- How is an AI Project Different from Traditional Projects?
- Splitting AI Into Two Distinct Categories
- Existing Artificial Intelligence Solutions
- Custom Artificial Intelligence Solutions
- A Slight Detour: Understanding the Pillars of AI Project Success
- The Challenges of AI Project Development: Why AI Projects Fail
- Misaligned Expectations
- Inefficient Data Management
- Answering the Question of the Hour: How to Manage Your AI Projects
- 1. Identifying Problem
- 2. Testing the Problem Solution Fit
- 3. Preparing and Managing Data
- 4. Choosing the Right Algorithm
- 5. Training the Algorithms
- 6. Deployment of the Project
- FAQs About the Steps of AI Project Management
- Q. How To Get Started with Artificial Intelligence And Machine Learning
- Q. What is a good idea for an artificial intelligence project?
- Q. Are AI projects better than traditional IT projects?
The question of whether or not do AI enables companies to streamline their processes and help them in delivering proactive solutions has been answered and dusted by the digital world.
There is hardly any industry operative in the world today that is oblivious to the high revenue and value offering potential that artificial intelligence comes packed with. A declarative fact that is evident from the promising AI technology trends for 2020 and later.
This quick adoption, while on one hand has come with a lot of benefits for both businesses and end-users, it is on the other hand is on a very native stage. Meaning, businesses are yet to find concrete use cases and return effectiveness. This nascency and benefit combination has given birth to a number of queries around how to manage your AI projects.
Seeing how complexity lies at the very center of the AI project management solutions, it is important to understand the intricacies of managing AI projects.
In this article, we answer every question and element surrounding how we, at Appinventiv, perform AI project management and the steps we follow to successfully transform a Proof of Value (POV) to efficient generative AI solutions & services.
Table Of Content
- How is an AI Project Different from Traditional Projects?
- Splitting AI Into Two Distinct Categories
- A Slight Detour: Understanding the Pillars of AI Project Success
- The Challenges of AI Project Development: Why AI Projects Fail
- Answering the Question of the Hour: How to Manage Your AI Projects
- FAQs About the Steps of AI Project Management
How is an AI Project Different from Traditional Projects?
AI project management calls for a different approach when parallels are drawn between them and traditional mobile app project management. Meaning, the differences between AI projects and traditional IT projects are manifold.
The traditional mobile app development process is a solution specified. Whenever it gets difficult to specify a solution, the results become uncertain and risky. This development type falls under the top-down programming.
Opposedly, in case of AI projects’ Proof of Value (POV), a bottom-up approach is followed. In that case, AI draws conclusions from its own rules and processes of working with an extensive data set.
The AI development landscape also tends to open up several opportunities as the cycle matures. Meaning, for a project to be deemed complete, it has to cross several stages of exploration and hits and trials. While the outcome of the approach is almost always high revenue friendly, it often leads to high development cost and extended development timelines.
The last part of the question surrounding how to manage your AI projects lies in making change management an integral part of the Agile process. The principle that AI program managers generally work on is fail-fast, wherein the idea is to explore expeditiously and fail right at the beginning of a wrong approach, instead of at a later stage in the development process.
Splitting AI Into Two Distinct Categories
The first part of planning your AI project starts with our team understanding the category it belongs to. Category one deals with projects that are common in nature, like translating a language in another, or converting images into words. Category two is more complex. It handles tasks like detecting heartbeat or monitoring sleep.
The two categories call for two distinct solutions – incorporation of an existing AI or creating custom AI project management solutions.
Existing Artificial Intelligence Solutions
There are a number of events where the inclusion of AI has become common and mainstream. Meaning, there are ready-made tools that our engineers only have to integrate AI into the applications. A few of the platforms that our team generally use include Microsoft Azure AI, Google AI Platform, and Amazon Machine Learning, etc.
Custom Artificial Intelligence Solutions
In case there is a complex project in hand, like recently we made a neural network driven healthcare app that gave users insights into their health on the basis of their voice, we have to resort to custom AI solution development. To make the process easier, Android 11 will be using its new Neural Networks API 1.3, as an effort to make your Machine Learning apps run smoothly on devices.
A Slight Detour: Understanding the Pillars of AI Project Success
Our journey with Artificial Intelligence started back in 2019. It took us an extended delivery timeline to understand that the secret to an AI project success lies in two pillars – people and data. Only in the presence of the two pillars, AI is able to improve customer experience to its entirety.
We started with bringing in experts from different sections that the application adhered to – irrespective of whether or not they had a technical expertise. It was necessary to feed in domain specific data into the algorithm to make the AI system efficient and unbiased.
The next part – the second pillar – was data. Data, when not rightly stored or when not in its entirety is utterly useless. Now, there are two types of data that a business ejects – structured (ones like date of birth, address, etc.) and unstructured data (invoices, voice recordings, emails, etc.). When in the process of AI project management, you have to consider both the data types.
There are certain steps that a data has to go through to become one that can be used for deep learning or Artificial Intelligence. Ones that our team of data engineers work on when we develop Artificial Intelligence (AI) solutions for scale-ups and enterprise clients.
The faster data finds a place in this pyramid, which is based on Maslow’s Hierarchy needs, the faster your AI project will start churning and the greater would be the possibility of engineers working on modeling instead of keeping their focus on data filtration.
The result of our exploration journey was an understanding of the different issues that come across when answering what creates a valuable A.I solution. Let us counter those issues before walking you through the stages of managing AI projects in a way that their Proof of Value (POV) reflects into the end system.
The Challenges of AI Project Development: Why AI Projects Fail
If we sit down to make a list of what challenges do companies face when implementing AI, the list will be very extensive. But at the core of it all of why Proof of Values fail, lies two prime causes – misaligned expectations and insufficient data management capabilities. The causes that hold back businesses from making money in AI.
Misaligned Expectations
More often than not, the majority of AI projects don’t see the light of the day because of the attached misalignment in expectations. The root cause of challenges of artificial intelligence in business often emerges because of heightened short term expectations from a technology which inherently operates on a long-term mode.
Next instance of misaligned expectations can be seen in businesses assuming their AI based solution will be accurate enough to meet different user perceptions. For example, in case of a music streaming application, assuming that the “next song” your AI is suggesting is exactly what the user believes to belong to the genre is a problem area. This is the reason why businesses often use the word ‘may’ when showcasing products or services that their users might be interested in, next.
Inefficient Data Management
AI tends to make wrong decisions on the basis of wrong datasets. The problem in AI project management solutions emerge when the data is incorrect or incomplete – in short, not prepared to fit into the AI model.
For an AI system to work as expected, it is necessary to have refined data which the system can use to learn and analyze patterns. When we build an AI-ready data set our focus is primarily on dividing the structured and unstructured information following the modern data collection strategy.
Answering the Question of the Hour: How to Manage Your AI Projects
1. Identifying Problem
The first step for us when it comes to managing AI projects is identifying the problem. We start with asking our partners two questions: “what is it you are willing to solve?” and “what is the desired outcome for you?”
When settling on a problem statement it is important to understand that AI in itself is not a solution but a means/tool to meet the need. Noting that, we choose multiple solutions, which can be built upon with the help of AI and not be dependent on it.
2. Testing the Problem Solution Fit
This stage, ideally answers how to start an AI project. Before we initiate the AI project development process, it is first important to test and be sure that people are willing to pay for what you are building.
We test the problem-solution fit through a number of techniques like traditional lean approach and Product Design Sprint.
One of the best things about AI technology is that it is very fairly easy to create a base level version of a solution by using real humans or MVP. The benefit of this is not just easy analysis of a solution but also within time guarantee that the product actually needs an AI solution.
3. Preparing and Managing Data
Having reached the point where we know that there exists a customer base for your solution and you have the confidence that the AI can be built, we initiate managing machine learning projects by gathering data and handling their management.
We start by dividing the available data in structured and unstructured forms. Although the stage is fairly easy when we are working with a startup or a company that doesn’t have multiple data, building multiple applied AI solutions for enterprise is what is tricky. Generally, big firms have huge proprietary database data which might be ready for AI and what could make it all the more difficult is the fact that the data might be stored in silos.
Our data engineers start with organizing and cleaning up the data, where in principle, they define a chronological order and add labels where needed.
4. Choosing the Right Algorithm
Although, to keep the essence of the article, we won’t mention the technicalities of AI algorithms here, but what is important to know is that there are different types of algorithms, which vary on the basis of the learning you do.
- Supervised Learning
At its core, classification predicts a label and regression predicts the quantity. We generally choose classification algorithms when we want to understand the chances of an event occurrence, e.g. the chance of rainfall tomorrow.
On the other hand, we go with regression algorithms when we have to quantify the scenario, e.g. when we want to know the chance of an area drowning.
There are several other algorithms that our engineers choose from depending on the project requirement – naïve Bayes classification, random forest, logistics regression, and support vector machine.
- Unsupervised Learning
The choice of algorithm would be very different here since the data is not organized or follows a certain type. We might use clustering algorithms for grouping objects together or association algorithms when finding links between different objects, etc.
5. Training the Algorithms
Once we have selected the algorithm we move on to training the model wherein we input data into the model, keeping the importance of model accuracy into consideration.
Our team of engineers understand that setting the minimum acceptable threshold and applying statistical discipline are the key steps to accelerate the development of AI, in a way that it calls for minimal fine-tuning later.
For training the algorithms and taking the next developmental steps, we employ tech experts who are experts in Python, R, Java, and C++. Depending on the project needs, we also involve experts who understand Julia – the top language for machine learning app development.
6. Deployment of the Project
We generally advise our partners to go with ready-made platforms like Machine Learning as a Service for their product launch and deployment needs. These platforms are developed to simplify and facilitate Artificial Intelligence and aid the deployment phase of an AI project. They also provide cloud-based advanced analytics that can be used to add in different languages and algorithms.
[Also Read: Consider Important Steps to Write a Masterful project Plan]
FAQs About the Steps of AI Project Management
Q. How To Get Started with Artificial Intelligence And Machine Learning
There are six steps that are covered in the process of AI project management: Identification of the problem, testing the problem solution fit, data management, selecting the right algorithm, training the algorithm, and deploying the product on the right platform.
Q. What is a good idea for an artificial intelligence project?
AI has gotten a scope across a number of industries. What is necessary is to find a use case that incorporates the technology in a way that generated data is organized and converted into actionable analysis. It is important to be realistic about your expectations from the AI solutions in terms of treating it as a tool helping in the advancement of your service, instead of it becoming a service itself.
Q. Are AI projects better than traditional IT projects?
It depends from situation to situation. There are indeed some projects that do better with AI inclusion, while there are other applications that become unnecessarily complex with the technology’s integration. Ultimately, it depends on the use case and how valued it would become with artificial intelligence.
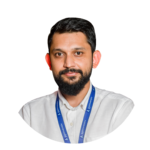
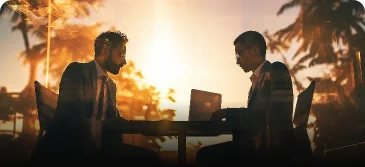
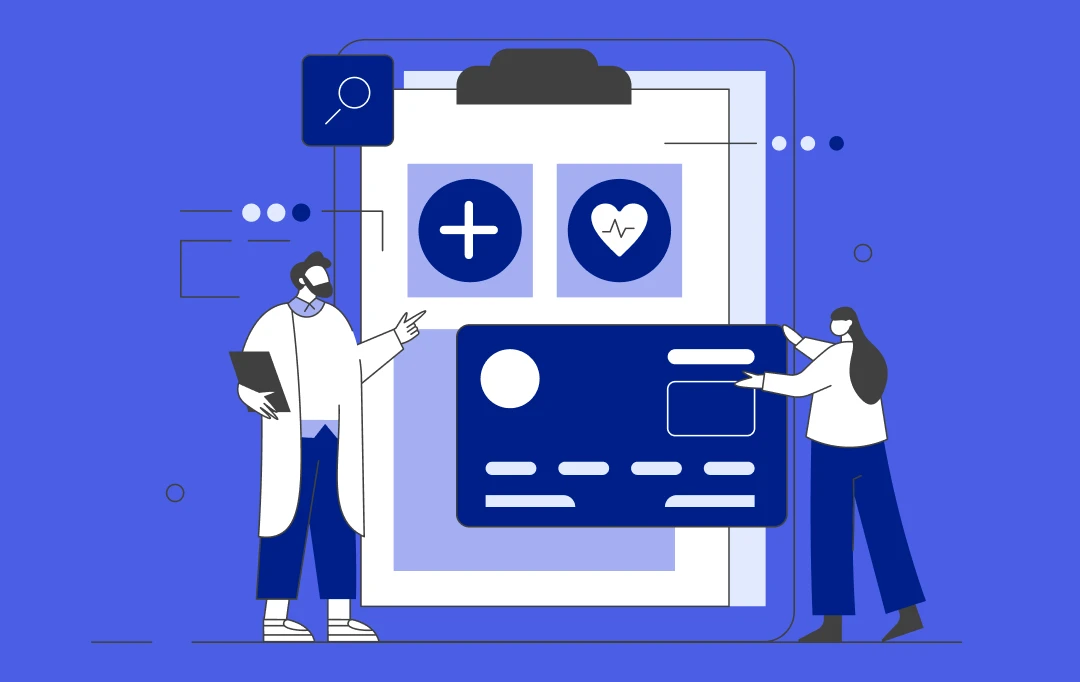
10 Ways AI is Transforming the Healthcare Sector in Australia
Key takeaways: Generative AI could add $13 billion annually to Australia’s healthcare sector by 2030. AI is already transforming operations across diagnostics, admin tasks, and patient care. Healthcare in remote areas is improving with AI-powered virtual assistants and monitoring tools. AI can help address workforce shortages by automating routine tasks and supporting clinical decisions. Major…
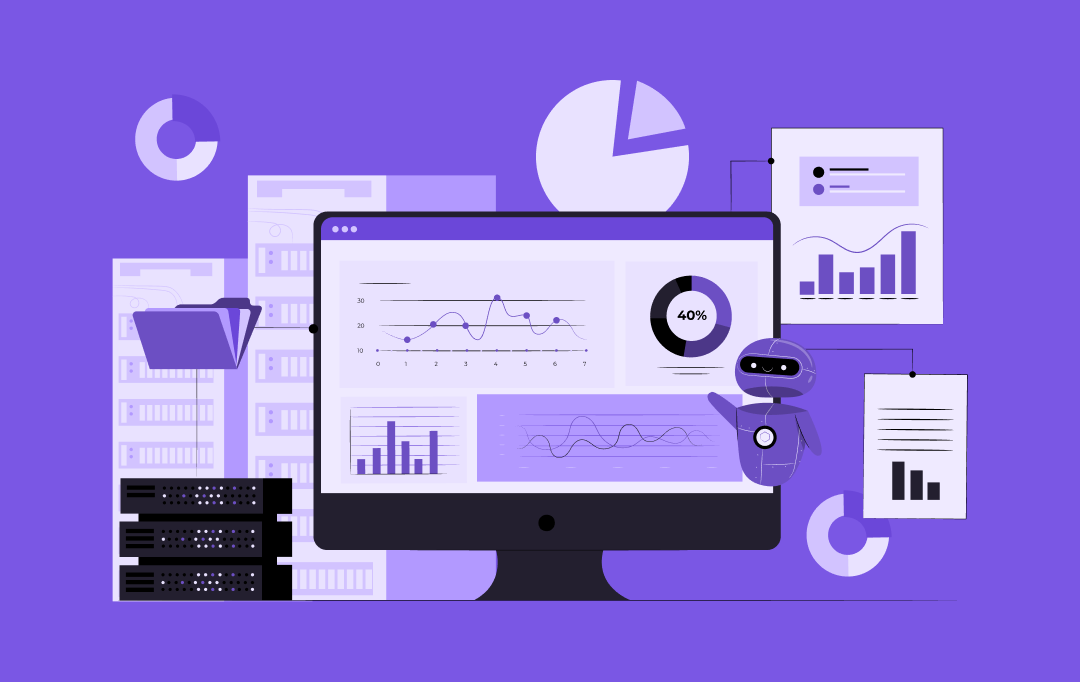
Exploring the Power of AI in Creating Dynamic & Interactive Data Visualizations
Key takeaways: AI transforms data visualization by providing automated insights, interactive dashboards, and NLP, thereby tackling revenue loss and improving data quality. Key features include predictive analytics, real-time data integration, and user-friendly visualizations, enabling faster and more informed decisions. Top 2025 tools, such as Tableau, Power BI, and Julius AI, offer NLP and real-time analytics…
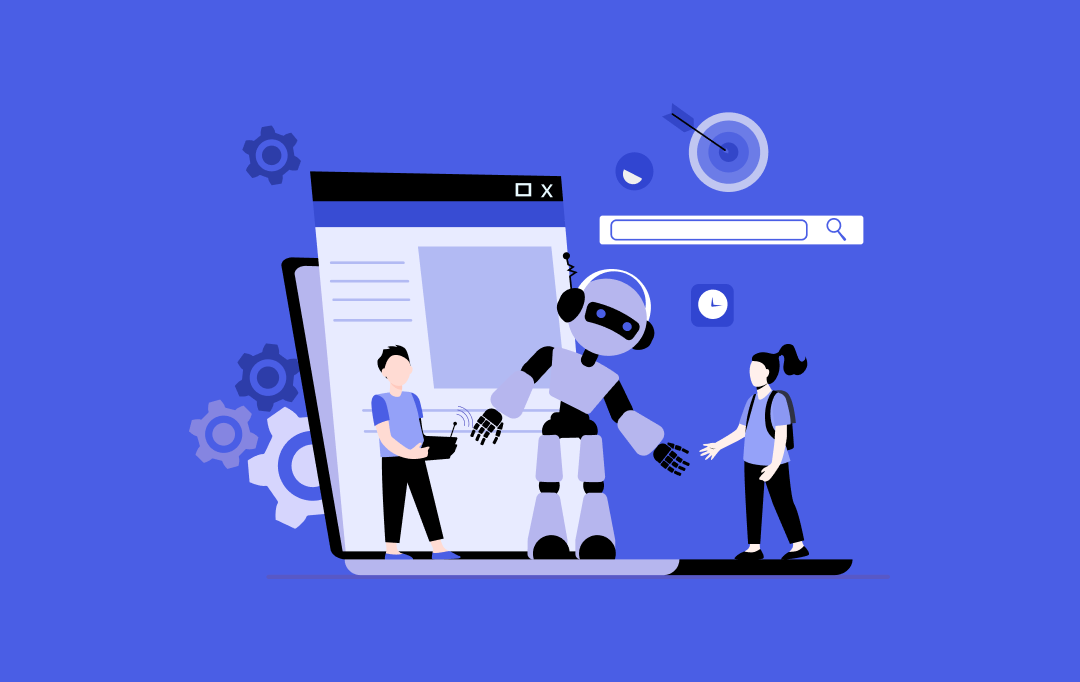
Practical Applications of AI as a Service for Your Business
Key takeaways: AIaaS enables fast, infrastructure-free AI adoption. Used across industries for automation and insights. Real-world examples show proven business impact. Pilot-first approach ensures smooth implementation. Key challenges include data, cost, and scaling. Trends include ethical AI, edge, and SME growth Imagine being able to cut down your operational costs while simultaneously improving decision-making and…