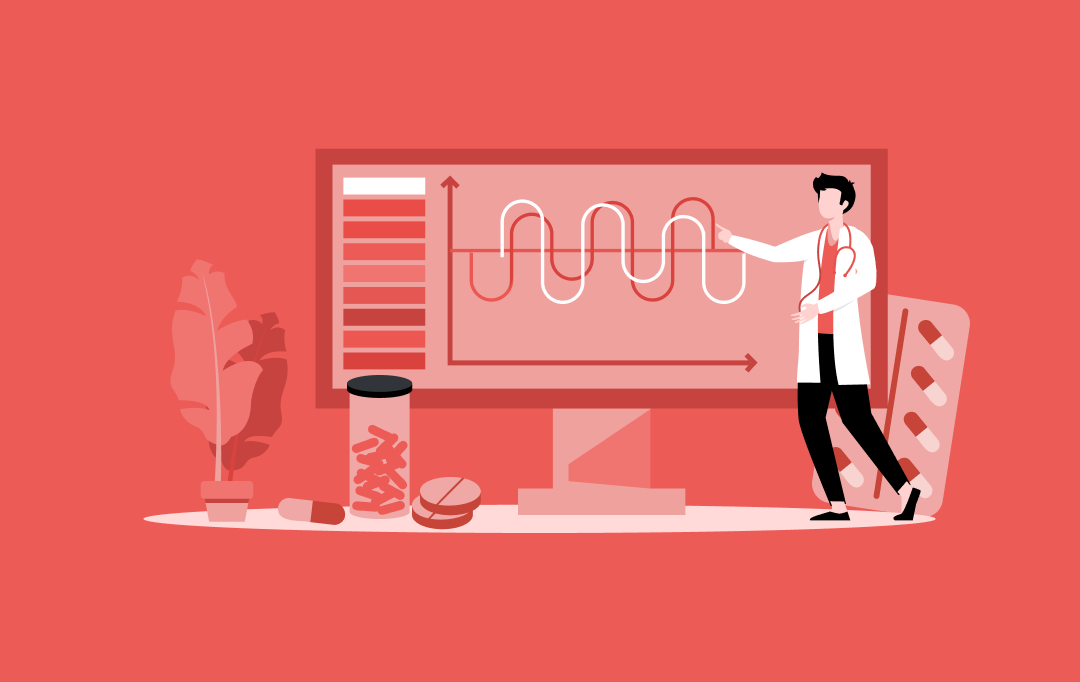
10 Use Cases and Examples of How Pharmacy Data Analytics is Transforming Operations and CX
Data has become the currency of modern business, and the pharmacy sector is no exception. From inventory optimization to regulatory compliance, pharmacies today face many challenges that demand precision and agility. Well, pharma data analytics is solving all these conundrums as the main lead, a transformative approach that now empowers businesses to get actionable insights…
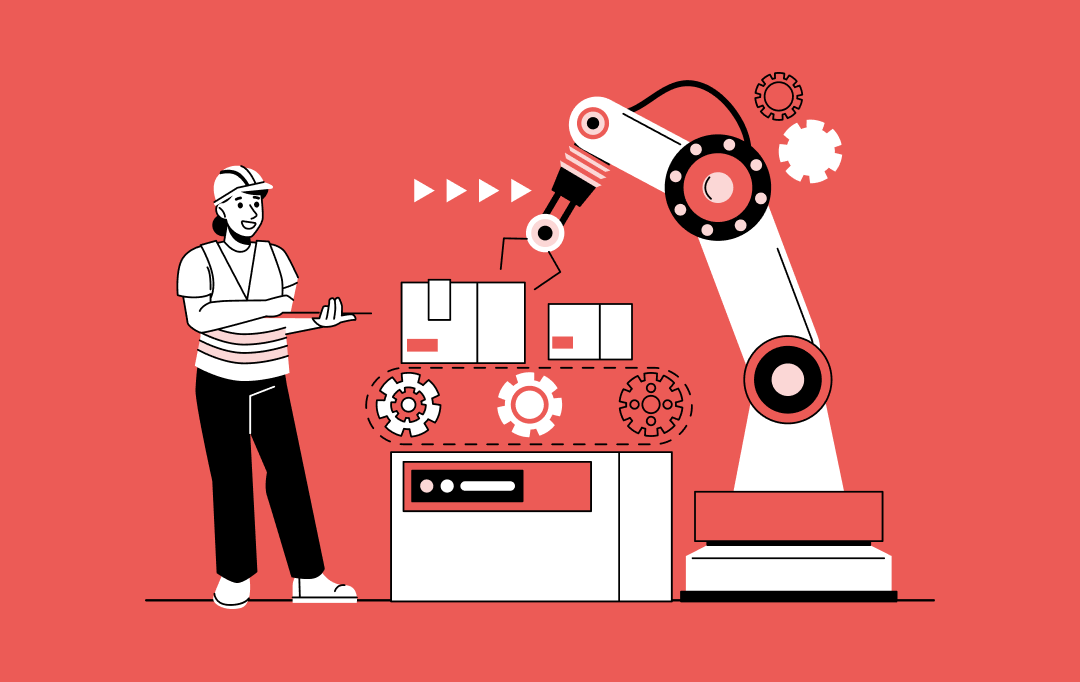
10 Use Cases and Benefits of Predictive Analytics in Manufacturing
Are you trying to stay in today’s manufacturing game with that old-school data analytics approach? Not gonna happen, buddy! Think about that old-school grind: manufacturing plant techies had to hustle around, scribbling notes and logging maintenance records by hand. All that manual intervention and hassle led to nothing more than messy data and bad calls.…