- The Most Prevalent Credit Card Fraud Techniques
- Card Skimming and Cloning
- Phishing and Social Engineering
- Card-Not-Present (CNP) Fraud
- Account Takeover (ATO) Fraud
- Identity Theft and Application Fraud
- Card Cracking
- Synthetic Identity Fraud
- Traditional Credit Card Fraud Detection vs. Machine Learning
- Traditional Credit Card Fraud Detection
- Machine Learning in Fraud Detection
- The Paradigm Shift
- Real-World Use Cases of Credit Card Fraud Detection System Using Machine Learning
- PayPal
- Visa
- Amazon
- American Express
- JPMorgan Chase
- Mastercard
- Stripe
- Alibaba
- How to Integrate ML for Credit Card Fraud Prevention in Your Business Model
- Data Collection and Integration
- Choose the Right ML Algorithms
- Data Preprocessing and Feature Engineering
- Real-Time Monitoring and Transaction Scoring
- Implement Continuous Learning
- Actionable Insights and Decision-Making
- Scalability and Flexibility
- Integration with Other Security Systems
- Credit Card Fraud Detection Challenges
- Data Quality and Availability
- Imbalanced Datasets
- Real-Time Processing Requirements
- Evolving Fraud Patterns
- Interpretability of ML Models
- Resource and Computational Costs
- Advantages of Machine Learning in Credit Card Fraud Prevention
- Real-Time Fraud Detection
- Enhanced Accuracy and Precision
- Adaptability to Evolving Fraud Tactics
- Scalability for High-Volume Transactions
- Proactive Fraud Prevention
- Improved Operational Efficiency
- Cost Savings
- Enhanced Customer Trust and Retention
- Appinventiv: Leverage ML Capabilities to Protect Millions of Daily Transactions
- FAQs
The rise of digital transactions has revolutionized commerce, offering speed, convenience, and global reach. However, it has also opened the floodgates to sophisticated fraud schemes.
Credit card fraud now ranks as one of the most pervasive threats in financial ecosystems, costing businesses and consumers billions annually U.S. credit card debt reached $1.14 trillion, emphasizing the critical need for robust fraud detection systems to safeguard financial transactions. For organizations, the stakes are immense – not just in financial losses but also in customer trust and regulatory compliance.
What makes this challenge so pressing is the evolving nature of fraud itself. Traditional rule-based systems, once sufficient to flag suspicious activity, are struggling to keep pace with attackers who employ advanced tactics to evade detection. This leaves enterprises searching for a robust, scalable solution that adapts in real-time.
Financial fraud detection using machine learning is reshaping fraud detection in credit card. By analyzing vast amounts of data at unprecedented speed and accuracy, ML algorithms can uncover patterns, and anomalies, and even predict fraudulent behavior before it occurs.
But how exactly does this technology work, and why should your organization prioritize its adoption?
This article uses machine learning to explore the transformative role of credit card fraud detection, highlighting its methodologies, benefits, and real-world impact. Whether navigating a C-suite strategy session or fine-tuning risk management processes, understanding this innovation is crucial to safeguarding your operations in today’s digital-first landscape.
Wanna lock down your financial ops with a rock-solid fraud detection system? Holler at our Fintech Software Dev Experts today and map out your security game plan.
The Most Prevalent Credit Card Fraud Techniques
Credit card transaction fraud detection has become increasingly sophisticated, with criminals leveraging traditional and advanced methods to exploit vulnerabilities in payment systems. Understanding these techniques is key to implementing effective defenses.
Card Skimming and Cloning
Fraudsters use skimming devices to secretly capture credit card information from the magnetic stripe during legitimate transactions. These devices are often installed on ATMs, gas station pumps, and point-of-sale terminals.
Once the card data is retrieved, it is cloned onto counterfeit cards, enabling unauthorized transactions. Modern skimming devices are difficult to detect; some use wireless technology to transmit stolen data in real-time.
Phishing and Social Engineering
In phishing schemes, attackers pose as trusted entities – such as banks or retailers – via emails, text messages, or phone calls to trick individuals into sharing sensitive information. This might include credit card details, passwords, or answers to security questions.
These attacks often exploit human emotions like fear or urgency, creating convincing scenarios to bypass skepticism. What makes this a step ahead is social engineering, which goes a step further by manipulating victims in person or over the phone to gain access to personal and financial data – all of this creates an environment where having a tool for fraud detection in credit cards ultimately becomes a necessity.
Card-Not-Present (CNP) Fraud
CNP fraud involves using stolen credit card information to make purchases where the physical card is not required, such as online or over the phone. Fraudsters exploit the anonymity of digital transactions, often testing stolen card details on low-value purchases to verify their validity.
The growing volume of e-commerce has made CNP fraud one of the most prevalent and challenging types of fraud to detect, as traditional security measures are often inadequate, thus calling for more stringent credit card fraud detection system development efforts.
Account Takeover (ATO) Fraud
Account takeover occurs when fraudsters gain unauthorized access to a cardholder’s online accounts, often through compromised credentials obtained via data breaches or weak passwords.
Once inside, they can change account details, order replacement cards, or make unauthorized transactions. Since the activity often mimics that of the legitimate account holder, ATO fraud can go unnoticed for extended periods, increasing financial and reputational risks.
Identity Theft and Application Fraud
Criminals use stolen personal information – such as Social Security numbers, addresses, and birthdates, to open new credit card accounts in the victim’s name. This is known as application fraud. Victims may remain unaware until they receive bills for unfamiliar accounts or discover unexpected entries on their credit reports.
Identity theft can also lead to long-term damage, including poor credit ratings and legal complications, making it a particularly damaging form of fraud.
Card Cracking
Card cracking involves using automated tools to guess credit card details such as expiration dates and security codes. Fraudsters can ‘crack’ a card’s information without physical access by systematically testing combinations. This technique is often used with stolen or leaked partial card details, allowing criminals to commit fraud with greater precision.
Synthetic Identity Fraud
Synthetic identity fraud is a sophisticated scheme where criminals create fake identities by combining real and fictitious information. For example, they might use a legitimate Social Security number with a phony name and birthdate to apply for credit cards.
Since the synthetic identity does not correspond to a real person, it can take years for the fraud to be detected. This type of fraud is particularly challenging for financial institutions, as it circumvents traditional verification processes.
Each technique highlights the dynamic and adaptive nature of credit card fraud. As criminals continue to innovate, businesses must adopt proactive and advanced detection measures to protect their operations and customers from financial and reputational harm, especially when investing in credit card fraud detection software development.
Though foundational, traditional fraud detection methods often fall short in tackling modern fraud’s sophisticated and dynamic nature. This gap has paved the way for advanced technologies like machine learning, revolutionizing how financial institutions safeguard transactions.
In the following section, we’ll explore how traditional detection frameworks compare to machine learning-based approaches and why a shift toward smarter systems is imperative in the fight against credit card fraud.
Traditional Credit Card Fraud Detection vs. Machine Learning
The evolution of credit card fraud detection methodologies has transformed how financial institutions combat fraud. While traditional systems rely on predefined rules, fraud detection machine learning introduces adaptive, data-driven techniques that enhance detection and prevention capabilities.
Traditional Credit Card Fraud Detection
Traditional systems detecting credit card fraud operate on static, rule-based frameworks that flag transactions based on pre-established criteria. For instance, a transaction exceeding a set spending threshold or occurring in an unusual location might be flagged. These systems rely on human analysts to develop and refine rules based on observed fraud patterns.
While effectively detecting known fraud scenarios, these systems struggle with new or unconventional fraud tactics. Their rigid nature often results in high false positive rates, blocking legitimate transactions and creating customer friction. Moreover, as transaction volumes increase, maintaining and updating rules becomes increasingly time-intensive and less effective at addressing emerging fraud trends.
Machine Learning in Fraud Detection
Credit card fraud detection machine learning takes a radically different approach by leveraging machine learning algorithms to analyze vast datasets, uncover hidden patterns, and identify anomalies indicative of fraud. Instead of relying solely on human-defined rules, ML models learn from historical data to understand typical transaction behaviors. Over time, these models can adapt to new fraud techniques without manual intervention.
A key advantage of ML-based credit card fraud detection tools is their ability to evaluate numerous factors simultaneously. For example, an ML model can assess variables such as transaction location, time, device used, and past behavior patterns to determine the likelihood of fraud. This multidimensional analysis enables more precise detection while minimizing false positives and negatives.
Additionally, using machine learning, credit card fraud detection systems excel in real-time processing, making them well-suited for modern high-transaction environments. By continually updating with new data, they can avoid fraudsters who constantly refine their techniques to exploit system vulnerabilities.
The Paradigm Shift
The shift from traditional to credit card fraud detection using machine learning represents a fundamental change in approach.
Conventional methods, while simpler and easier to implement, are no longer sufficient to combat the increasingly sophisticated tactics of fraudsters. To support this, machine learning offers a more dynamic and scalable solution, empowering financial institutions to stay proactive rather than reactive in their fraud prevention efforts.
The shift from traditional to machine learning-based fraud detection isn’t merely a technological upgrade but a reimagining of how financial institutions approach fraud prevention. Conventional systems, while foundational, often struggle to keep pace with fraudsters’ evolving tactics. With its ability to adapt and predict, machine learning bridges this gap, offering dynamic and highly effective solutions in combating modern fraud.
As we delve deeper, it becomes evident that the benefits of using machine learning for credit card fraud detection go beyond improving detection accuracy. They encompass real-time adaptability, operational efficiency, and enhanced customer trust – key factors defining secure financial transactions’ future.
Let’s explore how ML sets new standards in credit card fraud detection and reshape how institutions safeguard their customers and assets.
Real-World Use Cases of Credit Card Fraud Detection System Using Machine Learning
Machine learning has been instrumental in transforming fraud detection for global payment providers, financial institutions, and e-commerce platforms. These real-world machine learning credit card fraud detection examples illustrate how industry leaders leverage ML to safeguard transactions, minimize fraud losses, and enhance customer trust.
PayPal
With over 200 million active accounts, PayPal invests heavily in anti-fraud technologies, allocating $300 million annually. Initially reliant on logistic regression models, the company gradually incorporated more advanced fraud detection in credit card techniques such as gradient-boosted trees (GBTs) and neural networks, leading to a 50% improvement in detection accuracy.
Recently, PayPal adopted deep learning models, which are 10-20% more accurate than traditional ML algorithms. These models benefit from PayPal’s extensive fraud data collected over years of operations. Today, PayPal’s fraud loss rate is among the lowest in the industry at 0.28% (28 cents per $100 processed).
Visa
Visa employs neural networks in its fraud detection system, Visa Advanced Authorization, which assesses over 500 transaction attributes, including type, location, spending patterns, and time of day. This multi-layered fraud detection machine learning system processes millions of transactions in milliseconds and sends fraud probability scores to banks for real-time decisions.
As a result, Visa prevents up to $25 billion in annual fraud losses and maintains an exceptionally low fraud rate of less than 0.06% (6 cents per $100 transacted).
Amazon
Amazon has leveraged its extensive experience combating online fraud to launch Amazon Fraud Detector, a fully managed ML-based service available to AWS customers. Businesses integrate their historical data with Amazon’s proprietary datasets to train fraud detection models tailored to their needs.
Early adopters, including Truevo and ActiveCampaign, have reported success in identifying and mitigating fraud risks. This service underscores how ML-powered tools can be democratized for businesses of all sizes.
American Express
American Express is another machine learning credit card fraud detection example where the brand processes $1.2 trillion in annual transactions and has the lowest fraud rates in the industry. The company began implementing machine learning models in 2014 and now integrates deep learning for enhanced fraud prevention.
For small businesses, AmEx offers Enhanced Authorization, a free ML-based tool that helps merchants reduce fraudulent transactions by 60%. American Express ensures superior consumer and business protection by monitoring transactions in real-time.
JPMorgan Chase
JPMorgan Chase, through Chase Merchant Services, monitors $1.1 trillion in online payment volume annually. The platform uses supervised ML to detect known fraud patterns and unsupervised ML to identify atypical behavior.
This dual approach has enabled Chase to detect credit card fraud and reducing by over 50% in the last five years. By proactively identifying new threats, the company stays ahead of fraudsters in the ever-evolving financial landscape.
Mastercard
Mastercard employs Decision Intelligence, an AI-based solution that analyzes transaction history and cardholder behavior to predict fraudulent activities. The system uses reinforcement learning to refine its decision-making capabilities over time.
This approach for credit card transaction fraud detection not only enhances detection accuracy but also minimizes false declines, ensuring that legitimate transactions proceed seamlessly.
Stripe
Stripe’s Radar is a machine learning-powered fraud prevention tool integrated into its payment platform. Designed with developers in mind, Radar uses billions of data points to detect anomalies in real-time. Businesses can customize their fraud rules to align with their specific risk tolerance.
Radar has become a go-to tool for startups and enterprises alike, offering flexibility and robust protection against credit card fraud detection machine learning.
Alibaba
Alibaba uses ML-based credit card fraud detection tools to safeguard its vast e-commerce ecosystem. The company’s AI-powered fraud detection system monitors billions of daily transactions, identifying suspicious activities such as fake accounts and payment anomalies.
By combining ML with big data analytics, Alibaba has significantly reduced fraud rates across its platforms, ensuring a secure shopping experience for millions of users.
Tech giants are leveling up fintech security with machine learning—are you in? Dive into our fintech development services now and make your mark!
As we’ve seen in real-world use cases, companies like PayPal, Visa, and Amazon are already harnessing the power of credit card fraud detection using machine learning. These examples demonstrate how ML models can be trained on vast datasets and continuously adapt to new threats, ensuring fraudulent transactions are detected with greater accuracy and speed.
However, integrating ML into your business model for credit card fraud prevention goes beyond just observing these successes – it involves a systematic approach to harnessing ML’s capabilities within your infrastructure.
Businesses can achieve similar outcomes by implementing the right strategies and choosing suitable algorithms. Whether you’re looking to replicate the successes of industry giants or tailor the system to your specific needs, integrating ML will allow you to catch fraud faster and stay ahead of evolving fraudulent techniques.
Let’s explore how to implement credit card fraud detection with ML within your business model, turning these use cases into actionable steps.
How to Integrate ML for Credit Card Fraud Prevention in Your Business Model
Implementing machine learning for credit card fraud detection strategy can significantly enhance your ability to detect and mitigate fraudulent activities. By leveraging data-driven insights and advanced algorithms, businesses can build more robust fraud detection systems that improve over time.
Here’s the process of credit card fraud detection with ML:
Data Collection and Integration
The first step in the integration process is gathering relevant transaction data. This includes structured data (transaction amounts, merchant details, cardholder information) and unstructured data (customer behaviors, device usage), which can be sourced from internal transaction databases or third-party vendors.
Integrating the datasets into a central data repository would ultimately allow for the comprehensive analysis required by ML models.
Choose the Right ML Algorithms
Select ML models that align with your business needs. Depending on the scale of your business and the complexity of your transactions, you might choose between these types of credit card fraud detection tools:
- Supervised Learning: Suitable for businesses with historical fraud data. Algorithms such as decision trees or logistic regression learn from past fraudulent patterns to predict future fraud risk.
- Unsupervised Learning: Ideal for businesses that may not have labeled fraud data but want to detect anomalies. Clustering algorithms and anomaly detection can identify unusual behavior without prior knowledge of fraud patterns.
- Deep Learning: For larger enterprises or those dealing with complex, high-volume transactions, deep learning models like neural networks can provide superior accuracy in detecting subtle fraud indicators.
Data Preprocessing and Feature Engineering
Before feeding data into ML models, it needs to be cleaned and prepared. Businesses should invest in feature engineering, including meaningful features such as transaction frequency, location patterns, or unusual spending spikes.
Ultimately, proper data preprocessing is to help the ML model identify the most relevant patterns and improve its accuracy in detecting fraud.
Real-Time Monitoring and Transaction Scoring
Implementing credit card fraud detection with ML involves setting up a real-time monitoring system where each transaction is evaluated against the trained model to determine the likelihood of fraud.
Businesses can prioritize high-risk transactions by assigning a fraud score for further investigation or immediate action. For instance, transactions exceeding a certain threshold could be automatically blocked or flagged for manual review.
Implement Continuous Learning
One of the key benefits of the credit card fraud detection system using machine learning is its ability to learn and adapt over time. For seamless integration into your business model, ensure your system continuously updates as new data flows in.
Adaptive learning makes the model more accurate by incorporating recent fraud trends and behaviors. This process helps stay ahead of evolving fraud tactics.
Actionable Insights and Decision-Making
Integrating ML means your fraud prevention system doesn’t just identify fraud; it also provides actionable insights. With the right setup, your system can automatically initiate a response based on the fraud score – temporarily blocking a transaction, notifying the cardholder, or sending an alert for a manual review.
Scalability and Flexibility
For businesses of all sizes, scalability is crucial. ML models are designed to handle vast amounts of data and transactions. Whether small businesses or global enterprises, ML systems can scale to meet your growing fraud detection needs; as your business expands, the system can easily accommodate more transactions without compromising performance.
Integration with Other Security Systems
Implementing machine learning for credit card fraud with other security measures such as two-factor authentication (2FA), encryption, and secure payment gateways creates a comprehensive fraud prevention infrastructure. This multi-layered approach ensures maximum protection against fraud while providing a seamless customer experience.
As we move from understanding the process of credit card fraud detection with ML to addressing the challenges that arise, it’s essential to recognize that no solution is without its hurdles.
In our experience as a fintech software development company with decade-long expertise, even with the most advanced algorithms and vast datasets, obstacles such as data quality, real-time processing demands, and the evolving nature of fraud can hinder success.
However, we’ve seen firsthand that these challenges are not insurmountable. By carefully considering each issue and applying tailored solutions, businesses can overcome them and build more robust and adaptive fraud detection systems.
We have done this with our wide range of eCommerce and fintech-only clients by helping them address challenges head-on to create more accurate predictions, faster response times, and, ultimately, more secure transactions. Let’s dive deeper into the specific hurdles we’ve encountered and how we’ve helped our clients navigate them effectively.
Credit Card Fraud Detection Challenges
As experts in machine learning consulting services, we’ve had the opportunity to work with various clients looking to integrate ML into their credit card fraud detection systems. Throughout these experiences, we’ve encountered several challenges that, while not insurmountable, require careful consideration and tailored solutions to ensure successful implementation.
Here’s a look at some of the most common credit card fraud detection challenges we’ve faced and the strategies we applied to overcome them:
Data Quality and Availability
One of the primary challenges we encountered early on was the inconsistency and lack of quality in transaction data. Credit card fraud detection machine learning models are only as good as the data they are trained on, and we often found that many of our clients didn’t have sufficiently clean, labeled data to train robust models.
In some cases, fraud data was sparse, with only a small percentage of transactions being fraudulent, making it harder for the model to learn and generalize effectively.
Solution:
To address this, we advised clients to improve their data collection practices, ensuring that all relevant data, such as transaction details, customer behavior, and contextual information, was captured consistently. When labeled fraud data was limited, we applied data augmentation and
anomaly detection to artificially generate fraud patterns, enabling the models to become more accurate with limited samples.
Imbalanced Datasets
In credit card fraud detection, fraud events are inherently rare, creating highly imbalanced datasets where fraudulent transactions account for only a small fraction of all transactions. This imbalance often leads to models biased toward predicting non-fraudulent transactions, thus missing many actual fraud cases (false negatives).
Solution:
To solve this, we implemented various techniques to handle class imbalance, such as resampling methods (over-sampling the minority fraud class or under-sampling the majority non-fraud class). We also employed credit card fraud detection system development powered by anomaly detection, better suited to identify rare fraudulent transactions by focusing on outliers.
Moreover, we utilized specialized evaluation metrics like Precision-Recall AUC instead of accuracy, as this gave a clearer picture of model performance on imbalanced datasets.
Real-Time Processing Requirements
Credit card transaction fraud detection models must operate in real-time, where even a few seconds delay could result in fraudulent transactions being approved. This introduces a challenge for the system regarding processing speed, especially when dealing with large datasets or complex deep-learning models. Our clients needed fraud detection solutions that could process millions of transactions quickly without compromising accuracy.
Solution:
To address this, we optimized model deployment by leveraging model pruning and quantization techniques to reduce the complexity and size of models without sacrificing accuracy. Additionally, we suggested using streaming data pipelines and edge computing to analyze transactions in real-time as they come in, ensuring minimal latency and faster response times. These changes significantly improved the system’s ability to detect fraud immediately while keeping the detection process scalable.
Evolving Fraud Patterns
Fraudsters constantly adapt their strategies, introducing new fraudulent behavior patterns not in historical data. This means that models can quickly become outdated and less effective unless they continuously learn from new data. The challenge we faced was ensuring that the models remained adaptive and could detect emerging fraud tactics in real-time.
Solution:
To tackle this, we recommended adopting online learning and continuous model retraining in the software you build for detecting credit card fraud. By setting up a feedback loop where the model’s predictions were constantly evaluated and used to retrain it, we ensured that our client’s systems remained updated with the latest fraud trends. Additionally, we encouraged using hybrid models that combined both supervised and unsupervised learning, enabling the system to autonomously learn from labeled data and uncover new, previously unseen fraud patterns.
Interpretability of ML Models
A common challenge, especially with deep learning models, is the lack of transparency in decision-making. While complex models like neural networks can achieve impressive accuracy, they often operate as “black boxes,” making it difficult for stakeholders to understand how a decision was made. This lack of interpretability can be problematic in credit card fraud detection machine learning when decisions need to be explained to cardholders or regulatory bodies.
Solution:
To address this, we applied techniques like LIME (Local Interpretable Model-Agnostic Explanations) and SHAP (Shapley Additive Explanations), which helped provide insights into which features most influenced the model’s predictions.
We also used ensemble models that combined multiple simple models, making the decision process more transparent while maintaining high accuracy. By improving the interpretability of the models, we ensured that our clients could explain the reasoning behind fraud decisions better, enhancing both trust and compliance with regulations.
Resource and Computational Costs
ML models, especially deep learning ones, can be resource-intensive and require significant computational power to train and deploy. For many businesses, particularly smaller ones or those with limited budgets, the cost of implementing credit card fraud detection with ML and maintaining these systems can be a barrier.
Solution:
We recommended cloud-based solutions to address these resource challenges. By utilizing cloud platforms, businesses could scale their fraud detection models based on demand, avoiding the need for expensive on-premise hardware. We also suggested leveraging pre-trained models and transfer learning to reduce training time and computational costs, enabling businesses to integrate sophisticated models without requiring extensive in-house resources.
Advantages of Machine Learning in Credit Card Fraud Prevention
Financial institutions use machine learning to enhance their defenses as credit card fraud becomes more sophisticated. Unlike traditional systems, ML-based fraud detection offers dynamic, data-driven solutions that identify suspicious activity and adapt to new fraud tactics. Here are the key benefits of using machine learning for credit card fraud detection:
Real-Time Fraud Detection
Machine learning models process vast amounts of transactional data in real-time, enabling the detection of fraudulent activities as they occur. This instantaneous response minimizes potential financial losses and prevents fraudsters from exploiting a card further. Whether flagging an unusually large purchase or recognizing an out-of-pattern transaction, ML provides the speed and precision required for immediate action.
Enhanced Accuracy and Precision
ML algorithms analyze multiple variables simultaneously, uncovering complex patterns and correlations that are impossible to detect using manual methods. This capability significantly reduces false positives – legitimate transactions flagged as fraud – and false negatives – fraudulent transactions that go undetected. Enhanced accuracy ensures smoother customer experiences while effectively mitigating fraud risks.
Adaptability to Evolving Fraud Tactics
Fraudsters continuously develop new techniques to bypass traditional defenses. To counter this, one of the key advantages of machine learning in credit card fraud prevention lies in adapting to these evolving tactics. By learning from historical data and identifying emerging trends, ML models can detect anomalies that traditional systems would miss. This adaptability ensures that detection capabilities remain robust even as fraud strategies become more advanced.
Scalability for High-Volume Transactions
Transaction volumes are skyrocketing in today’s digital economy, especially in e-commerce and online banking. Credit card fraud detection using machine learning can effortlessly scale to analyze millions of transactions simultaneously, making them ideal for high-transaction environments. This scalability ensures consistent performance regardless of the data load, providing comprehensive protection across all customer interactions.
Proactive Fraud Prevention
Machine learning adopts a proactive approach unlike traditional systems that rely on predefined rules. ML models can anticipate potential fraud patterns by continuously analyzing and learning from data before they fully materialize. This predictive capability brought in through a credit card fraud detection system using machine learning helps financial institutions address vulnerabilities in advance, reducing the likelihood of successful fraud attempts.
Improved Operational Efficiency
Machine learning automates the fraud detection process, reducing the manual workload for fraud analysts. This allows institutions to allocate resources more effectively, focusing on high-priority cases or refining fraud prevention strategies. Additionally, the automation introduced by fraud detection machine learning reduces human error, enhancing the system’s overall reliability.
Cost Savings
By detecting and preventing fraud more efficiently, machine learning helps minimize the financial impact of fraudulent transactions. The reduced incidence of false positives also lowers operational costs, as fewer legitimate transactions need manual review. Over time, these cost savings contribute to a more sustainable and secure financial ecosystem.
Enhanced Customer Trust and Retention
Providing customers with a secure and seamless transaction experience builds trust and loyalty. One of the other prominent benefits of using machine learning for credit card fraud detection lies in the technology enabling financial institutions to protect their customers’ financial data while ensuring legitimate transactions proceed without interruptions. This balance between security and convenience is critical for retaining customer confidence in an increasingly competitive market.
While the advantages of machine learning in credit card fraud prevention highlight its transformative potential, understanding its real-world application provides deeper insight into its impact.
Our custom machine-learning services tackle fraud head-on, letting you see the magic in your digital product. Hit us up for a quote and a chat with our ML pros today.
Appinventiv: Leverage ML Capabilities to Protect Millions of Daily Transactions
Professional companies are revolutionizing fraud prevention and setting new benchmarks in risk mitigation across the financial ecosystem through the most advanced credit card fraud detection software development.
Machine learning has undeniably transformed the landscape of credit card fraud detection, offering businesses the tools to stay one step ahead of increasingly sophisticated fraudsters. By integrating advanced algorithms and continuously evolving models, organizations can improve the accuracy and speed of fraud detection and ensure a more secure, seamless experience for their customers.
Appinventiv is a leading fintech software development company renowned for developing next-gen fintech development services tailored to your business niche. Advanced technologies like AI and machine learning back our fintech development finesse. Thus, we provide expert machine learning consulting services to help businesses harness the power of ML in securing and making financial ops fraud-proof.
If you’re looking to integrate machine learning into your credit card fraud detection systems or need help navigating the complexities of its implementation, our team is here to assist. Contact us today to develop a robust fraud detection framework to secure transactions, driving your businesses further ahead.
FAQs
Q. What is credit card fraud detection?
A. Credit card fraud detection refers to identifying and preventing unauthorized or fraudulent transactions using a credit card. The goal is to recognize suspicious activity as early as possible to minimize financial losses and protect consumers and businesses. Traditional fraud detection methods rely on predefined rules, but with the rise of sophisticated fraud techniques, machine learning has become an increasingly effective tool. Using machine learning, these models built on credit card fraud detection analyze vast amounts of data in real-time, flagging potentially fraudulent transactions based on patterns and behaviors that deviate from normal usage.
Q. How is machine learning used in credit card fraud detection?
A. Machine learning is used in credit card fraud detection to analyze historical transaction data and identify patterns and anomalies. ML algorithms can learn from legitimate and fraudulent transactions, continually improving their ability to detect new types of fraud. Common machine learning techniques, such as supervised, unsupervised, and deep learning, allow systems to adapt over time, detect emerging fraud tactics, and provide real-time alerts. The more data these models are exposed to, the better they become at predicting and preventing fraud, minimizing false positives, and ensuring smoother transaction processing.
Q. What Are the Most Effective Ways to Prevent Credit Card Fraud?
A. The most effective types of credit card fraud detection include:
- Machine Learning-Based Detection: Leveraging advanced ML algorithms to monitor transactions and detect anomalies in real-time, improving fraud detection accuracy and reducing false positives.
- Tokenization: Replacing sensitive credit card information with a unique identifier (token) during transactions, making it useless to hackers if stolen.
- Multi-Factor Authentication (MFA): Requiring multiple forms of verification (e.g., SMS codes, biometrics) to ensure the legitimacy of a transaction.
- Secure Payment Gateways: Using encrypted communication channels for transactions to prevent data breaches.
- Regular Monitoring and Auditing: Continuously reviewing transaction data for irregularities and patterns that may suggest fraudulent activity.
Q. How does credit card fraud detection work?
A. Credit card fraud detection works by continuously monitoring transactions for unusual behavior or patterns that suggest fraudulent activity. Initially, systems rely on predefined rules based on past fraud cases, such as flagging transactions from unusual locations or large purchases in a short time. However, with the machine learning-powered credit card fraud detection system development, the process has become much more sophisticated. ML models analyze historical transaction data, customer profiles, and other relevant factors to develop models that can identify subtle signs of fraud. When a suspicious transaction is detected, it’s flagged for review or immediate action, such as blocking the payment or requiring additional verification. These systems learn over time, adapting to new fraud tactics and reducing false positives for a more efficient fraud detection process.
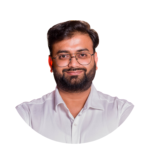
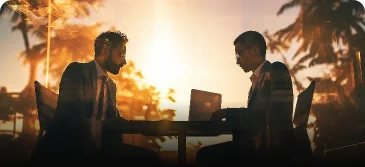
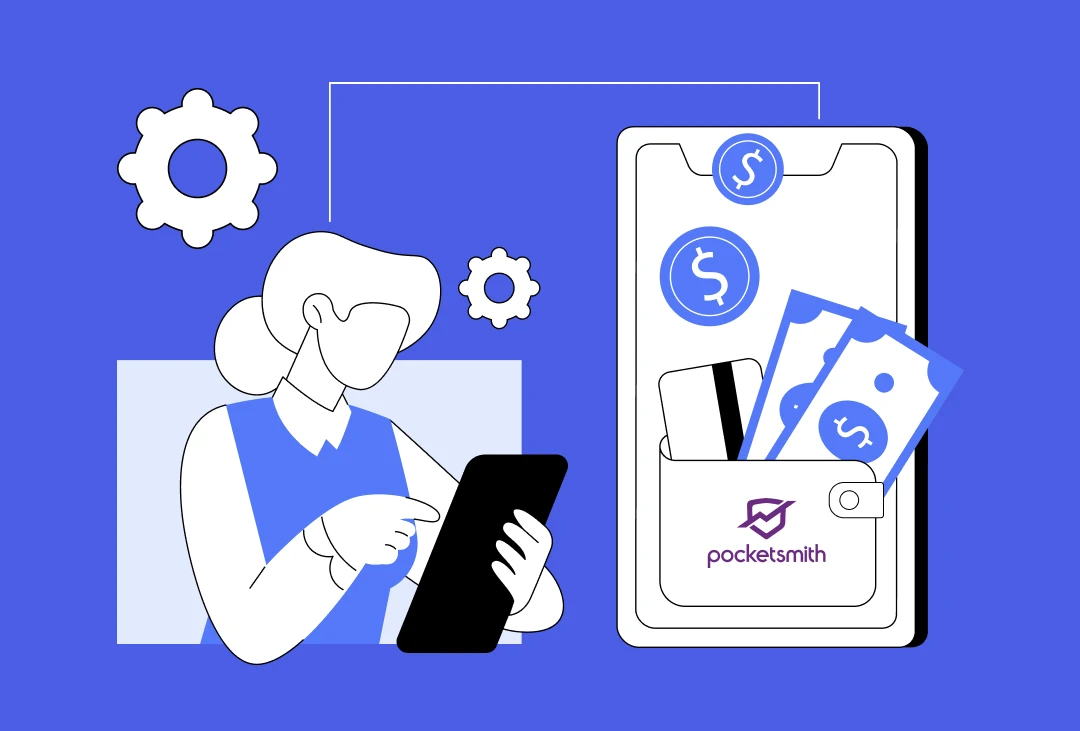
How Much Does it Cost to Build a Personal Finance App like Pocketsmith?
Imagine an app that knows when your rent is due, reminds you of upcoming bills, forecasts your savings 12 months ahead, and tells you if that third coffee this week is wrecking your budget. That’s the kind of experience apps like PocketSmith deliver, and it’s exactly why personal finance apps are becoming a must-have for…
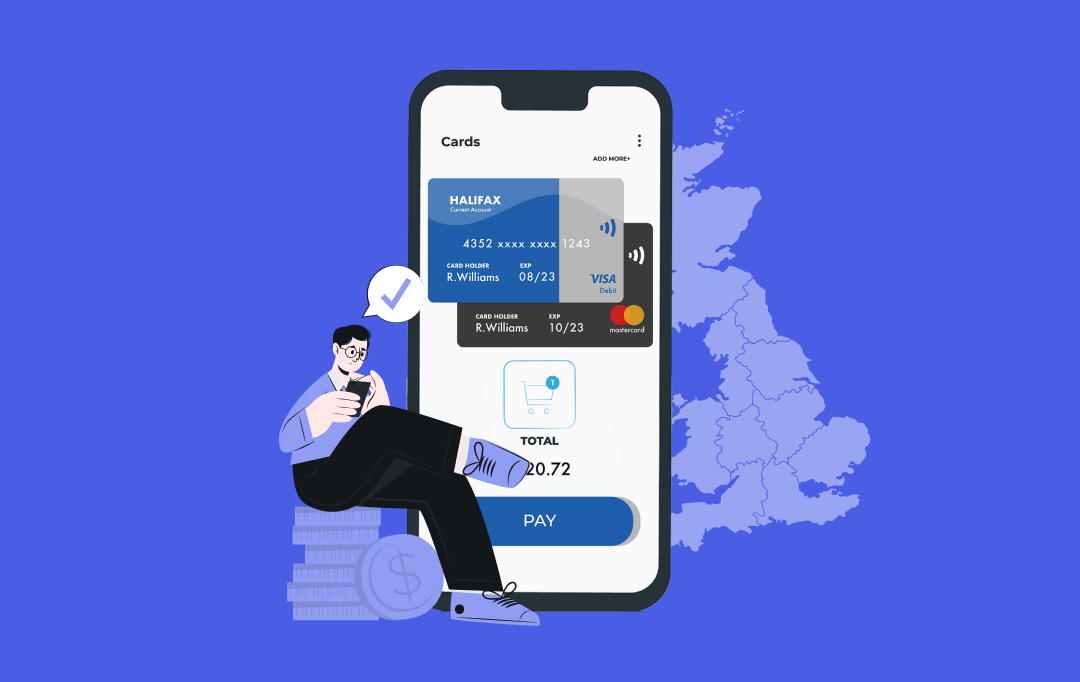
How Much Does it Cost to Build a Mobile Banking App Like Halifax in the UK?
The way people bank has changed dramatically. Gone are the days of waiting in long queues or rushing to a branch before closing hours. Today, mobile banking apps like Halifax have redefined convenience, allowing users to check balances, transfer funds, pay bills, and even apply for loans from their smartphones, anytime and anywhere. This shift…
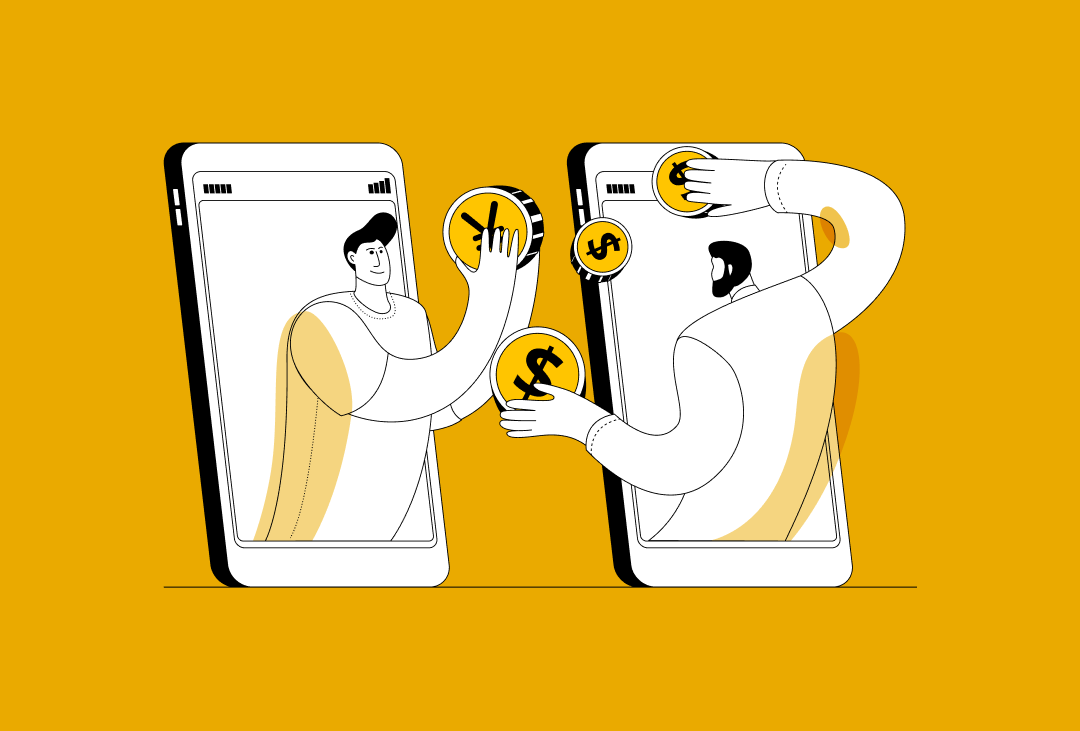
How to Approach Currency Converter App Development?
Imagine you’re about to travel abroad and need to quickly check how much your local currency is worth in another country, or perhaps you run an international business and constantly deal with multiple currencies. In both cases, a currency converter app is an indispensable tool. With global transactions becoming the norm and forex markets fluctuating…